Compression of Deep Learning Models for NLP
CIKM '20: The 29th ACM International Conference on Information and Knowledge Management Virtual Event Ireland October, 2020(2020)
摘要
In recent years, the fields of NLP and information retrieval have made tremendous progress thanks to deep learning models like RNNs and LSTMs, and Transformer[35] based models like BERT[9]. But these models are humongous in size. Real world applications however demand small model size, low response times and low computational power wattage. We will discuss six different types of methods (pruning, quantization, knowledge distillation, parameter sharing, matrix decomposition, and other Transformer based methods) for compression of such models to enable their deployment in real industry NLP projects. Given the critical need of building applications with efficient and small models, and the large amount of recently published work in this area, we believe that this tutorial is very timely. We will organize related work done by the 'deep learning for NLP' community in the past few years and present it as a coherent story.
更多查看译文
AI 理解论文
溯源树
样例
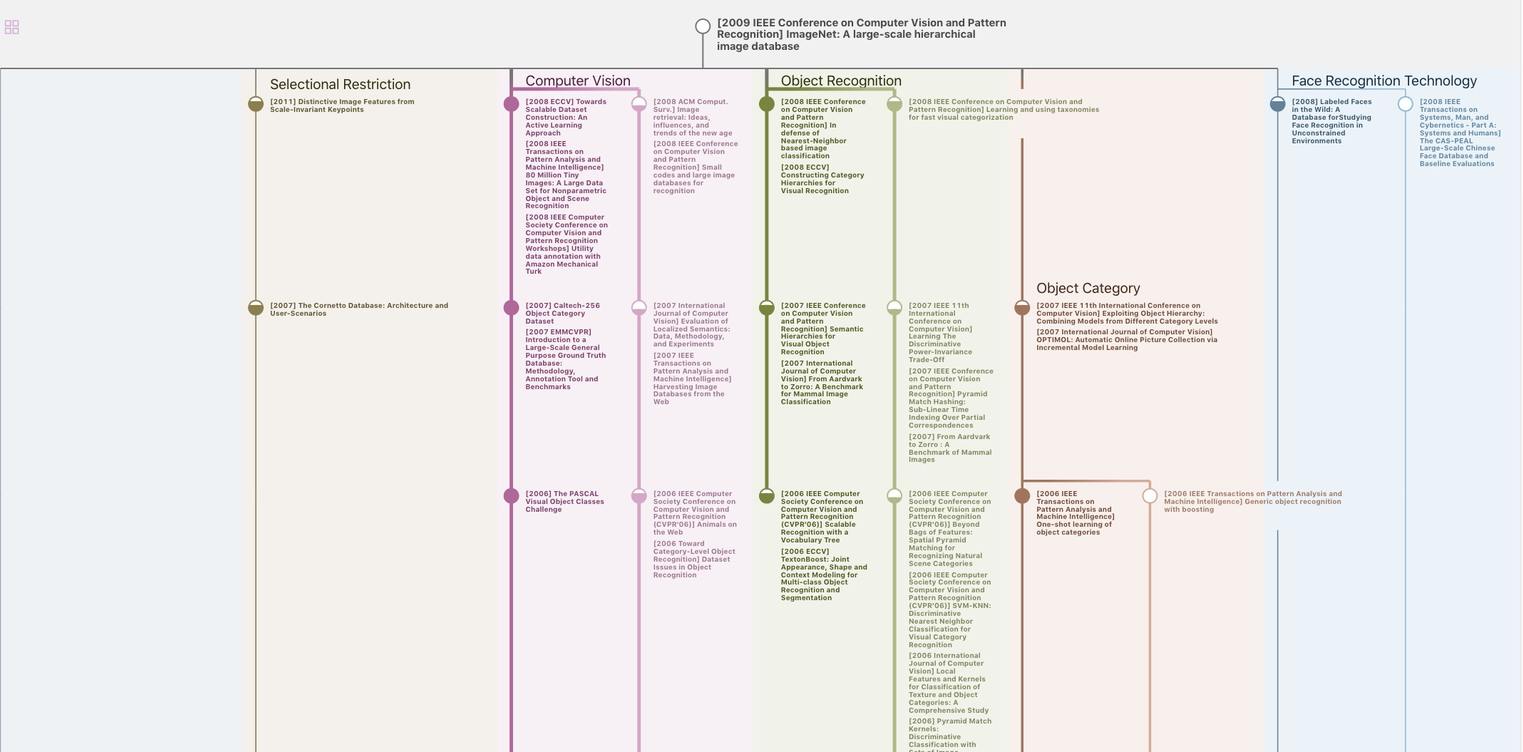
生成溯源树,研究论文发展脉络
Chat Paper
正在生成论文摘要