Pose Flow Learning From Person Images For Pose Guided Synthesis
IEEE TRANSACTIONS ON IMAGE PROCESSING(2021)
摘要
Pose guided synthesis aims to generate a new image in an arbitrary target pose while preserving the appearance details from the source image. Existing approaches rely on either hard-coded spatial transformations or 3D body modeling. They often overlook complex non-rigid pose deformation or unmatched occluded regions, thus fail to effectively preserve appearance information. In this article, we propose a pose flow learning scheme that learns to transfer the appearance details from the source image without resorting to annotated correspondences. Based on such learned pose flow, we proposed GarmentNet and SynthesisNet, both of which use multi-scale feature-domain alignment for coarse-to-fine synthesis. Experiments on the DeepFashion, MVC dataset and additional real-world datasets demonstrate that our approach compares favorably with the state-of-the-art methods and generalizes to unseen poses and clothing styles.
更多查看译文
关键词
Clothing, Strain, Image synthesis, Training, Annotations, Optical imaging, Faces, Pose guided synthesis, pose correspondence, optical flow learning
AI 理解论文
溯源树
样例
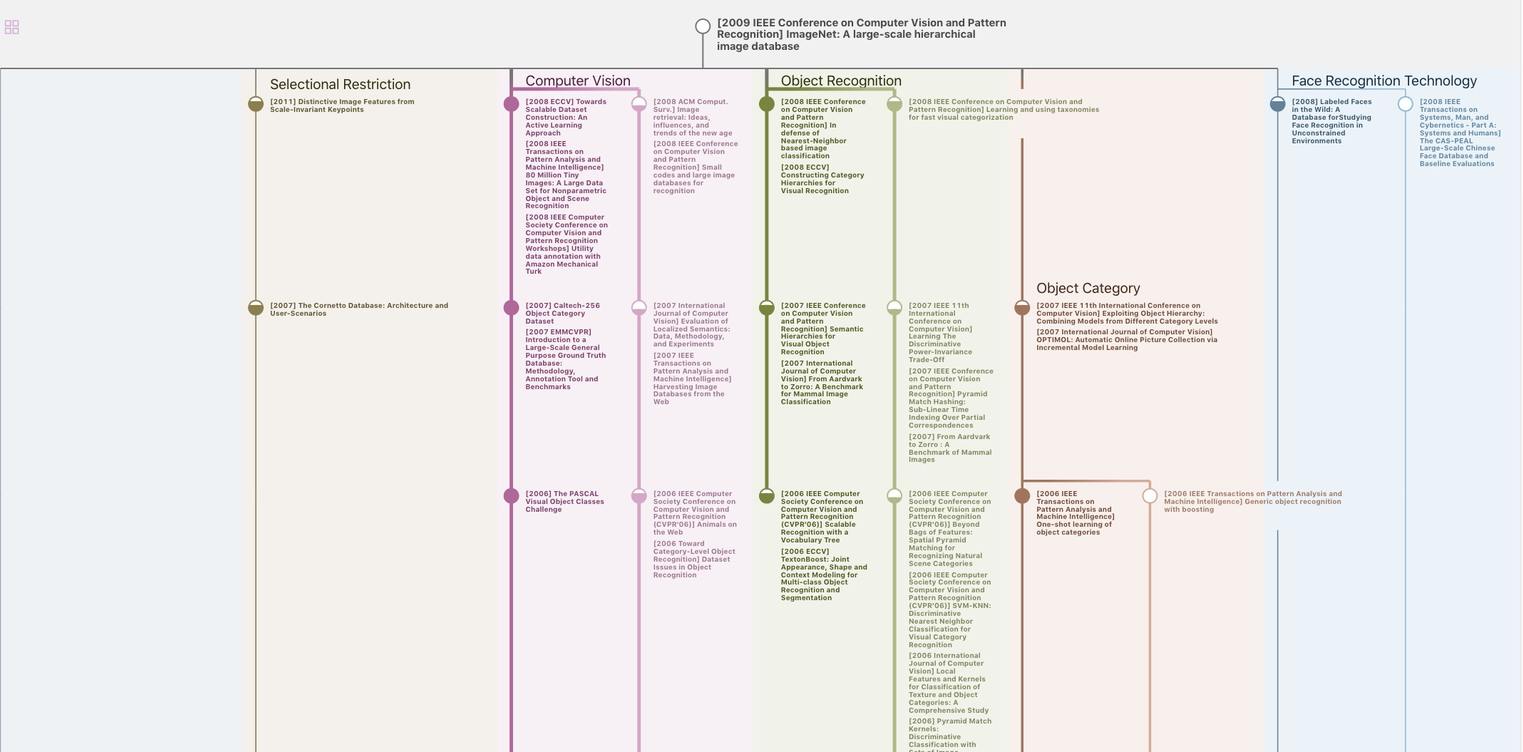
生成溯源树,研究论文发展脉络
Chat Paper
正在生成论文摘要