Learning Time Aware Assistance Functions for Numerical Fluid Solvers
user-5f8cfb314c775ec6fa691ca8(2019)
摘要
Improving the accuracy of numerical methods remains a central challenge in many disciplines and is especially important for nonlinear simulation problems. A representative example of such problems is fluid flow, which has been thoroughly studied to arrive at efficient simulations of complex flow phenomena. This paper presents a data-driven approach that learns to improve the accuracy of numerical solvers. The proposed method utilizes an advanced numerical scheme with a fine simulation resolution to acquire reference data. We, then, employ a neural network that infers a correction to move a coarse thus quickly obtainable result closer to the reference data. We provide insights into the targeted learning problem with different learning approaches: fully supervised learning methods with a naive and an optimized data acquisition as well as an unsupervised learning method with a differentiable Navier-Stokes solver. While our approach is very general and applicable to arbitrary partial differential equation models, we specifically highlight gains in accuracy for fluid flow simulations.
更多查看译文
AI 理解论文
溯源树
样例
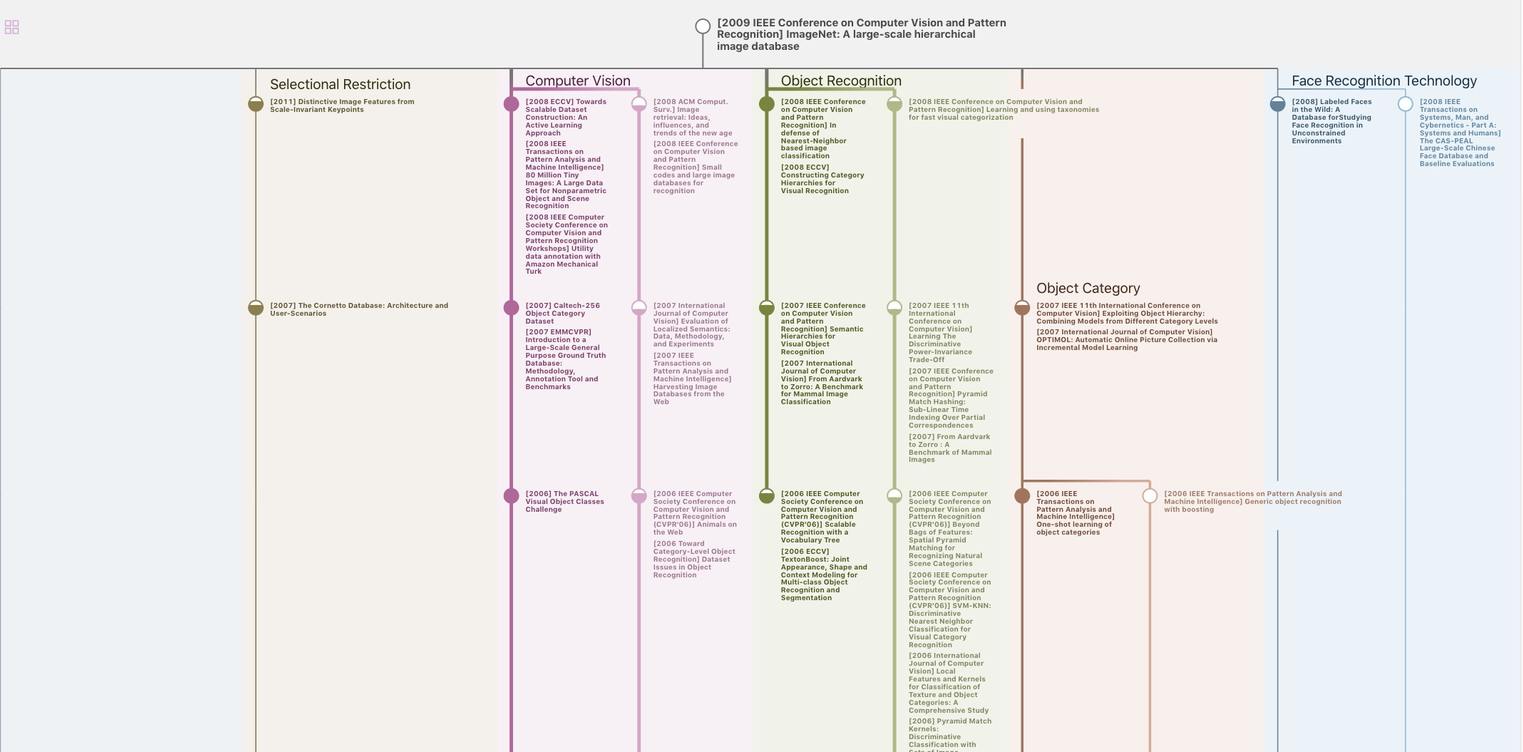
生成溯源树,研究论文发展脉络
Chat Paper
正在生成论文摘要