Sharper convergence bounds of Monte Carlo Rademacher Averages through Self-Bounding functions
arxiv(2020)
摘要
We derive sharper probabilistic concentration bounds for the Monte Carlo Empirical Rademacher Averages (MCERA), which are proved through recent results on the concentration of self-bounding functions. Our novel bounds allow obtaining sharper bounds to (Local) Rademacher Averages. We also derive novel variance-aware bounds for the special case where only one vector of Rademacher random variables is used to compute the MCERA. Then, we leverage the framework of self-bounding functions to derive novel probabilistic bounds to the supremum deviations, that may be of independent interest.
更多查看译文
关键词
monte carlo rademacher averages,sharper convergence bounds,self-bounding
AI 理解论文
溯源树
样例
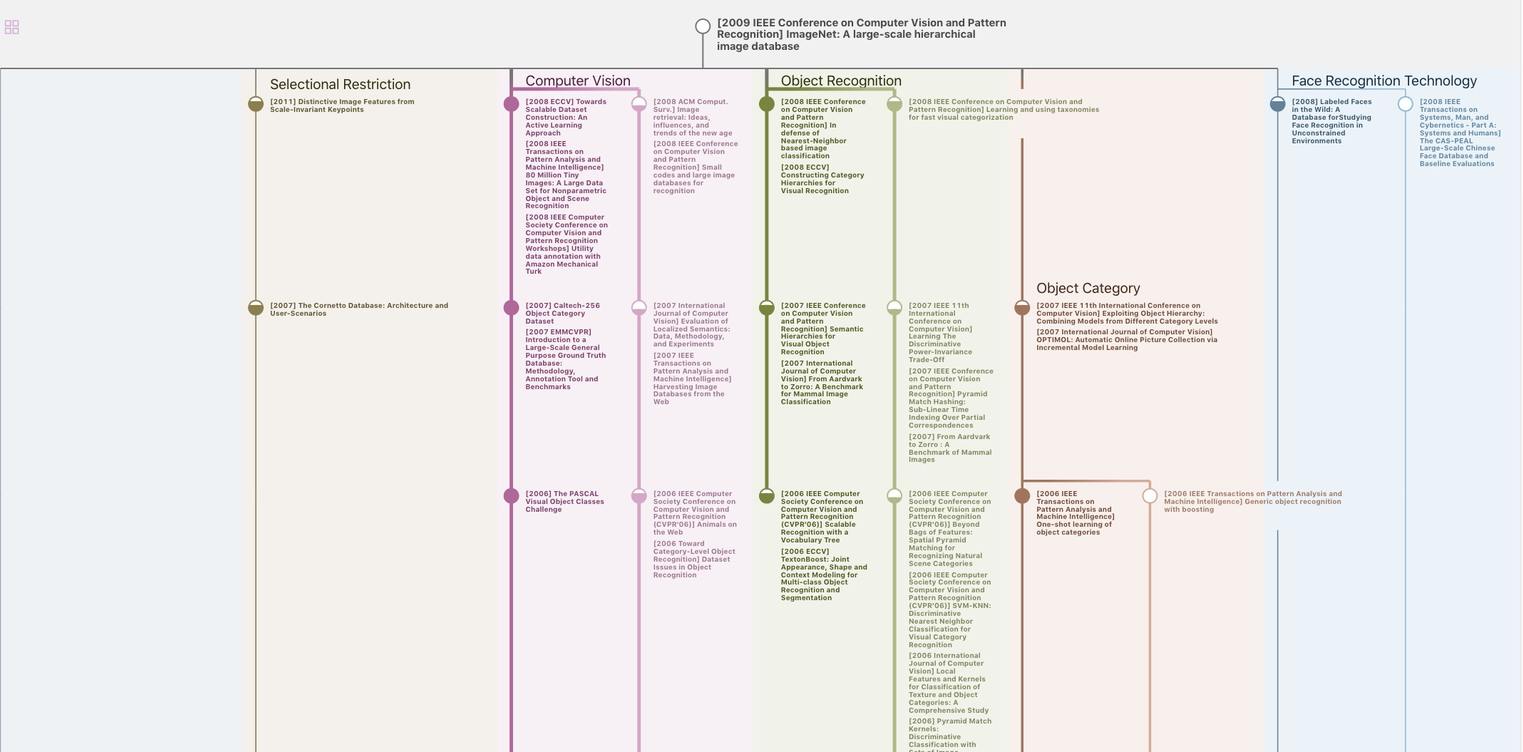
生成溯源树,研究论文发展脉络
Chat Paper
正在生成论文摘要