Riemannian Langevin algorithm for solving semidefinite programs
BERNOULLI(2023)
摘要
We propose a Langevin diffusion-based algorithm for non-convex optimization and sampling on a product manifold of spheres. Under a logarithmic Sobolev inequality, we establish a guarantee for finite iteration convergence to the Gibbs distribution in terms of Kullback-Leibler divergence. We show that with an appropriate temperature choice, the suboptimality gap to the global minimum is guaranteed to be arbitrarily small with high probability. As an application, we consider the Burer-Monteiro approach for solving a semidefinite program (SDP) with diagonal constraints, and analyze the proposed Langevin algorithm for optimizing the non-convex objective. In particular, we establish a logarithmic Sobolev inequality for the Burer-Monteiro problem when there are no spurious local minima, but under the presence saddle points. Combining the results, we then provide a global optimality guarantee for the SDP and the Max-Cut problem. More precisely, we show that the Langevin algorithm achieves epsilon accuracy with high probability in (Omega) over tilde (epsilon(-4.5)) iterations.
更多查看译文
关键词
Riemannian Langevin algorithm,non-convex optimization,logarithmic Sobolev inequality,semidefinite programs,Burer-Monteiro problem
AI 理解论文
溯源树
样例
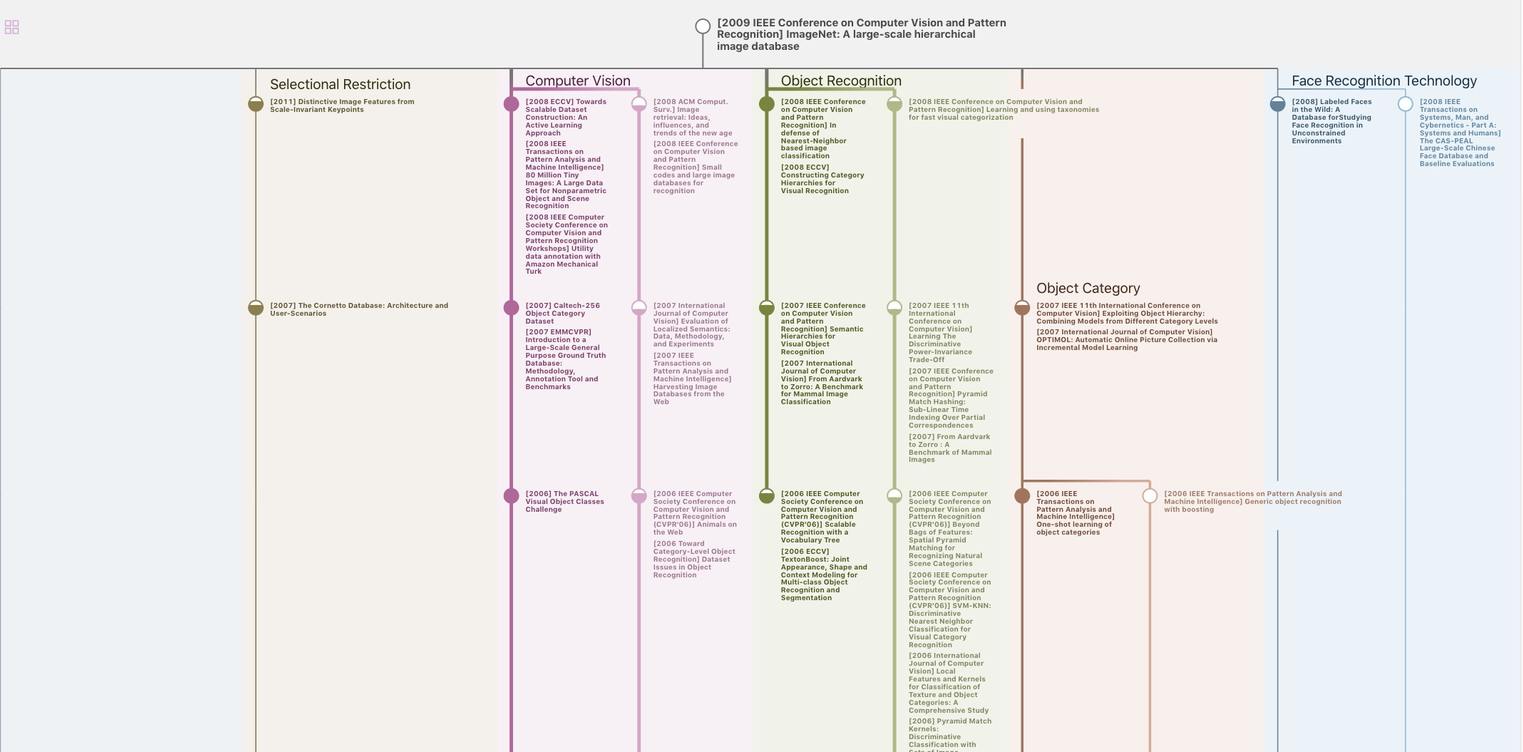
生成溯源树,研究论文发展脉络
Chat Paper
正在生成论文摘要