Topic Modeling with Contextualized Word Representation Clusters
arxiv(2020)
摘要
Clustering token-level contextualized word representations produces output that shares many similarities with topic models for English text collections. Unlike clusterings of vocabulary-level word embeddings, the resulting models more naturally capture polysemy and can be used as a way of organizing documents. We evaluate token clusterings trained from several different output layers of popular contextualized language models. We find that BERT and GPT-2 produce high quality clusterings, but RoBERTa does not. These cluster models are simple, reliable, and can perform as well as, if not better than, LDA topic models, maintaining high topic quality even when the number of topics is large relative to the size of the local collection.
更多查看译文
关键词
contextualized word representation clusters,topic modeling
AI 理解论文
溯源树
样例
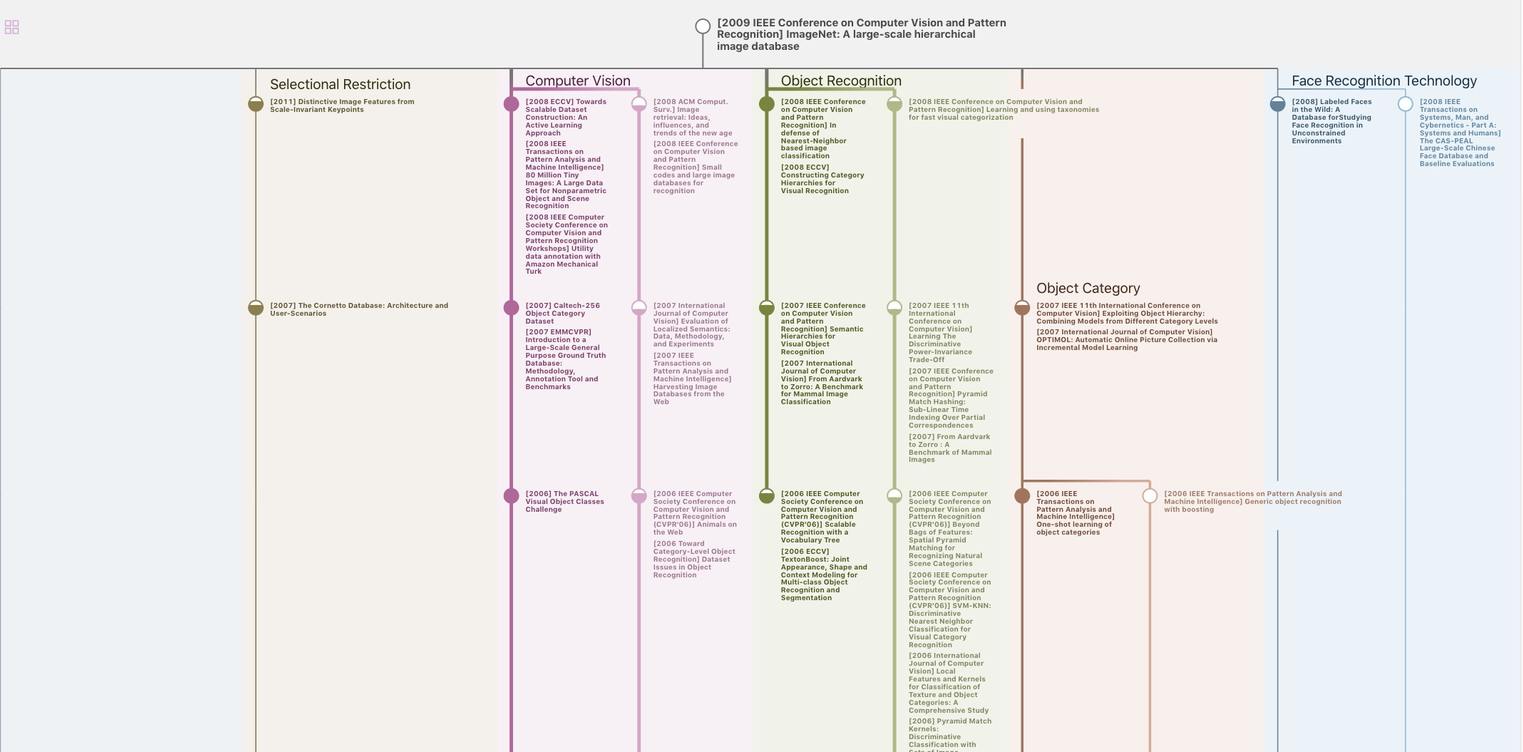
生成溯源树,研究论文发展脉络
Chat Paper
正在生成论文摘要