ST-GRAT: A Novel Spatio-temporal Graph Attention Networks for Accurately Forecasting Dynamically Changing Road Speed
CIKM '20: The 29th ACM International Conference on Information and Knowledge Management Virtual Event Ireland October, 2020(2020)
摘要
Predicting road traffic speed is a challenging task due to different types of roads, abrupt speed change and spatial dependencies between roads; it requires the modeling of dynamically changing spatial dependencies among roads and temporal patterns over long input sequences. This paper proposes a novel spatio-temporal graph attention (ST-GRAT) that effectively captures the spatio-temporal dynamics in road networks. The novel aspects of our approach mainly include spatial attention, temporal attention, and spatial sentinel vectors. The spatial attention takes the graph structure information (e.g., distance between roads) and dynamically adjusts spatial correlation based on road states. The temporal attention is responsible for capturing traffic speed changes, and the sentinel vectors allow the model to retrieve new features from spatially correlated nodes or preserve existing features. The experimental results show that ST-GRAT outperforms existing models, especially in difficult conditions where traffic speeds rapidly change (e.g., rush hours). We additionally provide a qualitative study to analyze when and where ST-GRAT tended to make accurate predictions during rush-hour times.
更多查看译文
关键词
traffic prediction, graph neural networks, spatial-temporal modeling, attention networks, time-series prediction
AI 理解论文
溯源树
样例
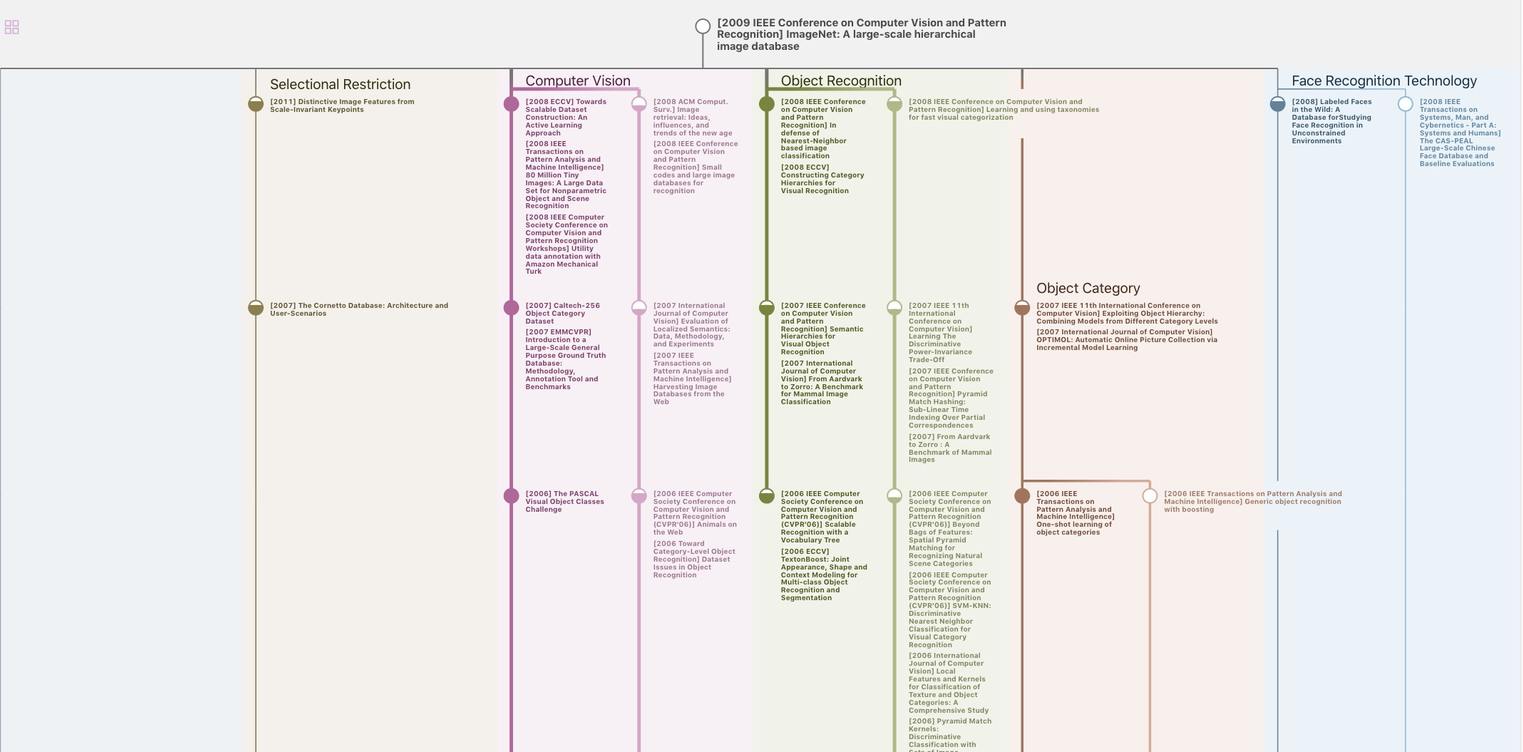
生成溯源树,研究论文发展脉络
Chat Paper
正在生成论文摘要