DuoRAT: Towards Simpler Text-to-SQL Models
NAACL-HLT(2020)
摘要
Recent research has shown that neural text-to-SQL models can effectively translate natural language questions into corresponding SQL queries on unseen databases. Working mostly on the Spider dataset, researchers have been proposing increasingly sophisticated modelling approaches to the problem. Contrary to this trend, in this paper we identify the aspects in which text-to-SQL models can be simplified. We begin by building DuoRAT, a re-implementation of the state-of-the-art RAT-SQL model that unlike RAT-SQL is using only relation-aware or vanilla transformers as the building blocks. We perform several ablation experiments using DuoRAT as the baseline model. Our experiments confirm the usefulness of some of the techniques and point out the redundancy of others, including structural SQL features and features that link the question with the schema.
更多查看译文
AI 理解论文
溯源树
样例
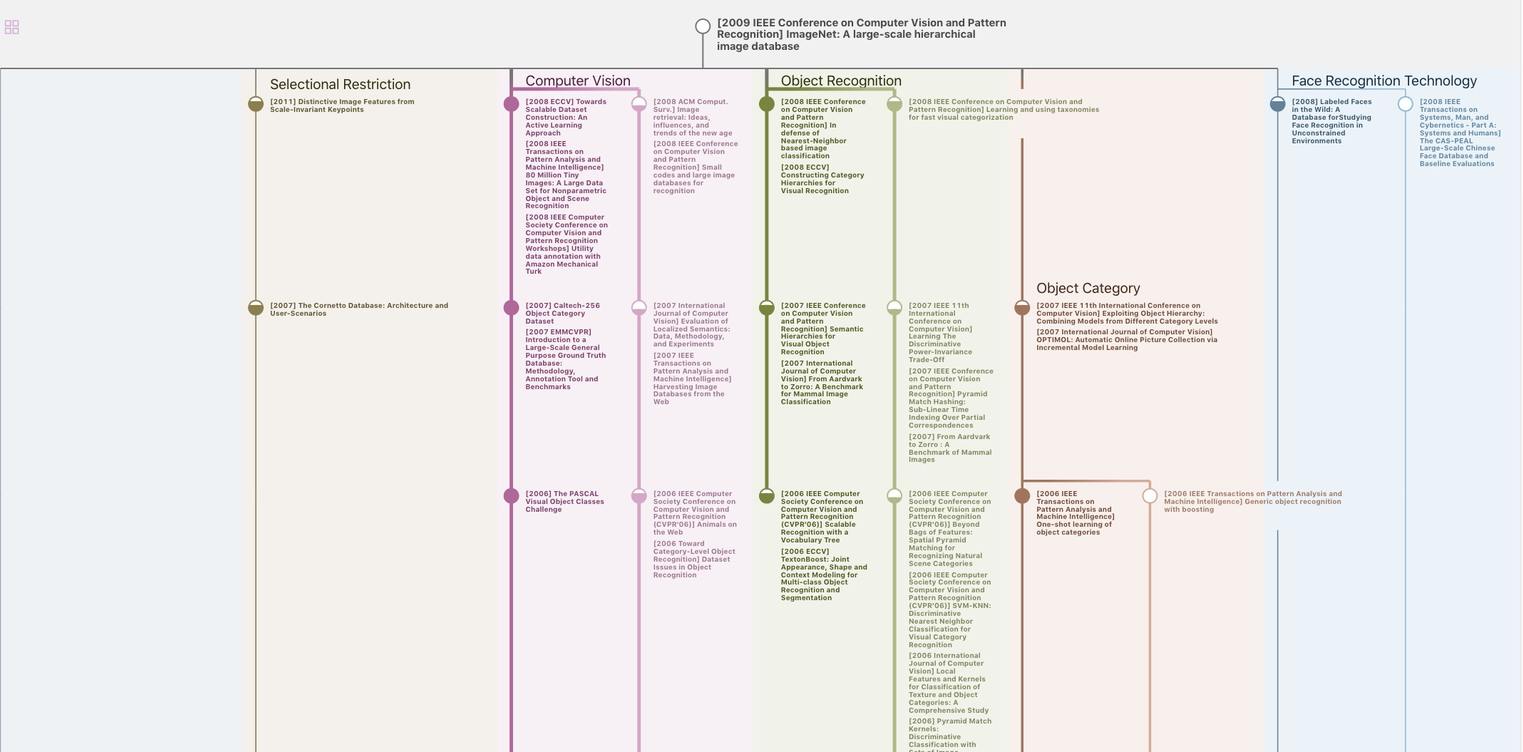
生成溯源树,研究论文发展脉络
Chat Paper
正在生成论文摘要