Characterizing Datasets for Social Visual Question Answering, and the New TinySocial Dataset
2020 Joint IEEE 10th International Conference on Development and Learning and Epigenetic Robotics (ICDL-EpiRob)(2020)
摘要
Modern social intelligence includes the ability to watch videos and answer questions about social and theory-of-mind-related content, e.g., for a scene in Harry Potter, “Is the father really upset about the boys flying the car?” Social visual question answering (social VQA) is emerging as a valuable methodology for studying social reasoning in both humans (e.g., children with autism) and AI agents. However, this problem space spans enormous variations in both videos and questions. We discuss methods for creating and characterizing social VQA datasets, including 1) crowdsourcing versus in-house authoring, including sample comparisons of two new datasets that we created (TinySocial-Crowd and TinySocial-InHouse) and the previously existing Social-IQ dataset; 2) a new rubric for characterizing the difficulty and content of a given video; and 3) a new rubric for characterizing question types. We close by describing how having well-characterized social VQA datasets will enhance the explainability of AI agents and can also inform assessments and educational interventions for people.
更多查看译文
关键词
social reasoning,social VQA datasets,Social-IQ dataset,question types,TinySocial dataset,modern social intelligence,social visual question answering,TinySocial-InHouse,TinySocial-Crowd,AI agents
AI 理解论文
溯源树
样例
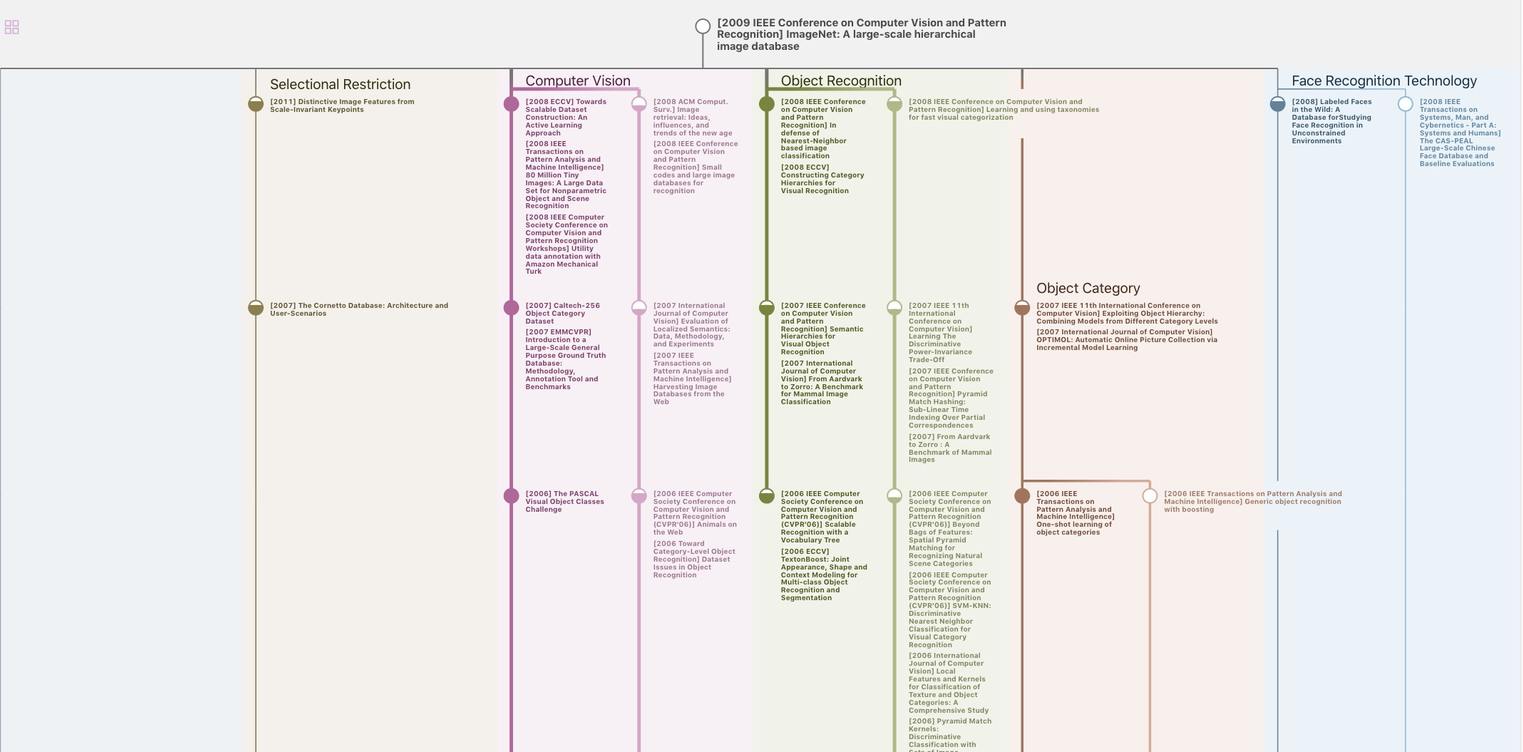
生成溯源树,研究论文发展脉络
Chat Paper
正在生成论文摘要