Few-shot Learning for Decoding Brain Signals
arxiv(2020)
摘要
Few-shot learning consists in addressing data-thrifty (inductive few-shot) or label-thrifty (transductive few-shot) problems. So far, the field has been mostly driven by applications in computer vision. In this work, we are interested in stressing the ability of recently introduced few-shot methods to solve problems dealing with neuroimaging data, a promising application field. To this end, we propose a benchmark dataset and compare multiple learning paradigms, including meta-learning, as well as various backbone networks. Our experiments show that few-shot methods are able to efficiently decode brain signals using few examples, and that graph-based backbones do not outperform simple structure-agnostic solutions, such as multi-layer perceptrons.
更多查看译文
关键词
decoding,brain signals,learning,few-shot
AI 理解论文
溯源树
样例
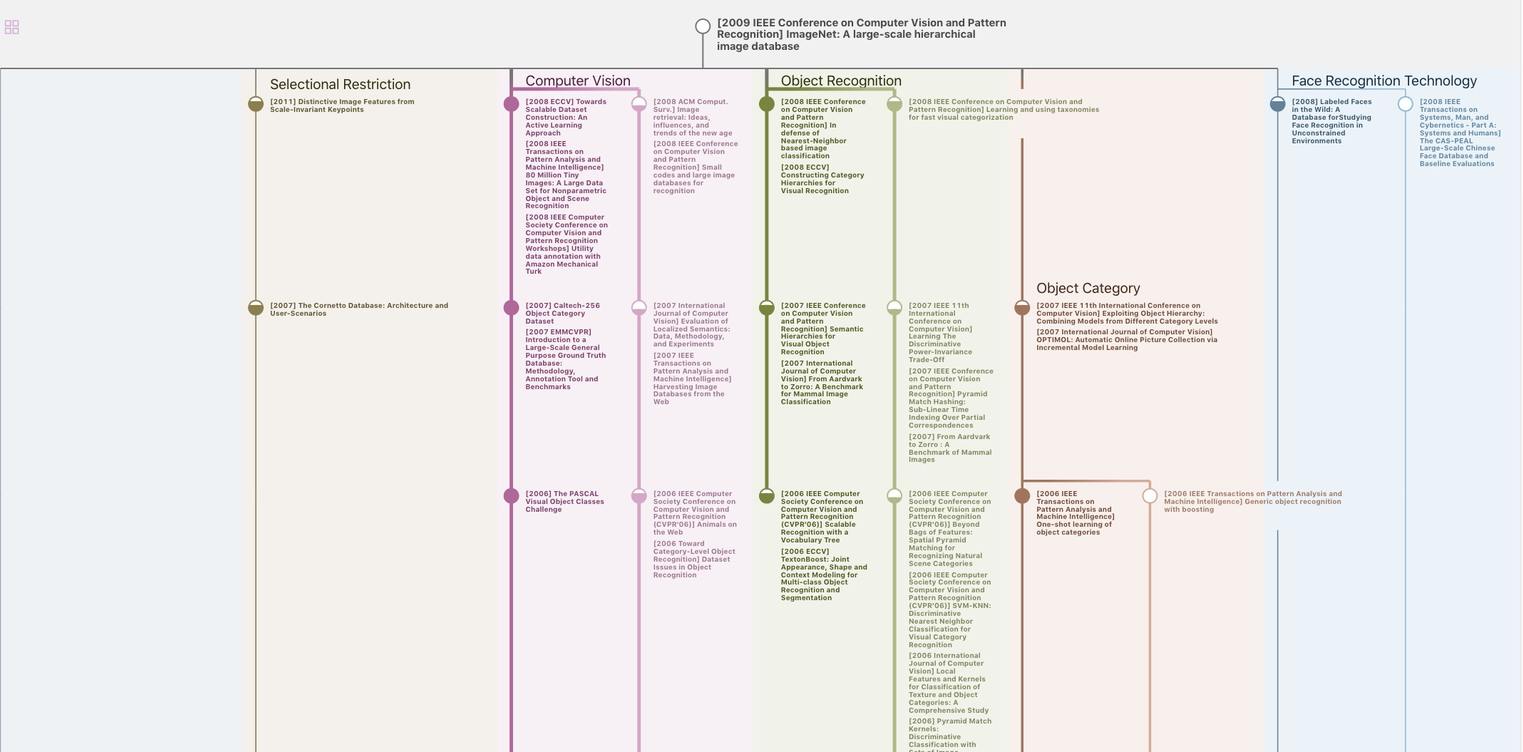
生成溯源树,研究论文发展脉络
Chat Paper
正在生成论文摘要