Discriminative Subtyping of Lung Cancers from Histopathology Images via Contextual Deep Learning
medRxiv(2020)
摘要
When designing individualized treatment protocols for cancer patients, clinicians must synthesize the information from multiple data modalities into a single parsimonious description of the patient9s personal disease. However, such a description of a patient is never observed. In this work, we propose to model these patient descriptions as latent \emph{discriminative subtypes}---sample representations which can be learned from one data modality and used to contextualize predictions based on another data modality. We apply contextual deep learning to learn these sample-specific discriminative subtypes from lung cancer histopathology imagery. Based on these subtypes, we produce sample-specific transcriptomic models which accurately classify samples as adenocarcinoma, squamous cell carcinoma, or healthy tissue (F1 score of 0.97, outperforming previous state-of-the-art multimodal approaches). Combining these data modalities in a single pipeline not only improves the predictive accuracy, but also gives biological interpretations of the discriminative subtypes and ties the phenotypic patterns present in histopathology images to biological processes.
更多查看译文
AI 理解论文
溯源树
样例
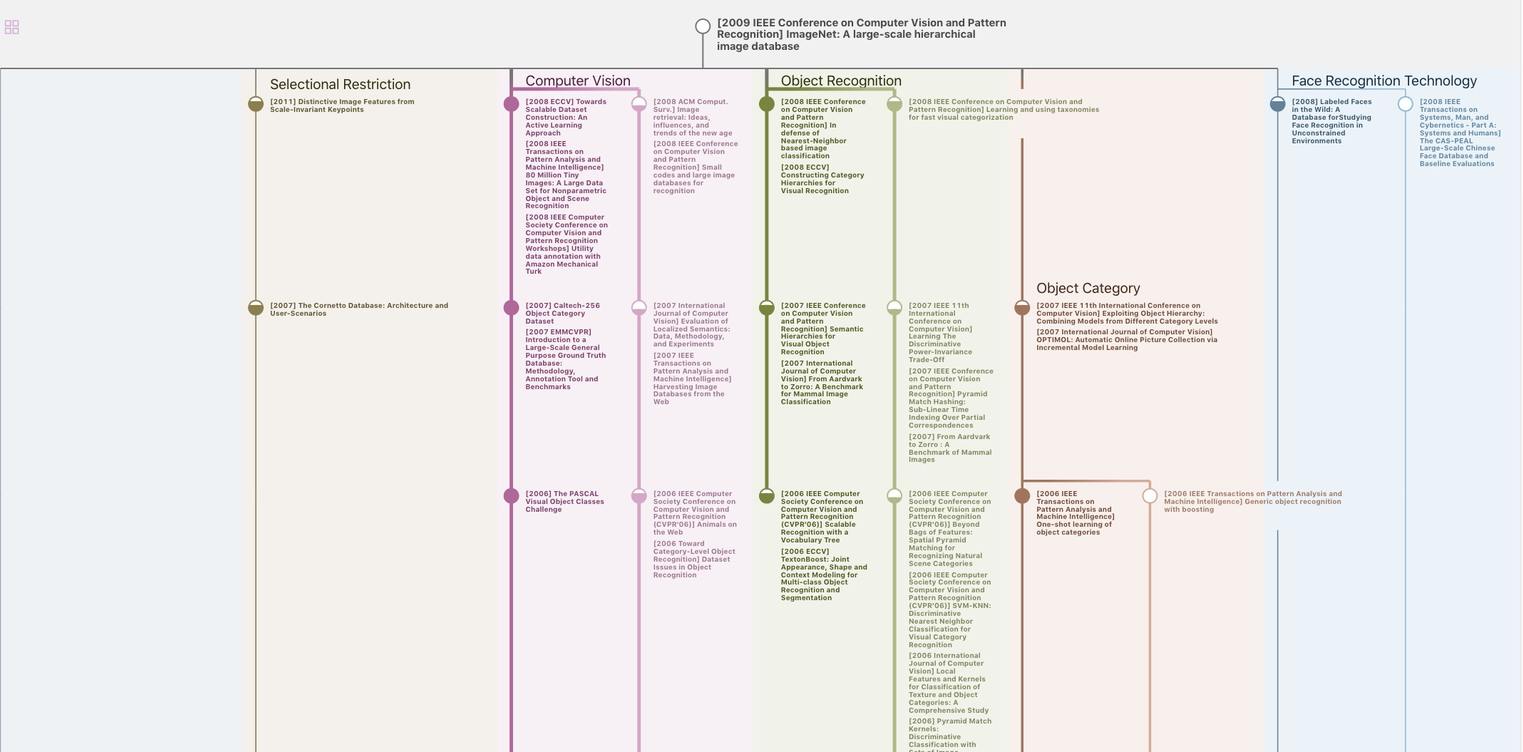
生成溯源树,研究论文发展脉络
Chat Paper
正在生成论文摘要