On Construction and Estimation of Stationary Mixture Transition Distribution Models
JOURNAL OF COMPUTATIONAL AND GRAPHICAL STATISTICS(2022)
摘要
Mixture transition distribution (MTD) time series models build high-order dependence through a weighted combination of first-order transition densities for each one of a specified number of lags. We present a framework to construct stationary MTD models that extend beyond linear, Gaussian dynamics. We study conditions for first-order strict stationarity which allow for different constructions with either continuous or discrete families for the first-order transition densities given a prespecified family for the marginal density, and with general forms for the resulting conditional expectations. Inference and prediction are developed under the Bayesian framework with particular emphasis on flexible, structured priors for the mixture weights. Model properties are investigated both analytically and through synthetic data examples. Finally, Poisson and Lomax examples are illustrated through real data applications. Supplementary files for this article are available online.
更多查看译文
关键词
Bayesian inference, First-order strict stationarity, Markov chain Monte Carlo, Non-Gaussian time series
AI 理解论文
溯源树
样例
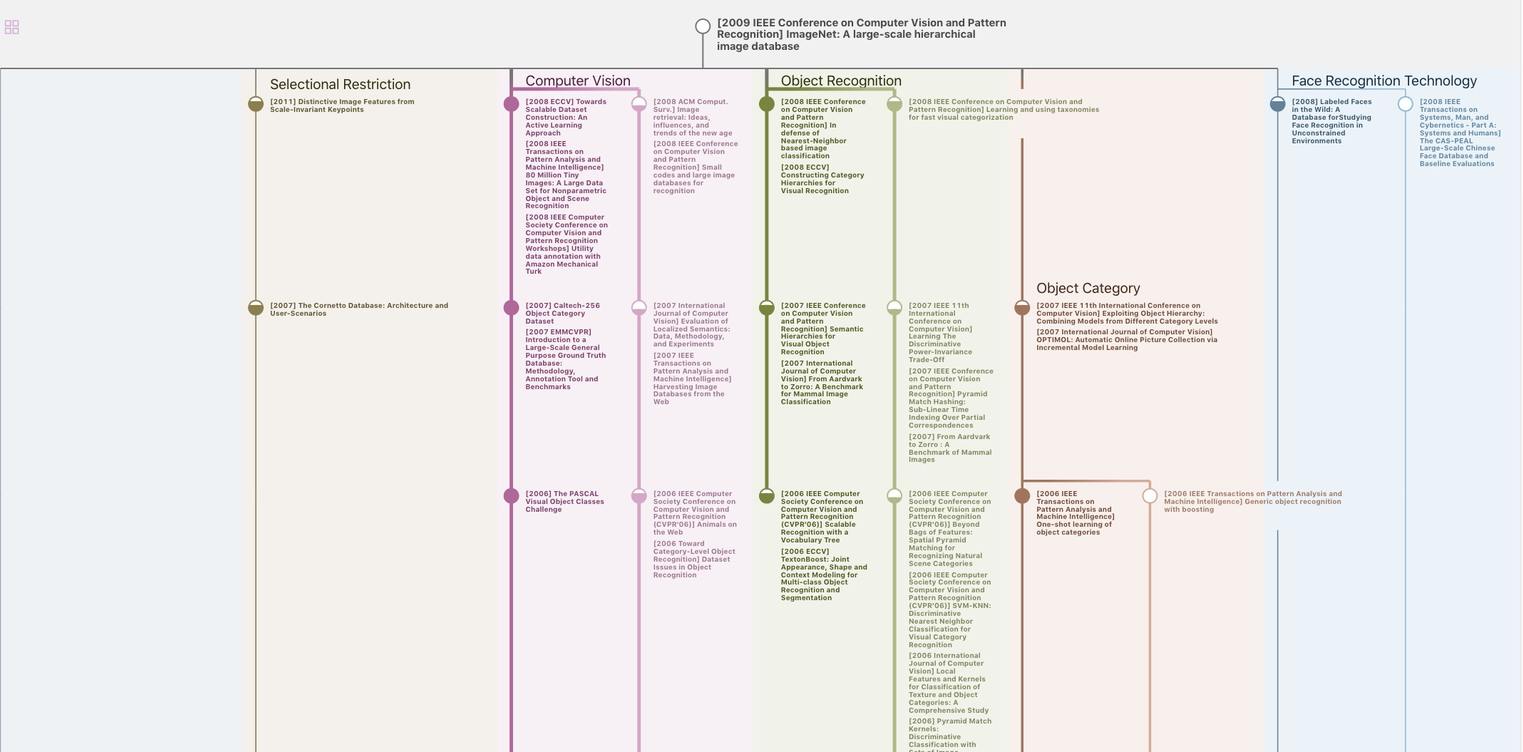
生成溯源树,研究论文发展脉络
Chat Paper
正在生成论文摘要