Attention Based Graph Evolution
Pacific-Asia Conference on Knowledge Discovery and Data Mining(2020)
摘要
Based on the recent success of deep generative models on continuous data, various new methods are being developed to generate discrete data such as graphs. However, these approaches focus on unconditioned generation, which limits their control over the generating procedure to produce graphs in context, thus limiting the applicability to real-world settings. To address this gap, we introduce an attention-based graph evolution model (AGE). AGE is a conditional graph generator based on the neural attention mechanism that can not only model graph evolution in both space and time, but can also model the transformation between graphs from one state to another. We evaluate AGE on multiple conditional graph-generation tasks, and our results show that it can generate realistic graphs conditioned on source graphs, outperforming existing methods in terms of quality and generality.
更多查看译文
关键词
Conditional graph generation, Attention, Graph evolution
AI 理解论文
溯源树
样例
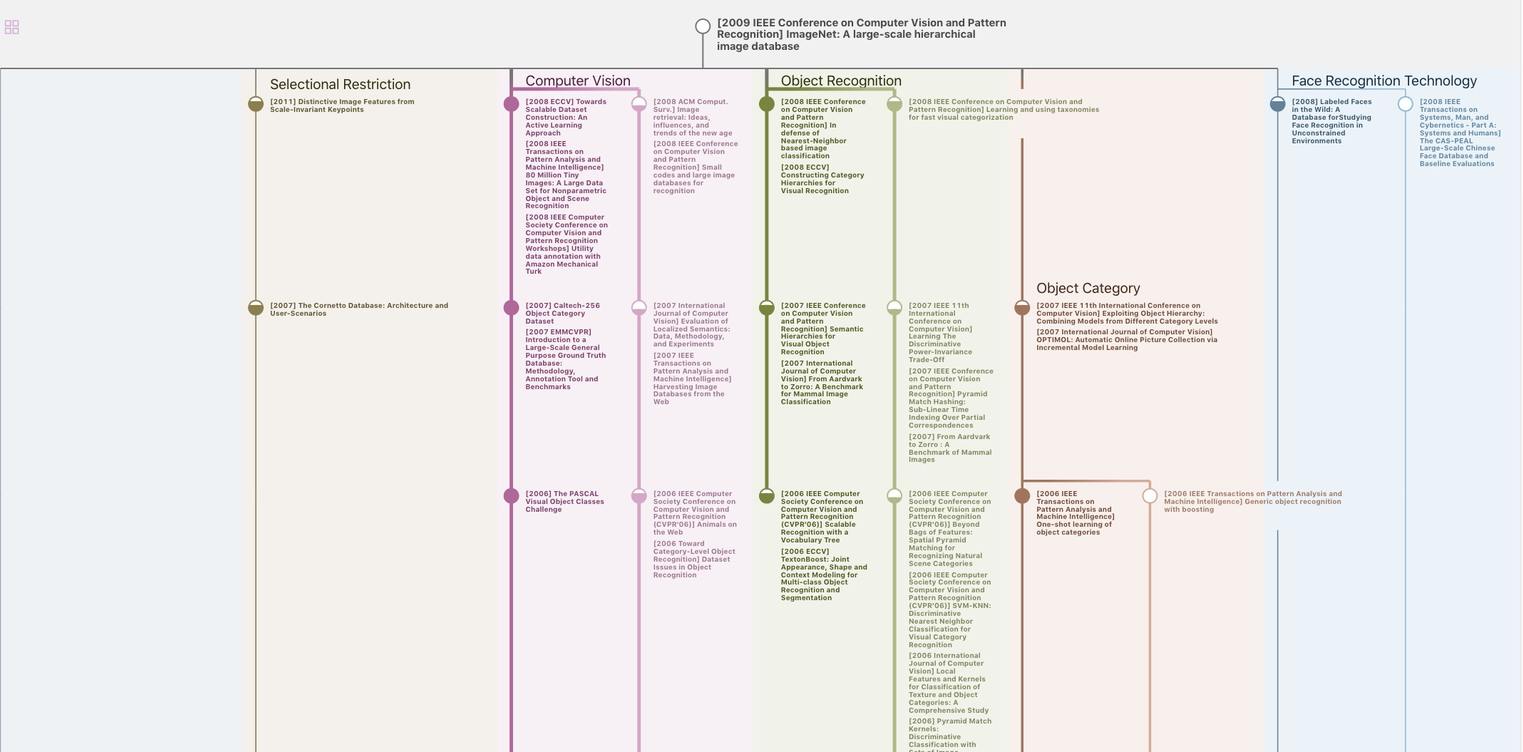
生成溯源树,研究论文发展脉络
Chat Paper
正在生成论文摘要