The neural architecture of language: Integrative reverse engineering converges on a model for predictive processing
bioRxiv(2020)
摘要
The neuroscience of perception has recently been revolutionized with an integrative reverse-engineering approach in which computation, brain function, and behavior are linked across many different datasets and many computational models. We here present a first systematic study taking this approach into higher-level cognition: human language processing, our species9 signature cognitive skill. We find that the most powerful 9transformer9 networks predict neural responses at nearly 100% and generalize across different datasets and data types (fMRI, ECoG). Across models, significant correlations are observed among all three metrics of performance: neural fit, fit to behavioral responses, and accuracy on the next-word prediction task (but not other language tasks), consistent with the long-standing hypothesis that the brain9s language system is optimized for predictive processing. Model architectures with initial weights further perform surprisingly similar to final trained models, suggesting that inherent structure - and not just experience with language - crucially contributes to a model9s match to the brain.
更多查看译文
AI 理解论文
溯源树
样例
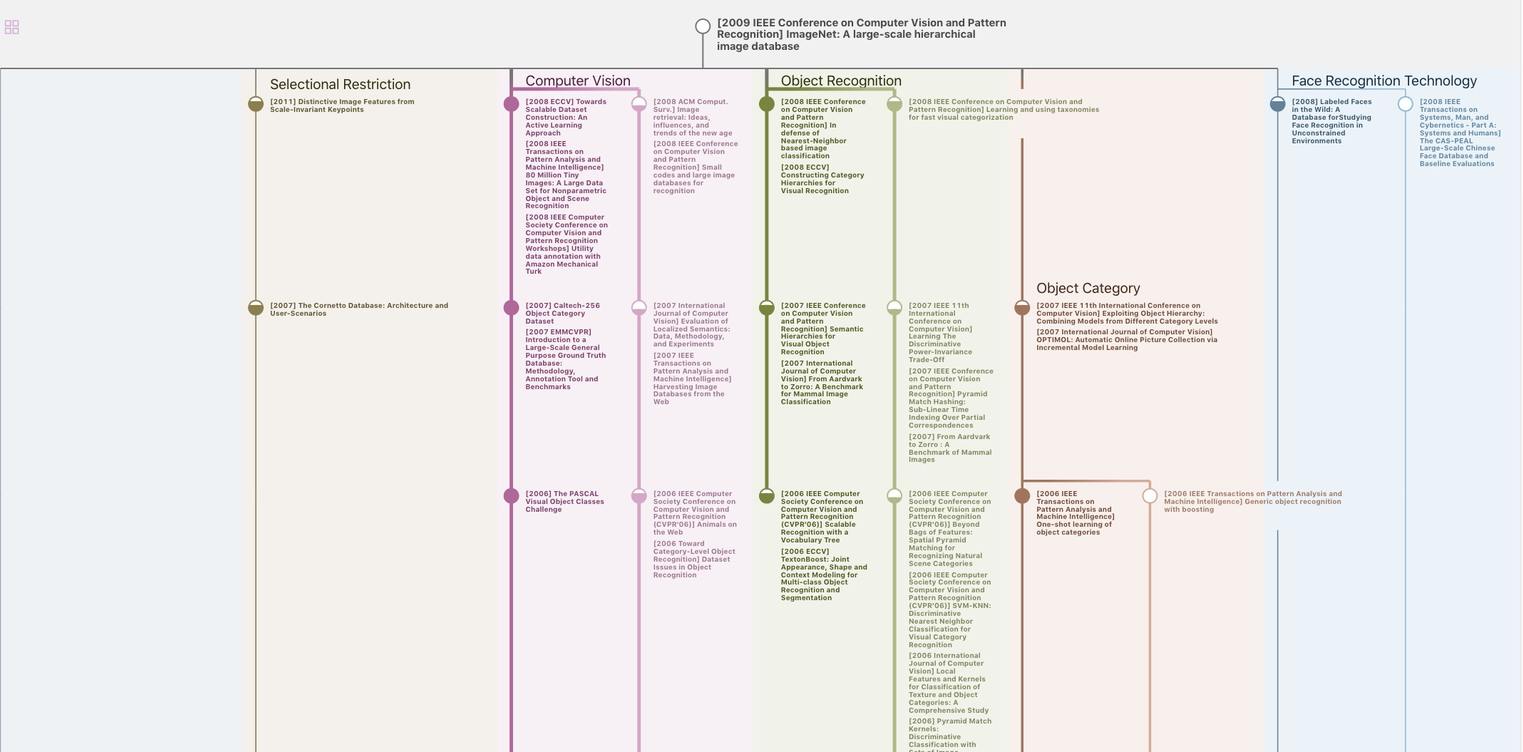
生成溯源树,研究论文发展脉络
Chat Paper
正在生成论文摘要