EMFORMER: EFFICIENT MEMORY TRANSFORMER BASED ACOUSTIC MODEL FOR LOW LATENCY STREAMING SPEECH RECOGNITION
2021 IEEE INTERNATIONAL CONFERENCE ON ACOUSTICS, SPEECH AND SIGNAL PROCESSING (ICASSP 2021)(2021)
摘要
This paper proposes an efficient memory transformer Emformer for low latency streaming speech recognition. In Emformer, the long-range history context is distilled into an augmented memory bank to reduce self-attention's computation complexity. A cache mechanism saves the computation for the key and value in self-attention for the left context. Emformer applies a parallelized block processing in training to support low latency models. We carry out experiments on benchmark LibriSpeech data. Under average latency of 960 ms, Emformer gets WER 2:50% on test-clean and 5:62% on test-other. Comparing with a strong baseline augmented memory transformer (AM-TRF), Emformer gets 4:6 folds training speedup and 18% relative real-time factor (RTF) reduction in decoding with relative WER reduction 17% on test-clean and 9% on test-other. For a low latency scenario with an average latency of 80 ms, Emformer achieves WER 3:01% on test-clean and 7:09% on test-other. Comparing with the LSTM baseline with the same latency and model size, Emformer gets relative WER reduction 9% and 16% on test-clean and test-other, respectively.
更多查看译文
关键词
Low Latency, Transformer, Emformer
AI 理解论文
溯源树
样例
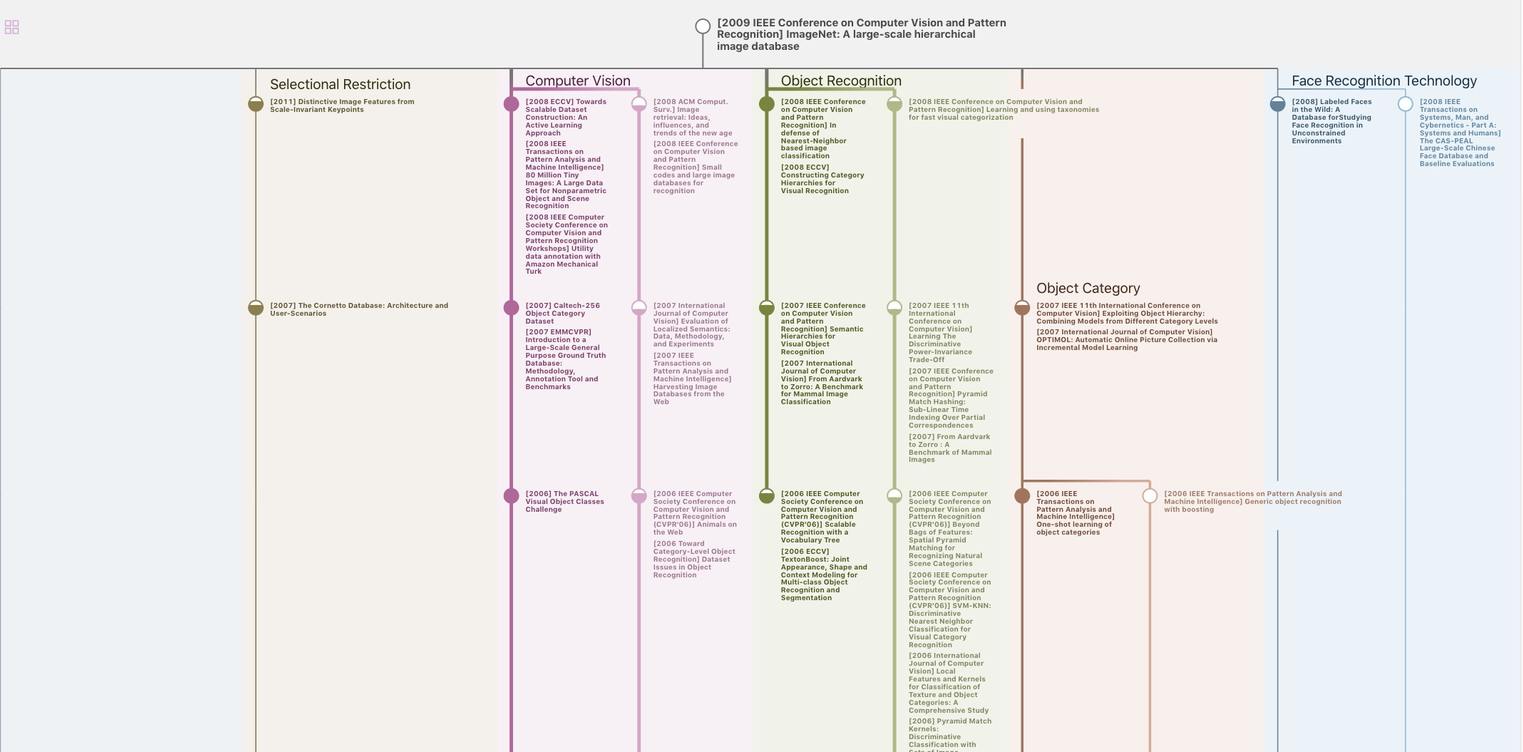
生成溯源树,研究论文发展脉络
Chat Paper
正在生成论文摘要