Boosted top quark tagging and polarization measurement using machine learning
PHYSICAL REVIEW D(2022)
摘要
Machine learning techniques are used for treating jets as images to explore the performance of boosted top quark tagging. Tagging performances are studied in both hadronic and leptonic channels of top quark decay, employing a convolutional neural network (CNN) based technique along with boosted decision trees (BDT). This computer vision approach is also applied to distinguish between left and right polarized top quarks. In this context, an experimentally measurable asymmetry variable is proposed to estimate the polarization. Results indicate that the CNN based classifier is more sensitive to top quark polarization than the standard kinematic variables. It is observed that the overall tagging performance in the leptonic channel is better than the hadronic case, and the former also serves as a better probe for studying polarization.
更多查看译文
AI 理解论文
溯源树
样例
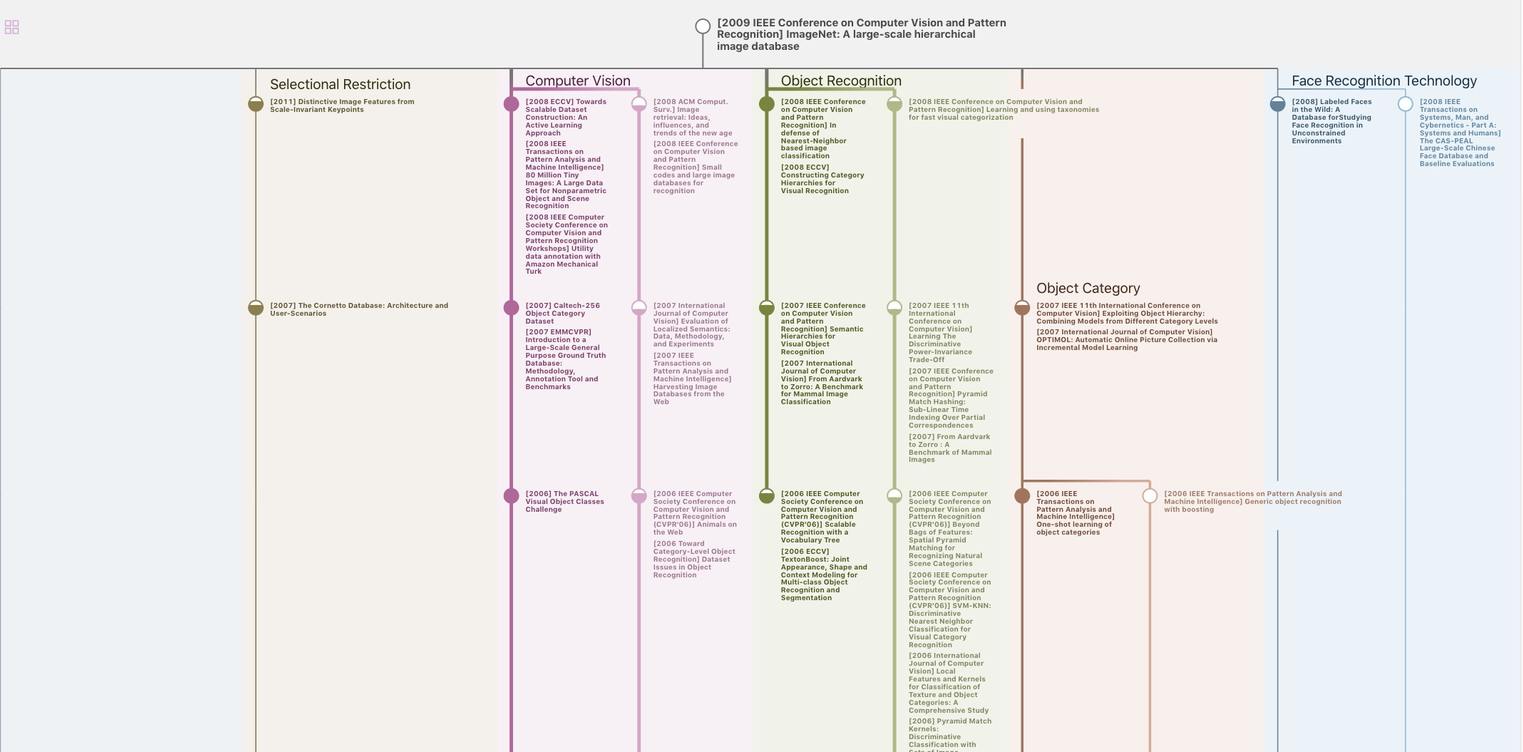
生成溯源树,研究论文发展脉络
Chat Paper
正在生成论文摘要