sDTM: A Supervised Bayesian Deep Topic Model for Text Analytics
Available at SSRN 3612168(2023)
摘要
Topic modeling methods such as latent Dirichlet allocation (LDA) are powerful tools for analyzing massive amounts of textual data. They have been used extensively in information systems (IS) and business discipline research to identify latent topics for data exploration and as a feature engineering mechanism to derive new variables for analyses. However, existing topic modeling approaches are mostly unsupervised and only leverage textual data, while ignoring additional useful metadata often associated with text, such as star ratings in customer reviews or categories of posts in online forums. As a result, the identified topics and variables derived based on the learned topic model may not be accurate, which could lead to incorrect estimations that affect subsequent empirical analysis and to inferior performance on predictive tasks. In this study, we propose a novel supervised deep topic modeling approach called sDTM, which combines a neural variational autoencoder model and a recurrent neural network. sDTM leverages the auxiliary data associated with text to enhance the topic modeling capability. We conduct empirical case studies and predictive analytics on an online consumer review data set and an online knowledge community data set. Experimental results show that in comparison with benchmark methods, sDTM can enhance both the empirical estimation and predictive performance. sDTM makes methodological contributions to the IS literature and has direct relevance for research using text analytics.
更多查看译文
关键词
supervised topic modeling,Bayesian variational inference,deep learning,text analysis
AI 理解论文
溯源树
样例
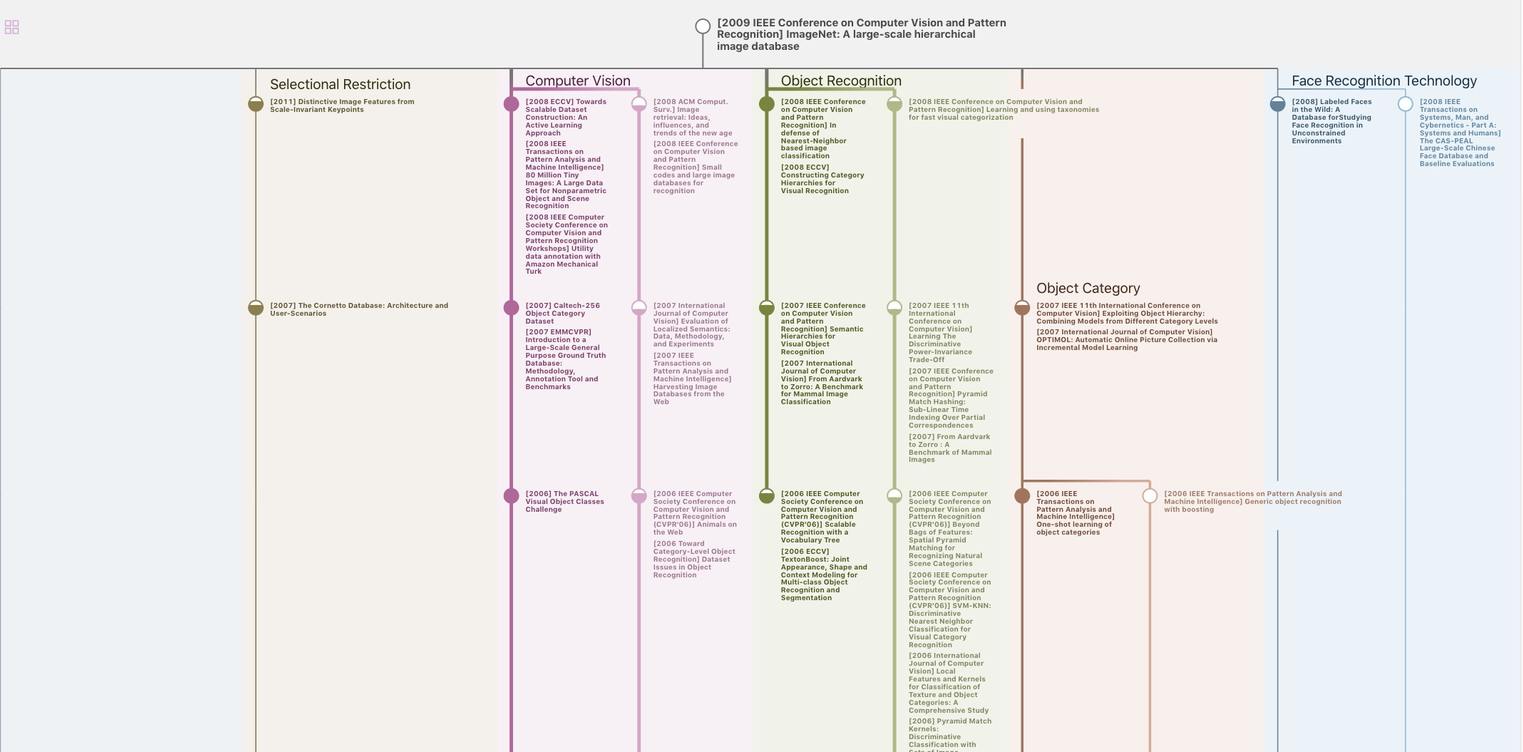
生成溯源树,研究论文发展脉络
Chat Paper
正在生成论文摘要