CLASS-CONDITIONAL DEFENSE GAN AGAINST END-TO-END SPEECH ATTACKS
2021 IEEE INTERNATIONAL CONFERENCE ON ACOUSTICS, SPEECH AND SIGNAL PROCESSING (ICASSP 2021)(2021)
摘要
In this paper we propose a novel defense approach against end-to-end adversarial attacks developed to fool advanced speech-to-text systems such as DeepSpeech and Lingvo. Unlike conventional defense approaches, the proposed approach does not directly employ low-level transformations such as autoencoding a given input signal aiming at removing potential adversarial perturbation. Instead of that, we find an optimal input vector for a class conditional generative adversarial network through minimizing the relative chordal distance adjustment between a given test input and the generator network. Then, we reconstruct the 1D signal from the synthesized spectrogram and the original phase information derived from the given input signal. Hence, this reconstruction does not add any extra noise to the signal and according to our experimental results, our defense-GAN considerably outperforms conventional defense algorithms both in terms of word error rate and sentence level recognition accuracy.
更多查看译文
关键词
Speech processing, Schur decomposition, chordal distance, adversarial subspace, adversarial defense
AI 理解论文
溯源树
样例
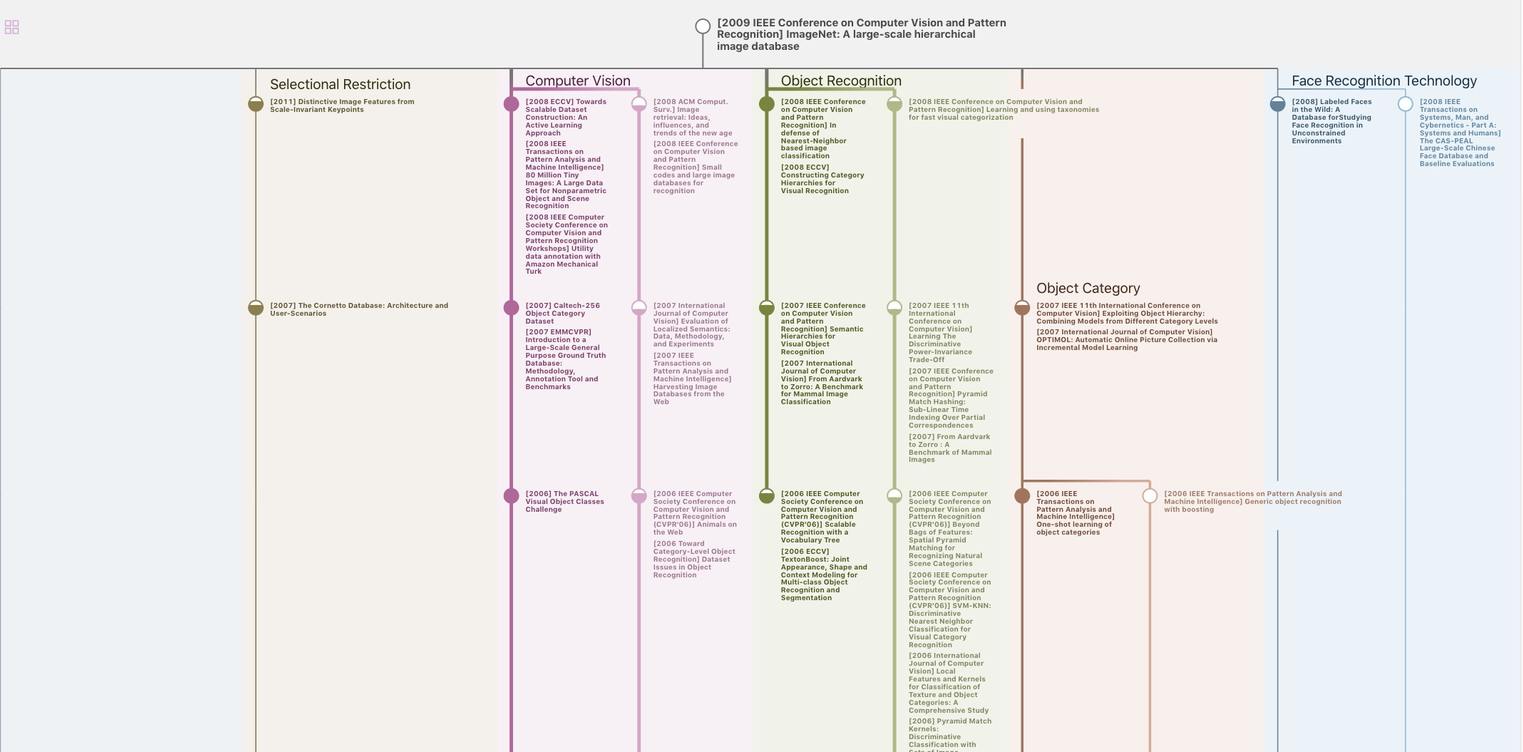
生成溯源树,研究论文发展脉络
Chat Paper
正在生成论文摘要