A UNIFIED APPROACH TO TRANSLATE CLASSICAL BANDIT ALGORITHMS TO STRUCTURED BANDITS
IEEE Journal on Selected Areas in Information Theory(2021)
摘要
We consider a finite-armed structured bandit problem in which mean rewards of different arms are known functions of a common hidden parameter theta*. This problem setting subsumes several previously studied frameworks that assume linear or invertible reward functions. We propose a novel approach to gradually estimate the hidden theta* and use the estimate together with the mean reward functions to substantially reduce exploration of sub-optimal arms. This approach enables us to fundamentally generalize any classic bandit algorithm including UCB and Thompson Sampling to the structured bandit setting. We prove via regret analysis that our proposed UCB-C and TS-C algorithms (structured bandit versions of UCB and Thompson Sampling, respectively) pull only a subset of the sub-optimal arms O(log T) times while the other sub-optimal arms (referred to as non-competitive arms) are pulled O(1) times. As a result, in cases where all sub-optimal arms are non-competitive, which can happen in many practical scenarios, the proposed algorithms achieve bounded regret.
更多查看译文
关键词
Multi-Armed Bandits, Sequential decision making, Online learning, Statistical learning, Regret bounds
AI 理解论文
溯源树
样例
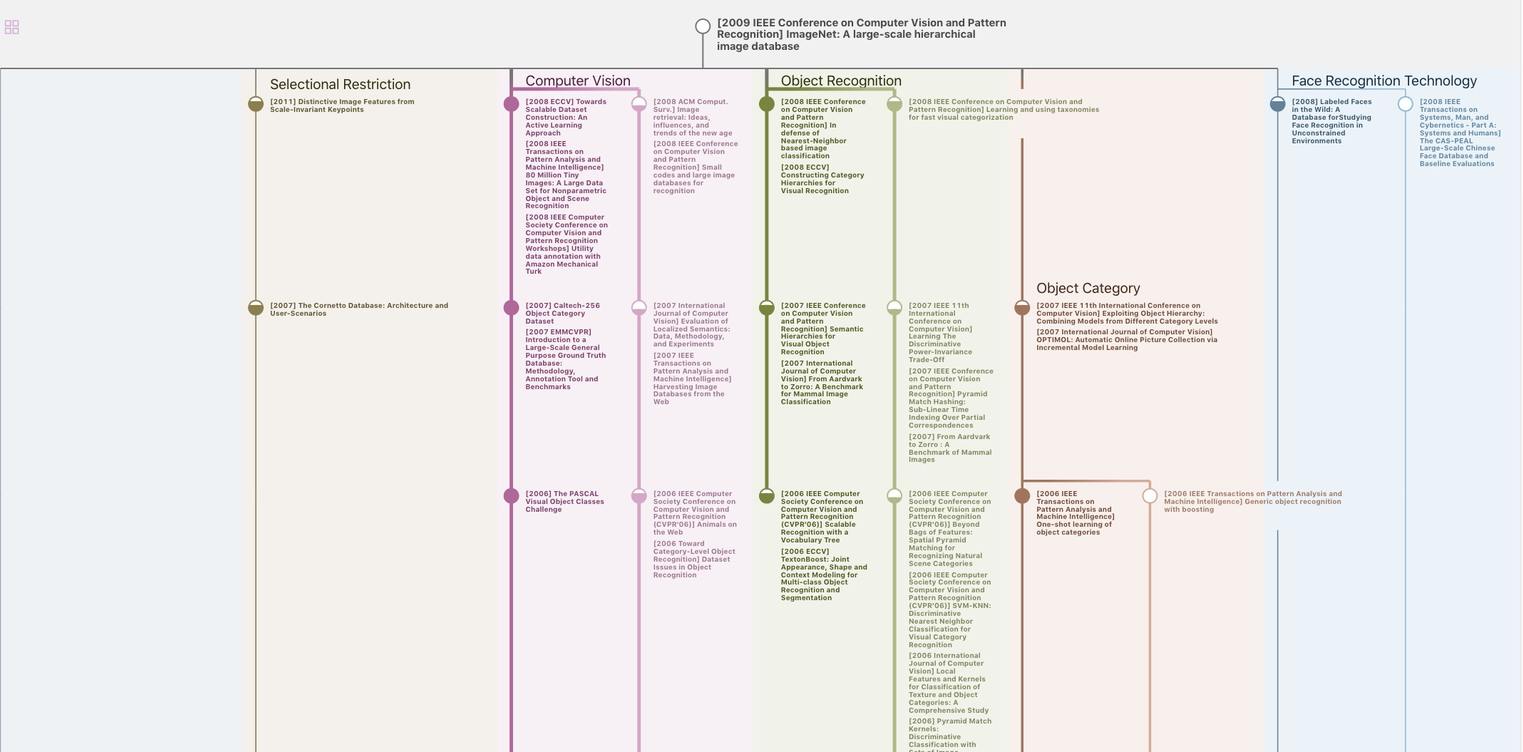
生成溯源树,研究论文发展脉络
Chat Paper
正在生成论文摘要