UNROLLING OF DEEP GRAPH TOTAL VARIATION FOR IMAGE DENOISING
2021 IEEE INTERNATIONAL CONFERENCE ON ACOUSTICS, SPEECH AND SIGNAL PROCESSING (ICASSP 2021)(2021)
摘要
While deep learning (DL) architectures like convolutional neural networks (CNNs) have enabled effective solutions in image denoising, in general their implementations overly rely on training data, lack interpretability, and require tuning of a large parameter set. In this paper, we combine classical graph signal filtering with deep feature learning into a competitive hybrid design-one that utilizes interpretable analytical low-pass graph filters and employs 80% fewer network parameters than state-of-the-art DL denoising scheme DnCNN. Specifically, to construct a suitable similarity graph for graph spectral filtering, we first adopt a CNN to learn feature representations per pixel, and then compute feature distances to establish edge weights. Given a constructed graph, we next formulate a convex optimization problem for denoising using a graph total variation (GTV) prior. Via a l(1) graph Laplacian reformulation, we interpret its solution in an iterative procedure as a graph low-pass filter and derive its frequency response. For fast filter implementation, we realize this response using a Lanczos approximation. Experimental results show that in the case of statistical mistmatch, our algorithm outperformed DnCNN by up to 3dB in PSNR.
更多查看译文
关键词
image denoising, graph signal processing, deep learning
AI 理解论文
溯源树
样例
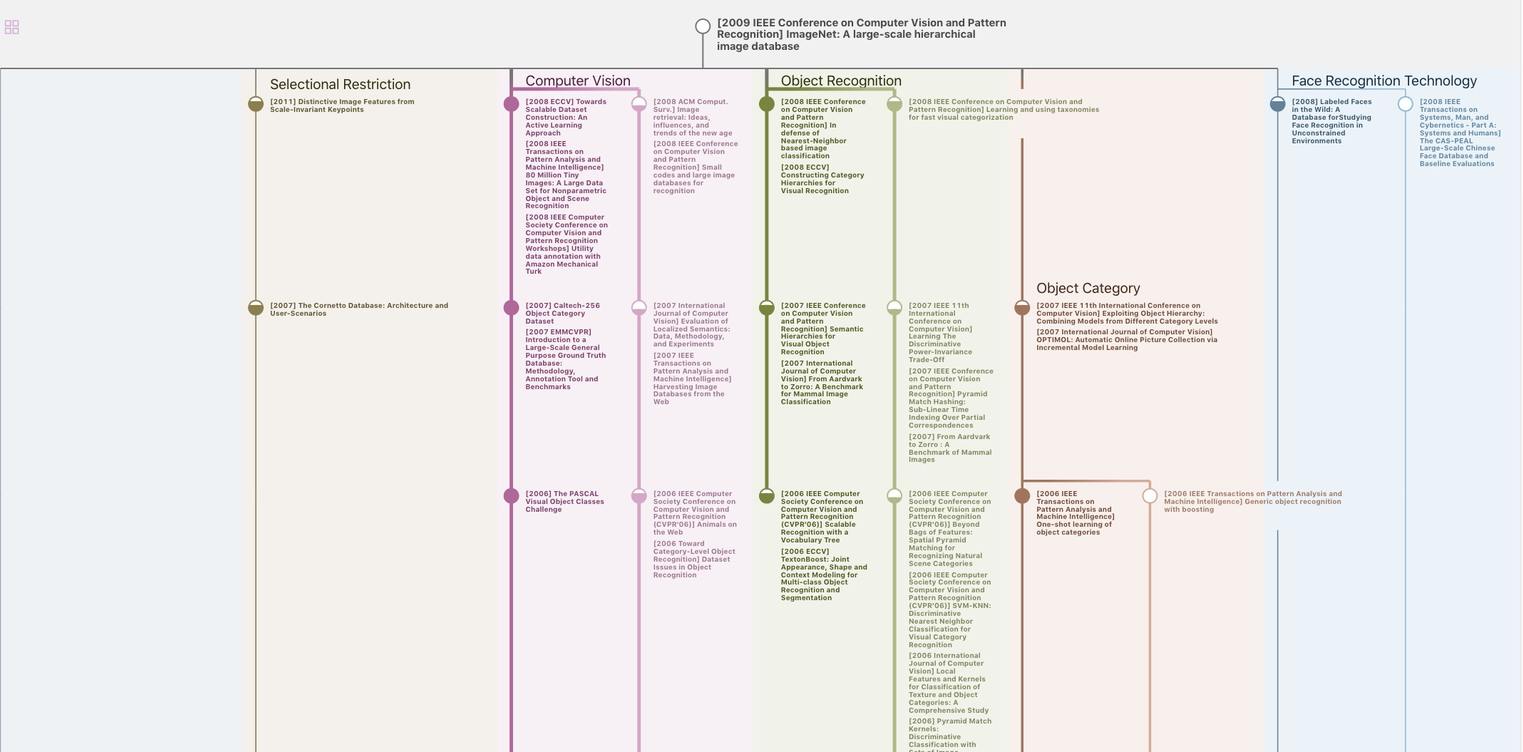
生成溯源树,研究论文发展脉络
Chat Paper
正在生成论文摘要