Semantics-Guided Representation Learning With Applications To Visual Synthesis
2020 25TH INTERNATIONAL CONFERENCE ON PATTERN RECOGNITION (ICPR)(2020)
摘要
Learning interpretable and interpolatable latent representations has been an emerging research direction, allowing researchers to understand and utilize the derived latent space for further applications such as visual synthesis or recognition. While most existing approaches derive an interpolatable latent space and induces smooth transition in image appearance, it is still not clear how to observe desirable representations which would contain semantic information of interest. In this paper, we aim to learn meaningful representations and simultaneously perform semantic-oriented and visually-smooth interpolation. To this end, we propose an angular triplet-neighbor loss (ATNL) that enables learning a latent representation whose distribution matches the semantic information of interest. With the latent space guided by ATNL, we further utilize spherical semantic interpolation for generating semantic warping of images, allowing synthesis of desirable visual data. Experiments on MNIST and CMU Multi-PIE datasets qualitatively and quantitatively verily the effectiveness of our method.
更多查看译文
关键词
Representation learning, Semantic interpolation
AI 理解论文
溯源树
样例
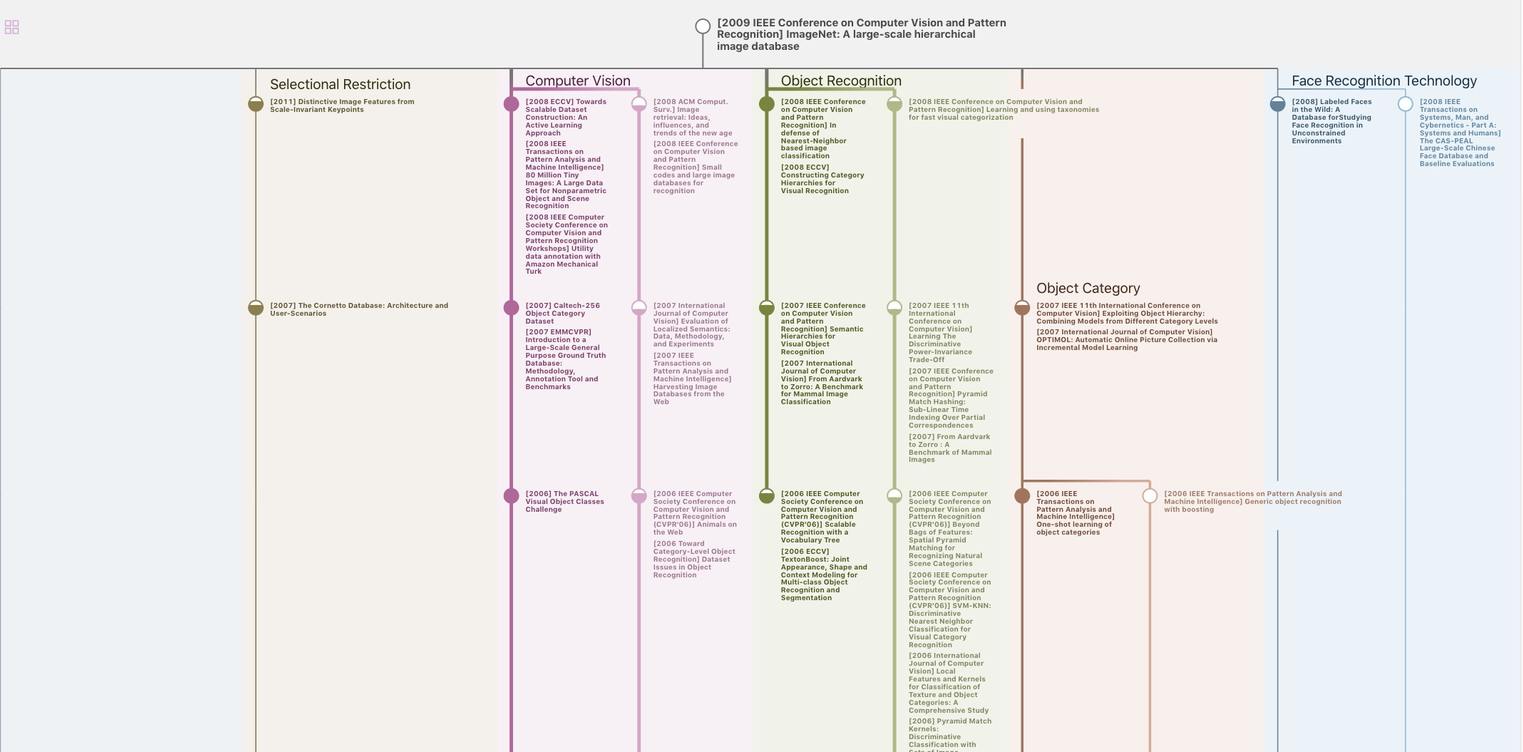
生成溯源树,研究论文发展脉络
Chat Paper
正在生成论文摘要