AdaCrowd: Unlabeled Scene Adaptation for Crowd Counting
IEEE Transactions on Multimedia(2022)
摘要
We address the problem of image-based crowd counting. In particular, we propose a new problem called
unlabeled scene-adaptive crowd counting
. Given a new target scene, we would like to have a crowd counting model specifically adapted to this particular scene based on the target data that capture some information about the new scene. In this paper, we propose to use one or more unlabeled images from the target scene to perform the adaptation. In comparison with the existing problem setups (e.g. fully supervised), our proposed problem setup is closer to the real-world applications of crowd counting systems. We introduce a novel
AdaCrowd
framework to solve this problem. Our framework consists of a crowd counting network and a guiding network. The guiding network predicts some parameters in the crowd counting network based on the unlabeled images from a particular scene. This allows our model to adapt to different target scenes. The experimental results on several challenging benchmark datasets demonstrate the effectiveness of our proposed approach compared with other alternative methods. Code is available at
https://github.com/maheshkkumar/adacrowd
更多查看译文
关键词
Computer vision,crowd counting,deep learning,scene adaptation
AI 理解论文
溯源树
样例
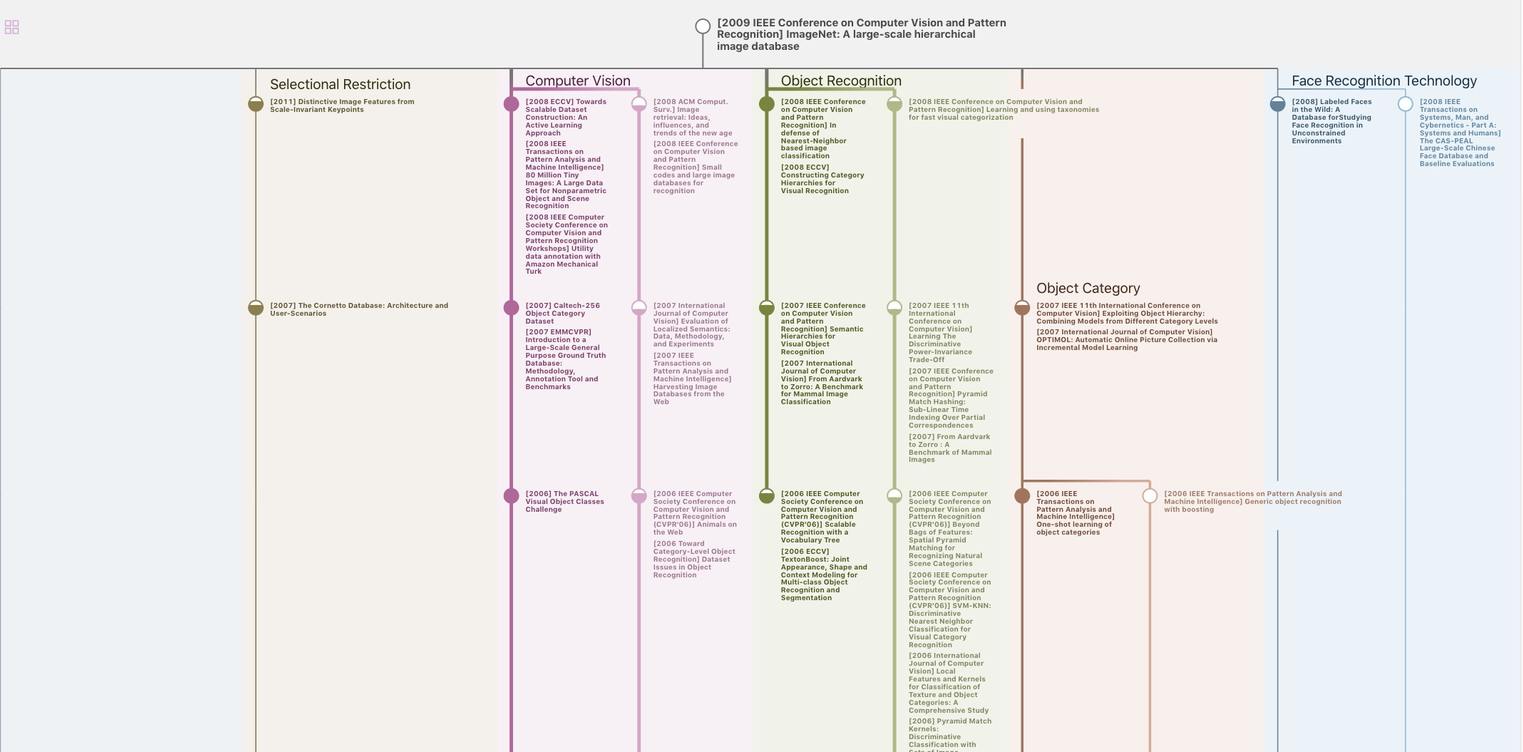
生成溯源树,研究论文发展脉络
Chat Paper
正在生成论文摘要