Modular mechanisms for Bayesian optimization
NIPS workshop on Bayesian optimization(2014)
摘要
The design of methods for Bayesian optimization involves a great number of choices that are often implicit in the overall algorithm design. In this work we argue for a modular approach to Bayesian optimization and present a Python implementation, pybo, that allows us to easily vary these choices. In particular this includes selection of the acquisition function, kernel, and hyperpriors as well as less-discussed components such as the recommendation and initialization strategies. Ultimately this approach provides us a straightforward mechanism to examine the effect of each choice both individually and in combination.
更多查看译文
AI 理解论文
溯源树
样例
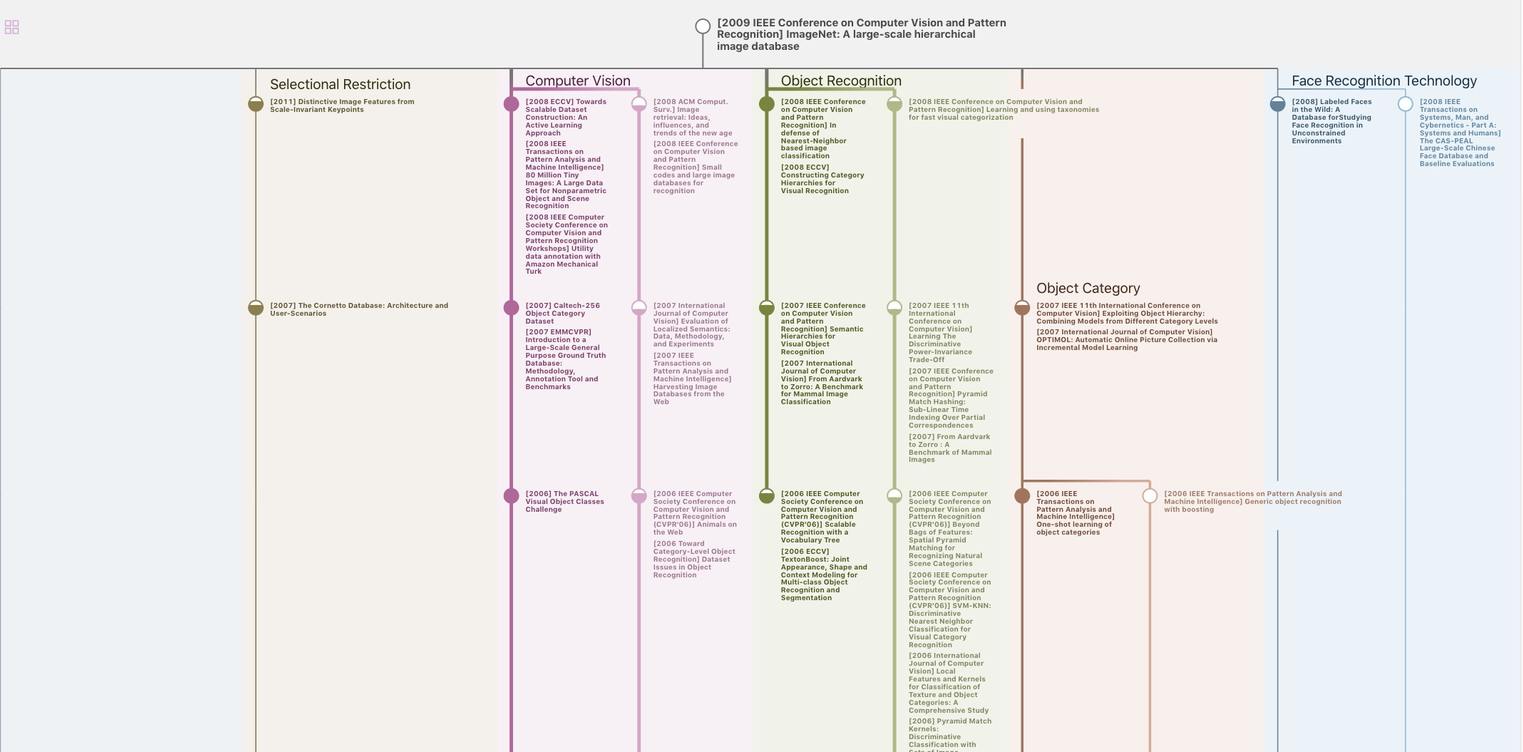
生成溯源树,研究论文发展脉络
Chat Paper
正在生成论文摘要