PseudoSeg: Designing Pseudo Labels for Semantic Segmentation
ICLR(2021)
摘要
Recent advances in semi-supervised learning (SSL) demonstrate that a combination of consistency regularization and pseudo-labeling can effectively improve image classification accuracy in the low-data regime. Compared to classification, semantic segmentation tasks require much more intensive labeling costs. Thus, these tasks greatly benefit from data-efficient training methods. However, structured outputs in segmentation render particular difficulties (e.g., designing pseudo-labeling and augmentation) to apply existing SSL strategies. To address this problem, we present a simple and novel re-design of pseudo-labeling to generate well-calibrated structured pseudo labels for training with unlabeled or weakly-labeled data. Our proposed pseudo-labeling strategy is network structure agnostic to apply in a one-stage consistency training framework. We demonstrate the effectiveness of the proposed pseudo-labeling strategy in both low-data and high-data regimes. Extensive experiments have validated that pseudo labels generated from wisely fusing diverse sources and strong data augmentation are crucial to consistency training for segmentation. The source code is available at https://github.com/googleinterns/wss.
更多查看译文
关键词
designing pseudoseg labels,semantic segmentation
AI 理解论文
溯源树
样例
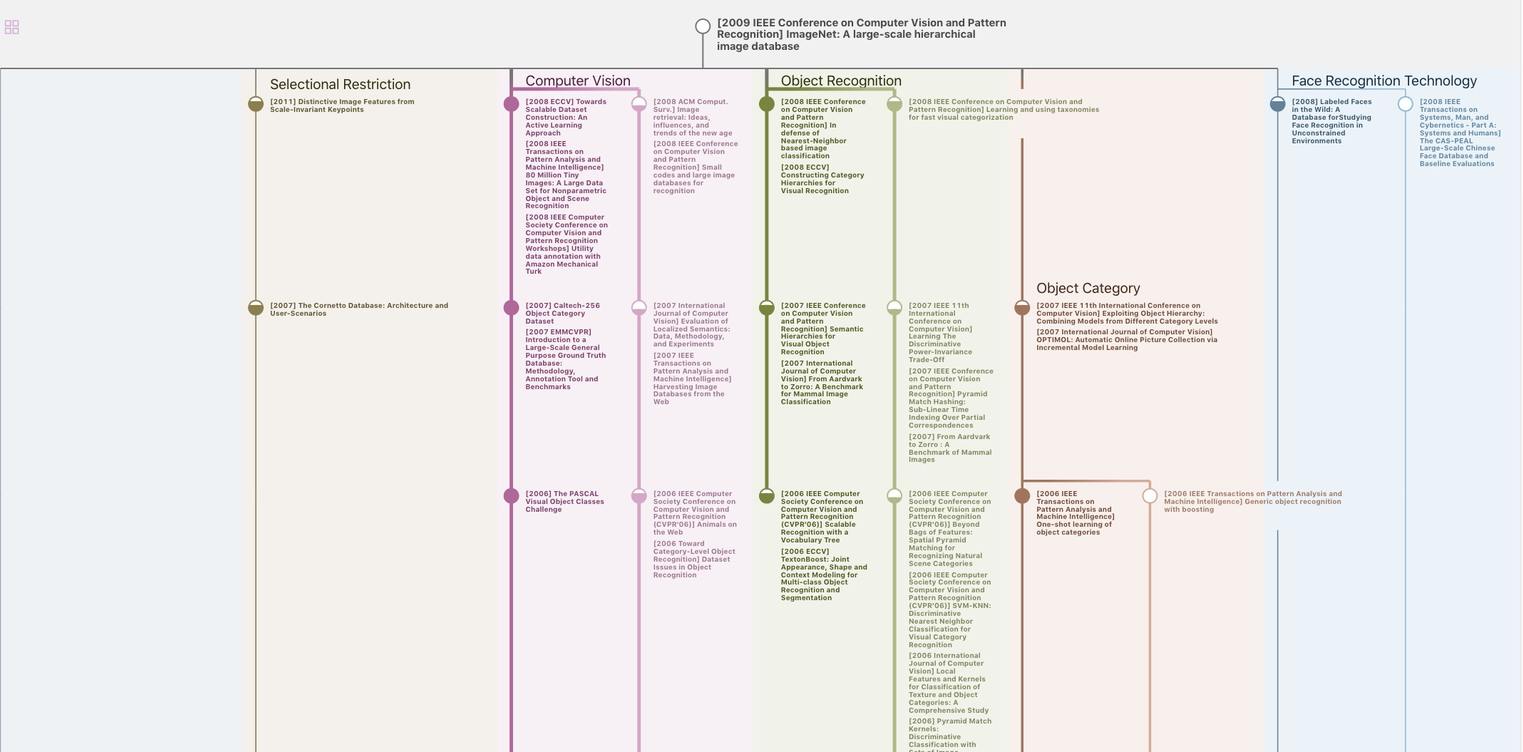
生成溯源树,研究论文发展脉络
Chat Paper
正在生成论文摘要