A Fourier based Approach to Generalization and Optimization in Deep Learning
IEEE Journal on Selected Areas in Information Theory(2020)
摘要
The success of deep neural networks stems from their ability to generalize well on real data; however, Zhang et al. zhang2016understanding have observed that neural networks can easily overfit randomly-generated labels. This observation highlights the following question: why do gradient methods succeed in finding generalizable solutions for neural networks while there exist solutions with poor generalization behavior? In this work, we use a Fourier-based approach to study the generalization properties of gradient-based methods over 2-layer neural networks with band-limited activation functions. Our results indicate that in such settings if the underlying distribution of data enjoys nice Fourier properties including bandlimitedness and bounded Fourier norm, then the gradient descent method can converge to local minima with nice generalization behavior. We also establish a Fourier-based generalization error …
更多查看译文
关键词
Deep learning,Fourier analysis,generalization bounds,norm-based regularization
AI 理解论文
溯源树
样例
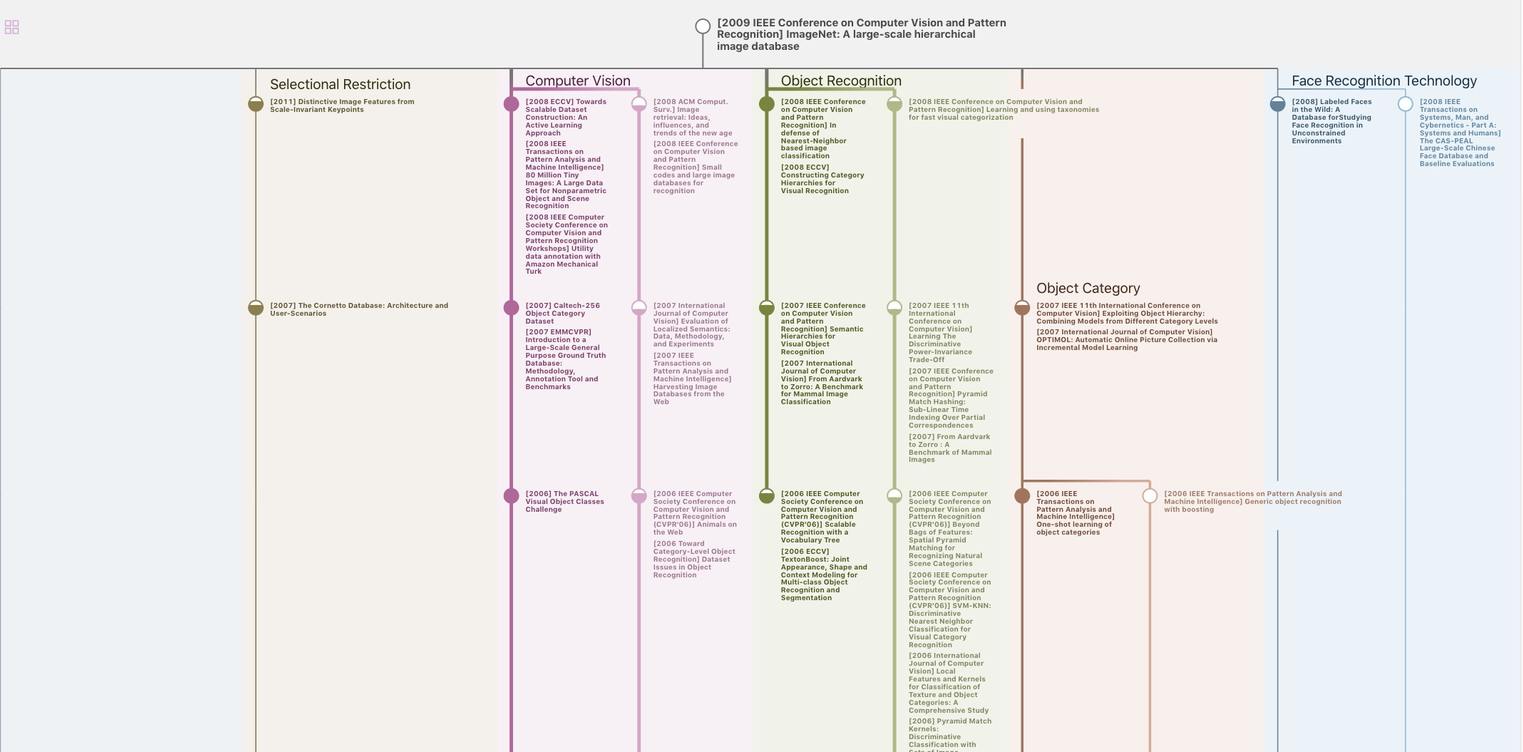
生成溯源树,研究论文发展脉络
Chat Paper
正在生成论文摘要