DeepWiPHY: Deep Learning-Based Receiver Design and Dataset for IEEE 802.11ax Systems
IEEE Transactions on Wireless Communications(2021)
摘要
In this work, we develop DeepWiPHY, a deep learning-based architecture to replace the channel estimation, common phase error (CPE) correction, sampling rate offset (SRO) correction, and equalization modules of IEEE 802.11ax based orthogonal frequency division multiplexing (OFDM) receivers. We first train DeepWiPHY with a synthetic dataset, which is generated using representative indoor channel models and includes typical radio frequency (RF) impairments that are the source of nonlinearity in wireless systems. To further train and evaluate DeepWiPHY with real-world data, we develop a passive sniffing-based data collection testbed composed of Universal Software Radio Peripherals (USRPs) and commercially available IEEE 802.11ax products. The comprehensive evaluation of DeepWiPHY with synthetic and real-world datasets (110 million synthetic OFDM symbols and 14 million real-world OFDM symbols) confirms that, even without fine-tuning the neural network's architecture parameters, DeepWiPHY achieves comparable performance to or outperforms the conventional WLAN receivers, in terms of both bit error rate (BER) and packet error rate (PER), under a wide range of channel models, signal-to-noise (SNR) levels, and modulation schemes.
更多查看译文
关键词
Deep learning,IEEE 802.11ax,Wi-Fi 6,OFDM,channel estimation,hardware impairment compensation,dataset generation,experimental evaluation
AI 理解论文
溯源树
样例
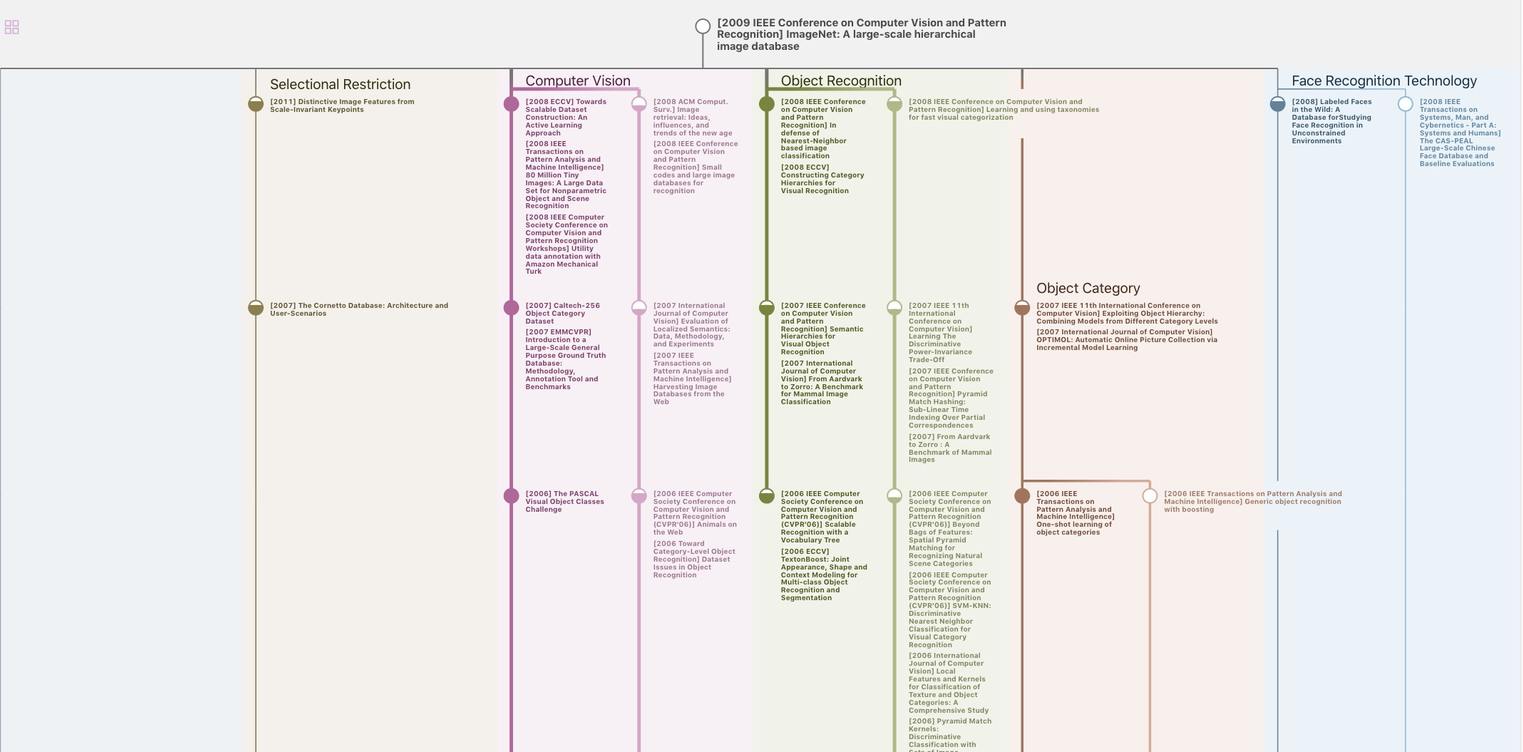
生成溯源树,研究论文发展脉络
Chat Paper
正在生成论文摘要