Graph based optimization approaches for machine learning, uncertainty quantification and networks
Handbook of Numerical Analysis(2019)
摘要
Recently, the graphical framework, which provides information about the connections between pieces of data via a similarity graph, has become a popular setting for problems involving high dimensional data. Moreover, some of the best performing graph-based methods consist of solving variational problems on graphs. In this chapter, we describe several recent graph-based optimization algorithms for applications such as data classification, uncertainty quantification and the social sciences. In particular, the data classification procedures we consider are derived using optimization techniques, including those involving the Ginzburg–Landau functional, the Merriman–Bence–Osher (MBO) scheme and total variation. Approaches to dealing with class size constraints are also included. Moreover, the uncertainty quantification algorithms we describe involve a Bayesian approach to uncertainty quantification for graph …
更多查看译文
关键词
Diffuse interfaces, Graphical models, Graph Laplacian, Machine learning, Uncertainty quantification, Social networks, Community detection, Data clustering, Modularity, MBO scheme
AI 理解论文
溯源树
样例
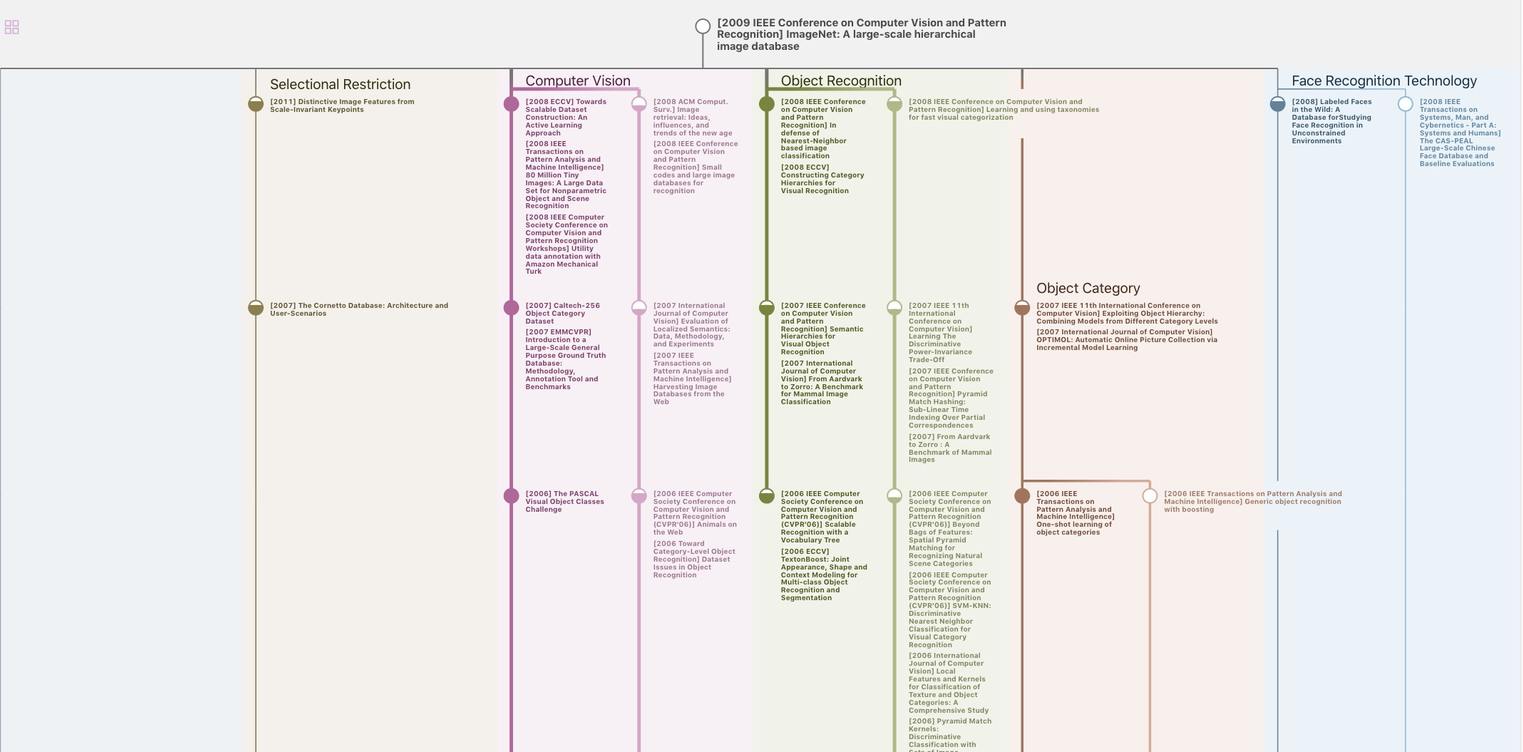
生成溯源树,研究论文发展脉络
Chat Paper
正在生成论文摘要