Instance-Wise Minimax-Optimal Algorithms For Logistic Bandits
24TH INTERNATIONAL CONFERENCE ON ARTIFICIAL INTELLIGENCE AND STATISTICS (AISTATS)(2021)
摘要
Logistic Bandits have recently attracted substantial attention, by providing an uncluttered yet challenging framework for understanding the impact of non-linearity in parametrized bandits. It was shown by Faury et al. (2020) that the learning-theoretic difficulties of Logistic Bandits can be embodied by a large (sometimes prohibitively) problem-dependent constant kappa, characterizing the magnitude of the reward's non-linearity. In this paper we introduce a novel algorithm for which we provide a refined analysis. This allows for a better characterization of the effect of non-linearity and yields improved problem-dependent guarantees. In most favorable cases this leads to a regret upper-bound scaling as (O) over tilde (d root T/kappa), which dramatically improves over the (O) over tilde (d root T + kappa) state-of-the-art guarantees. We prove that this rate is minimax-optimal by deriving a Omega(d root T/kappa) problem-dependent lower-bound. Our analysis identifies two regimes (permanent and transitory) of the regret, which ultimately re-conciliates (Faury et al., 2020) with the Bayesian approach of Dong et al. (2019). In contrast to previous works, we find that in the permanent regime non-linearity can dramatically ease the exploration-exploitation trade-off. While it also impacts the length of the transitory phase in a problem-dependent fashion, we show that this impact is mild in most reasonable configurations.
更多查看译文
AI 理解论文
溯源树
样例
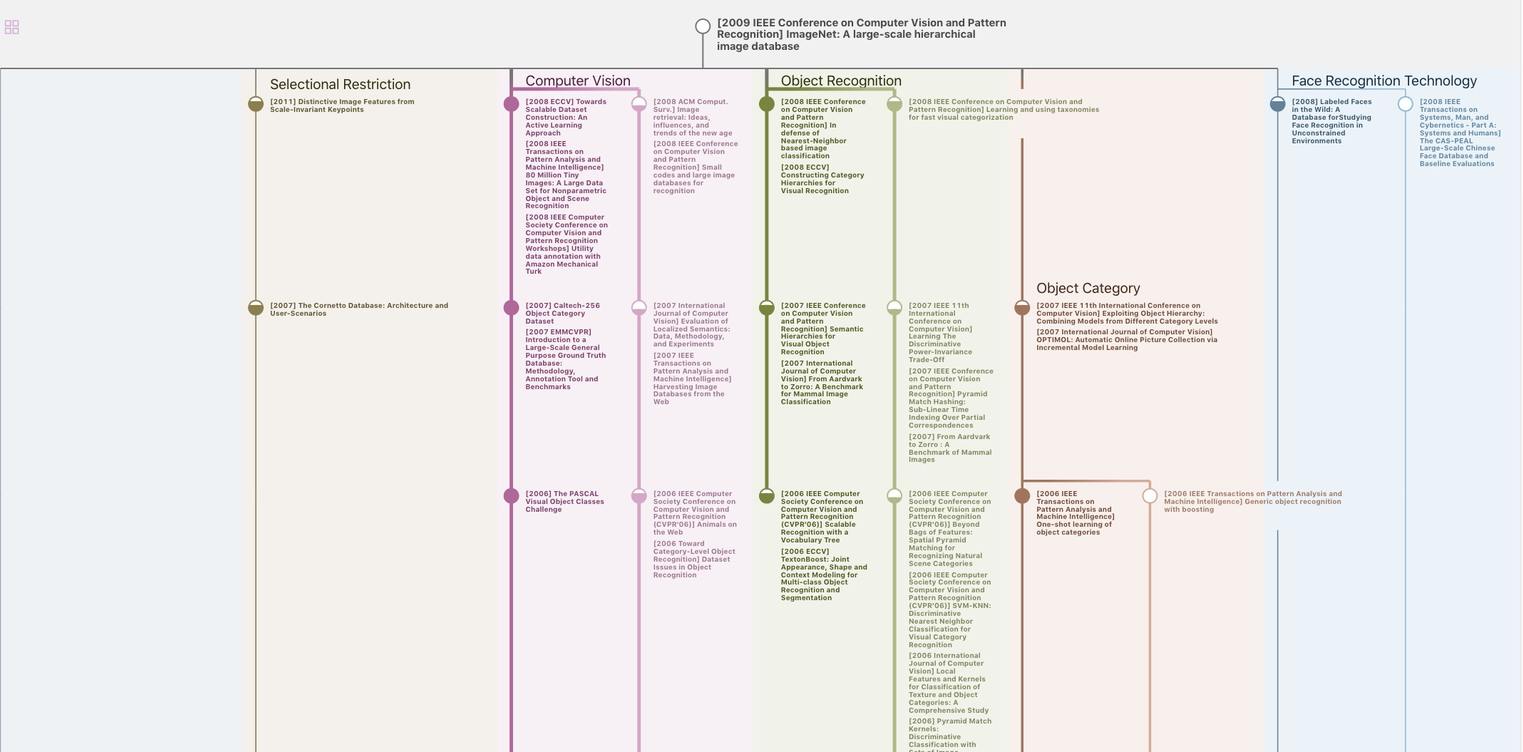
生成溯源树,研究论文发展脉络
Chat Paper
正在生成论文摘要