On the robustness of noise-blind low-rank recovery from rank-one measurements
Linear Algebra and its Applications(2022)
摘要
We prove new results about the robustness of well-known convex noise-blind optimization formulations for the reconstruction of low-rank matrices from an underdetermined system of random linear measurements. Specifically, our results address random Hermitian rank-one measurements as used in a version of the phase retrieval problem; that is, each measurement can be represented as the inner product of the unknown matrix and the outer product of a given realization of the standard complex Gaussian random vector.
更多查看译文
关键词
15A83,90C17,90C22
AI 理解论文
溯源树
样例
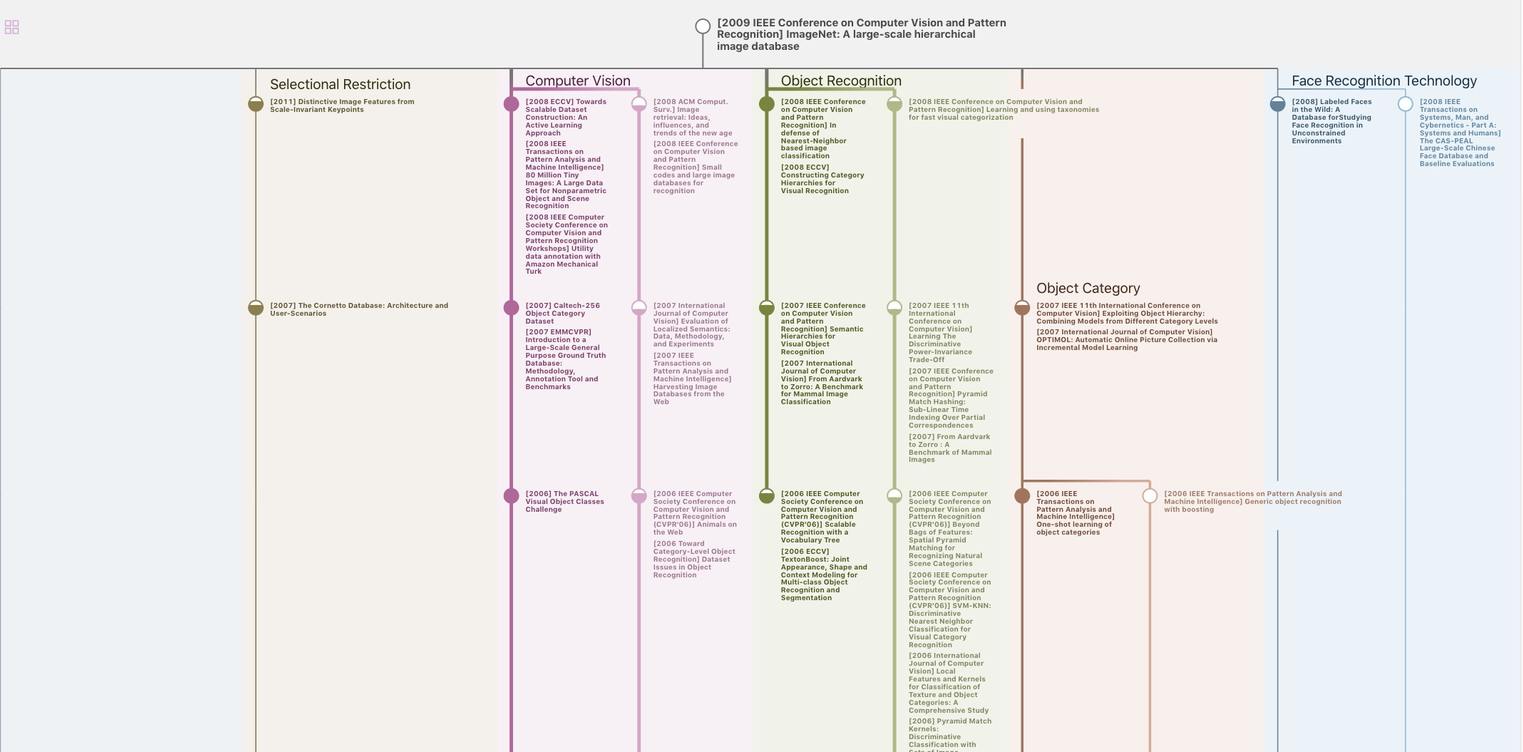
生成溯源树,研究论文发展脉络
Chat Paper
正在生成论文摘要