A Multi-Modal Approach for Driver Gaze Prediction to Remove Identity Bias
ICMI '20: INTERNATIONAL CONFERENCE ON MULTIMODAL INTERACTION Virtual Event Netherlands October, 2020(2020)
摘要
Driver gaze prediction is an important task in Advanced Driver Assistance System (ADAS). Although the Convolutional Neural Network (CNN) can greatly improve the recognition ability, there are still several unsolved problems due to the challenge of illumination, pose and camera placement. To solve these difficulties, we propose an effective multi-model fusion method for driver gaze estimation. Rich appearance representations, i.e. holistic and eyes regions, and geometric representations, i.e. landmarks and Delaunay angles, are separately learned to predict the gaze, followed by a score-level fusion system. Moreover, pseudo-3D appearance supervision and identity-adaptive geometric normalization are proposed to further enhance the prediction accuracy. Finally, the proposed method achieves state-of-the-art accuracy of 82.5288% on the test data, which ranks 1st at the EmotiW2020 driver gaze prediction sub-challenge.
更多查看译文
AI 理解论文
溯源树
样例
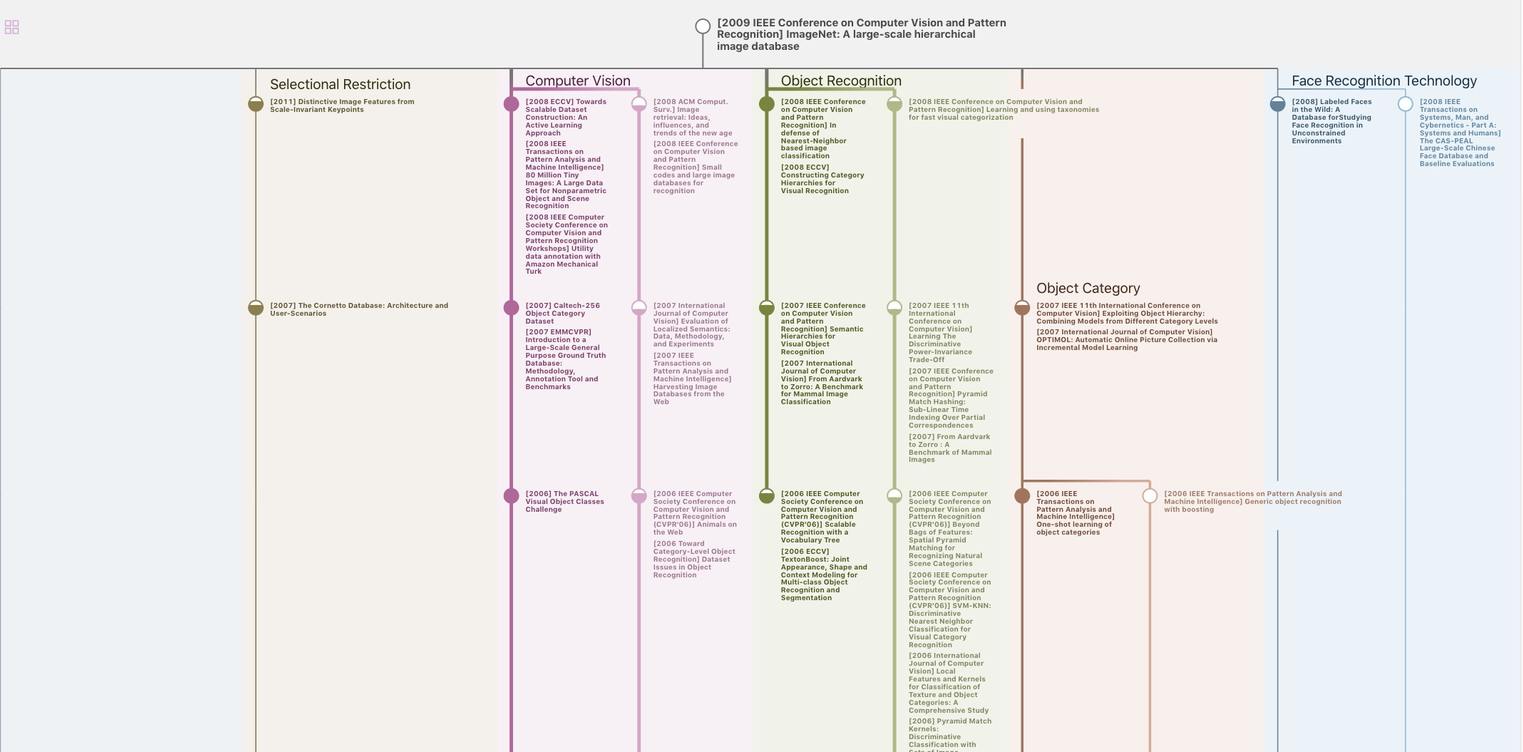
生成溯源树,研究论文发展脉络
Chat Paper
正在生成论文摘要