Ensembled CTR Prediction via Knowledge Distillation
CIKM '20: The 29th ACM International Conference on Information and Knowledge Management Virtual Event Ireland October, 2020(2020)
摘要
Recently, deep learning-based models have been widely studied for click-through rate (CTR) prediction and lead to improved prediction accuracy in many industrial applications. However, current research focuses primarily on building complex network architectures to better capture sophisticated feature interactions and dynamic user behaviors. The increased model complexity may slow down online inference and hinder its adoption in real-time applications. Instead, our work targets at a new model training strategy based on knowledge distillation (KD). KD is a teacher-student learning framework to transfer knowledge learned from a teacher model to a student model. The KD strategy not only allows us to simplify the student model as a vanilla DNN model but also achieves significant accuracy improvements over the state-of-the-art teacher models. The benefits thus motivate us to further explore the use of a powerful ensemble of teachers for more accurate student model training. We also propose some novel techniques to facilitate ensembled CTR prediction, including teacher gating and early stopping by distillation loss. We conduct comprehensive experiments against 12 existing models and across three industrial datasets. Both offline and online A/B testing results show the effectiveness of our KD-based training strategy.
更多查看译文
关键词
CTR prediction, recommender systems, online advertising, knowledge distillation, model ensemble
AI 理解论文
溯源树
样例
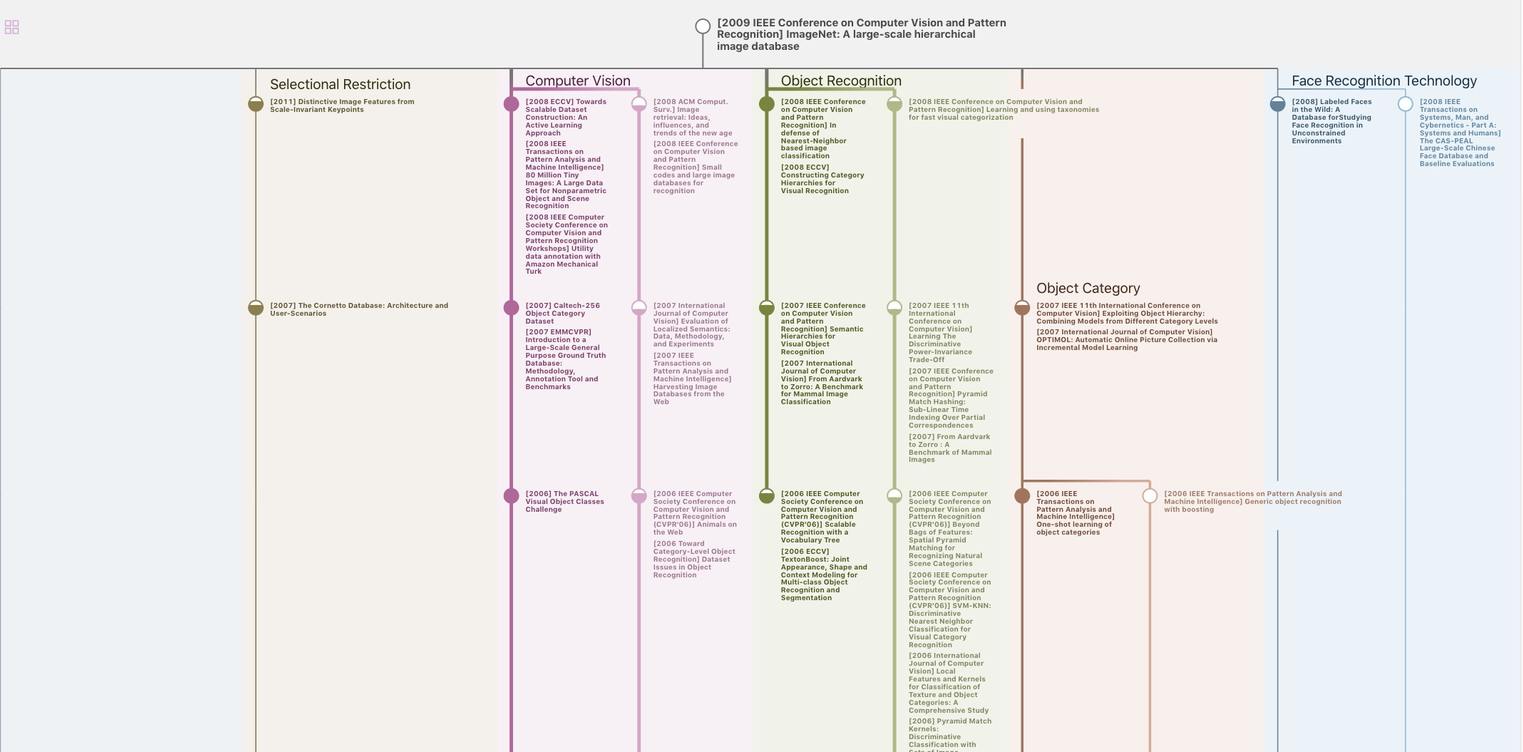
生成溯源树,研究论文发展脉络
Chat Paper
正在生成论文摘要