Box2Seg: Attention Weighted Loss and Discriminative Feature Learning for Weakly Supervised Segmentation
European Conference on Computer Vision(2020)
摘要
We propose a weakly supervised approach to semantic segmentation using bounding box annotations. Bounding boxes are treated as noisy labels for the foreground objects. We predict a per-class attention map that saliently guides the per-pixel cross entropy loss to focus on foreground pixels and refines the segmentation boundaries. This avoids propagating erroneous gradients due to incorrect foreground labels on the background. Additionally, we learn pixel embeddings to simultaneously optimize for high intra-class feature affinity while increasing discrimination between features across different classes. Our method, Box2Seg, achieves state-of-the-art segmentation accuracy on PASCAL VOC 2012 by significantly improving the mIOU metric by \(2.1\%\) compared to previous weakly supervised approaches. Our weakly supervised approach is comparable to the recent fully supervised methods when fine-tuned with limited amount of pixel-level annotations. Qualitative results and ablation studies show the benefit of different loss terms on the overall performance.
更多查看译文
关键词
attention weighted loss,segmentation,discriminative feature learning
AI 理解论文
溯源树
样例
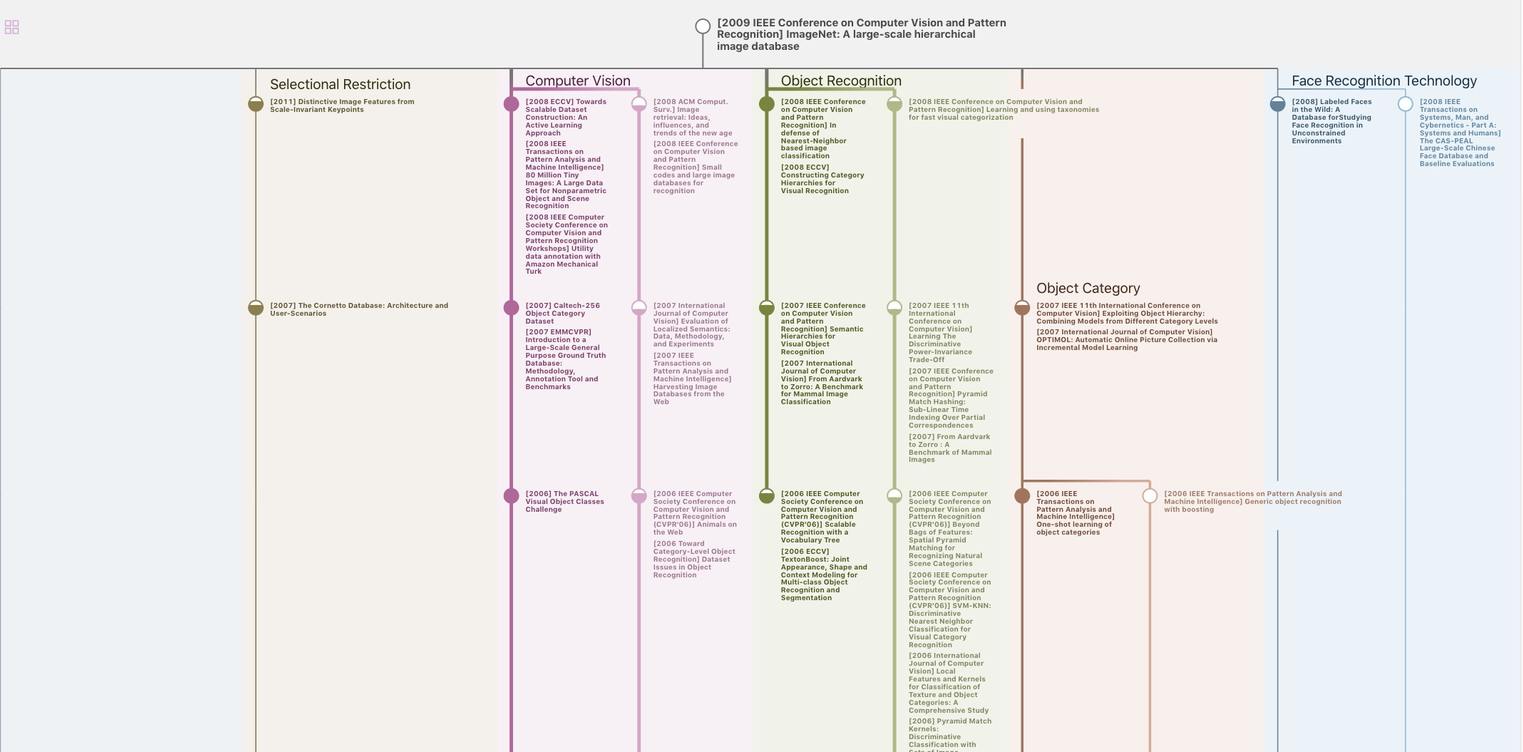
生成溯源树,研究论文发展脉络
Chat Paper
正在生成论文摘要