Unsupervised deep learning for grading of age-related macular degeneration using retinal fundus images
arxiv(2020)
摘要
Many diseases are classified based on human-defined rubrics that are prone to bias. Supervised neural networks can automate the grading of retinal fundus images, but require labor-intensive annotations and are restricted to the specific trained task. Here, we employed an unsupervised network with Non-Parametric Instance Discrimination (NPID) to grade age-related macular degeneration (AMD) severity using fundus photographs from the Age-Related Eye Disease Study (AREDS). Our unsupervised algorithm demonstrated versatility across different AMD classification schemes without retraining, and achieved unbalanced accuracies comparable to supervised networks and human ophthalmologists in classifying advanced or referable AMD, or on the 4-step AMD severity scale. Exploring the networks behavior revealed disease-related fundus features that drove predictions and unveiled the susceptibility of more granular human-defined AMD severity schemes to misclassification by both ophthalmologists and neural networks. Importantly, unsupervised learning enabled unbiased, data-driven discovery of AMD features such as geographic atrophy, as well as other ocular phenotypes of the choroid, vitreous, and lens, such as visually-impairing cataracts, that were not pre-defined by human labels.
更多查看译文
关键词
macular degeneration,unsupervised deep learning,deep learning,age-related
AI 理解论文
溯源树
样例
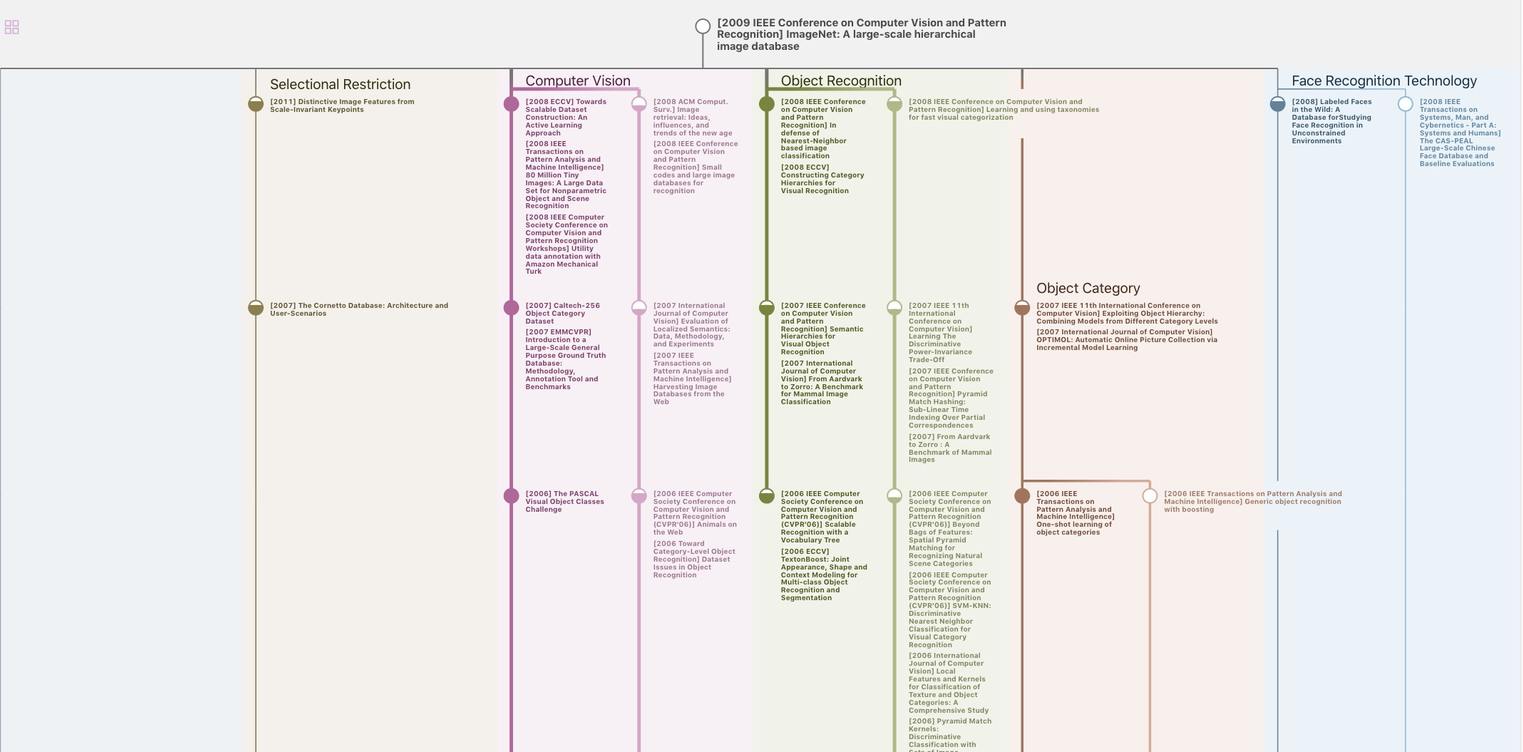
生成溯源树,研究论文发展脉络
Chat Paper
正在生成论文摘要