Convolutional Neural Network For Multiple Particle Identification In The Microboone Liquid Argon Time Projection Chamber
PHYSICAL REVIEW D(2021)
摘要
We present the multiple particle identification (MPID) network, a convolutional neural network for multiple object classification, developed by MicroBooNE. MPID provides the probabilities that an interaction includes an e(-), gamma, mu(-), pi(+/-), and protons in a liquid argon time projection chamber single readout plane. The network extends the single particle identification network previously developed by MicroBooNE [Convolutional neural networks applied to neutrino events in a liquid argon time projection chamber, R. Acciarri et al. J. Instrum. 12, P03011 (2017)]. MPID takes as input an image either cropped around a reconstructed interaction vertex or containing only activity connected to a reconstructed vertex, therefore relieving the tool from inefficiencies in vertex finding and particle clustering. The network serves as an important component in MicroBooNE's deep-learning-based.e search analysis. In this paper, we present the network's design, training, and performance on simulation and data from the MicroBooNE detector.
更多查看译文
AI 理解论文
溯源树
样例
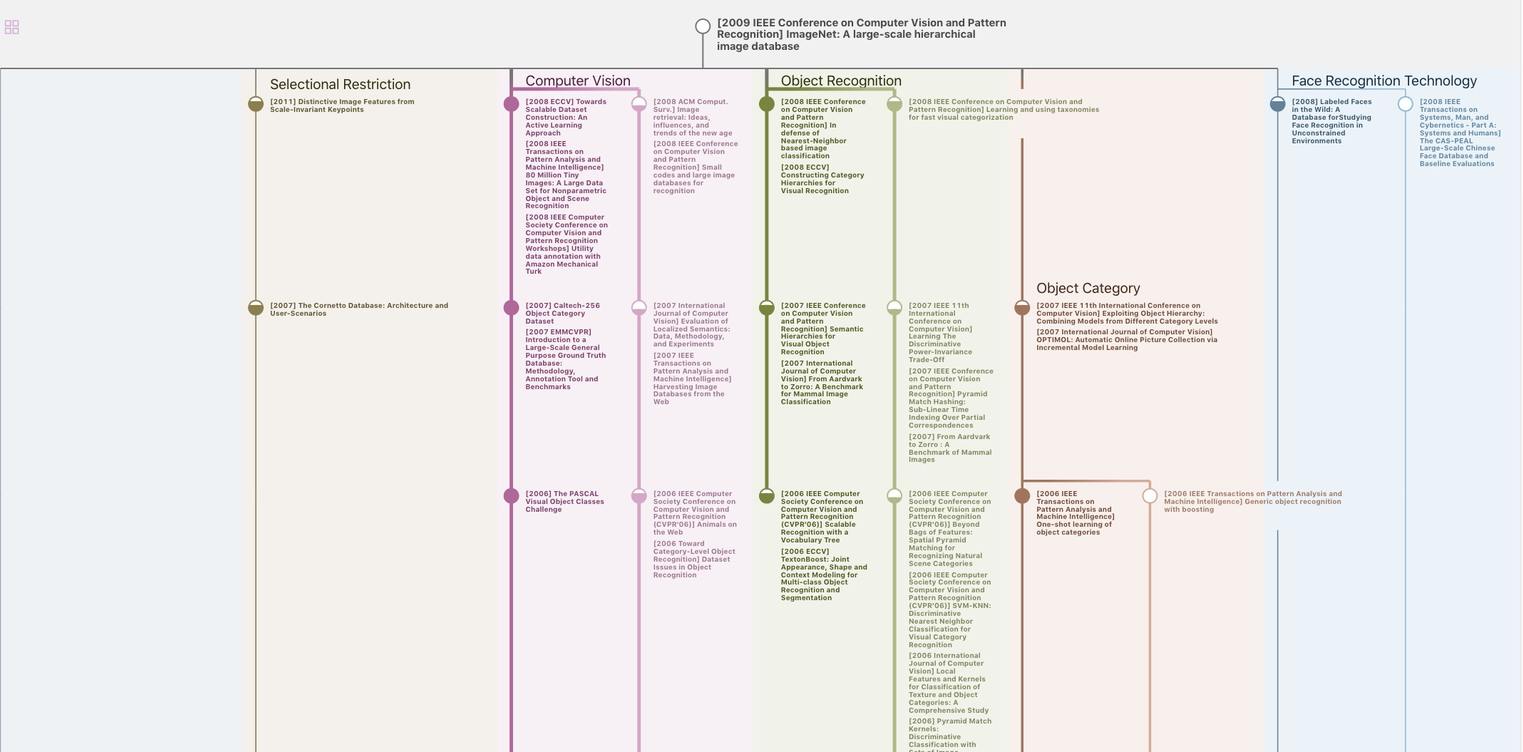
生成溯源树,研究论文发展脉络
Chat Paper
正在生成论文摘要