Semi-Supervised Max-Sum Clustering
CIKM '20: The 29th ACM International Conference on Information and Knowledge Management Virtual Event Ireland October, 2020(2020)
摘要
We study max-sum clustering in a semi-supervised setting. Our objective function maximizes the pairwise within-cluster similarity with respect to some null hypothesis regarding the similarity. This is a natural objective that does not require any additional parameters, and is a generalization of the well-known modularity objective function. We show that for such an objective function in a semi-supervised setting we can compute an additive approximation of the optimal solution in the general case, and a constant-factor approximation when the optimal objective value is large. The supervision that we consider is in the form of cluster assignment queries and same-cluster queries; we also study the setting where the query responses are noisy. Our algorithm also generalizes to the min-sum objective function, for which we can achieve similar performance guarantees. We present computational experiments to show that our framework is effective for clustering text data - we are able to find clusterings that are close to the queried clustering and have a good objective value.
更多查看译文
关键词
semi-supervised learning, clustering, max-sum clustering, min-sum clustering, modularity, text clustering
AI 理解论文
溯源树
样例
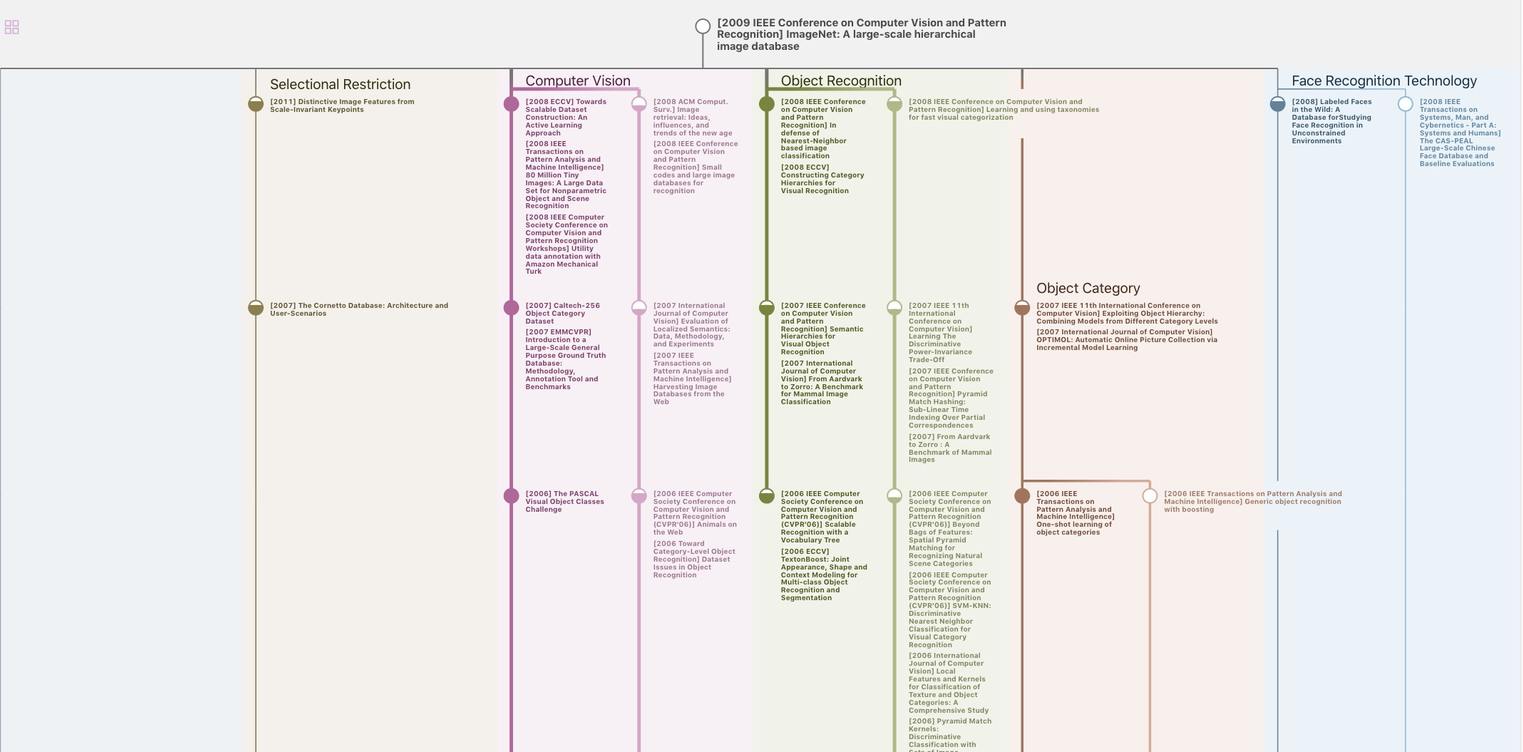
生成溯源树,研究论文发展脉络
Chat Paper
正在生成论文摘要