Time-varying forecast combination for high-dimensional data
JOURNAL OF ECONOMETRICS(2023)
摘要
In this paper, we propose a new nonparametric estimator of time-varying forecast combination weights. When the number of individual forecasts is small, we study the asymptotic properties of the local linear estimator. When the number of candidate forecasts exceeds or diverges with the sample size, we consider penalized local linear estimation with the group SCAD penalty. We show that the estimator exhibits the oracle property and correctly selects relevant forecasts with probability approaching one. Simulations indicate that the proposed estimators outperform existing combination schemes when structural changes exist. An empirical application on inflation and unemployment forecasting highlights the merits of our approach relative to other popular methods in the literature.(c) 2023 Elsevier B.V. All rights reserved.
更多查看译文
关键词
Cross validation,Forecast combination,High dimension,Local linear estimation,SCAD,Sparsity
AI 理解论文
溯源树
样例
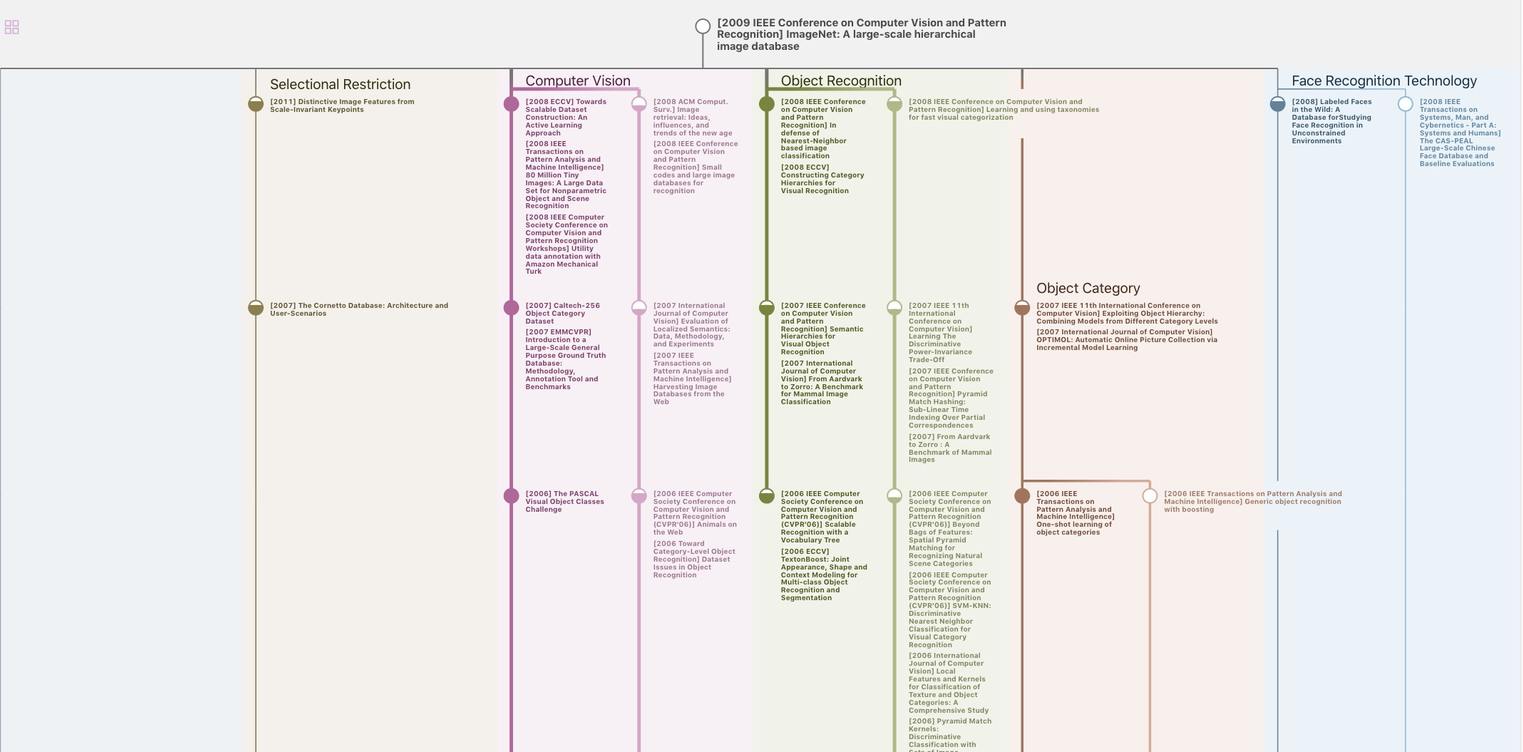
生成溯源树,研究论文发展脉络
Chat Paper
正在生成论文摘要