"Keep it Simple, Lazy" - MetaLazy: A New MetaStrategy for Text Classification
CIKM '20: The 29th ACM International Conference on Information and Knowledge Management Virtual Event Ireland October, 2020(2020)
摘要
Recent advances in text-related tasks on theWeb, such as text (topic) classification and sentiment analysis, have been made possible by exploiting mostly the "rule of more": more data (massive amounts) more computing power, more complex solutions. We propose a shift in the paradigm to do "more with less" by focusing, at maximum extent, just on the task at hand (e.g., classify a single test instance). Accordingly, we propose MetaLazy, a new supervised lazy text classification meta-strategy that greatly extends the scope of lazy solutions. Lazy classifiers postpone the creation of a classification model until a given test instance for decision making is given. MetaLazy exploits new ideas and solutions, which have in common their lazy nature, producing altogether a solution for text classification, which is simpler, more efficient, and less data demanding than new alternatives. It extends and evolves the lazy creation of the model for the test instance by allowing: (i) to dynamically choose the best classifier for the task; (ii) the exploration of distances in the neighborhood of the test document when learning a classification model, thus diminishing the importance of irrelevant training instances; and (iii) a better representational space for training and test documents by augmenting them, in a lazy fashion, with new co-occurrence based features considering just those observed in the specific test instance. In a sizeable experimental evaluation, considering topics and sentiment analysis datasets and nine baselines, we show that our MetaLazy instantiations are among the top performers in most situations, even when compared to state-of-the-art deep learning classifiers such as Deep Network Transformer Architectures.
更多查看译文
关键词
machine learning, text classification, lazy learning
AI 理解论文
溯源树
样例
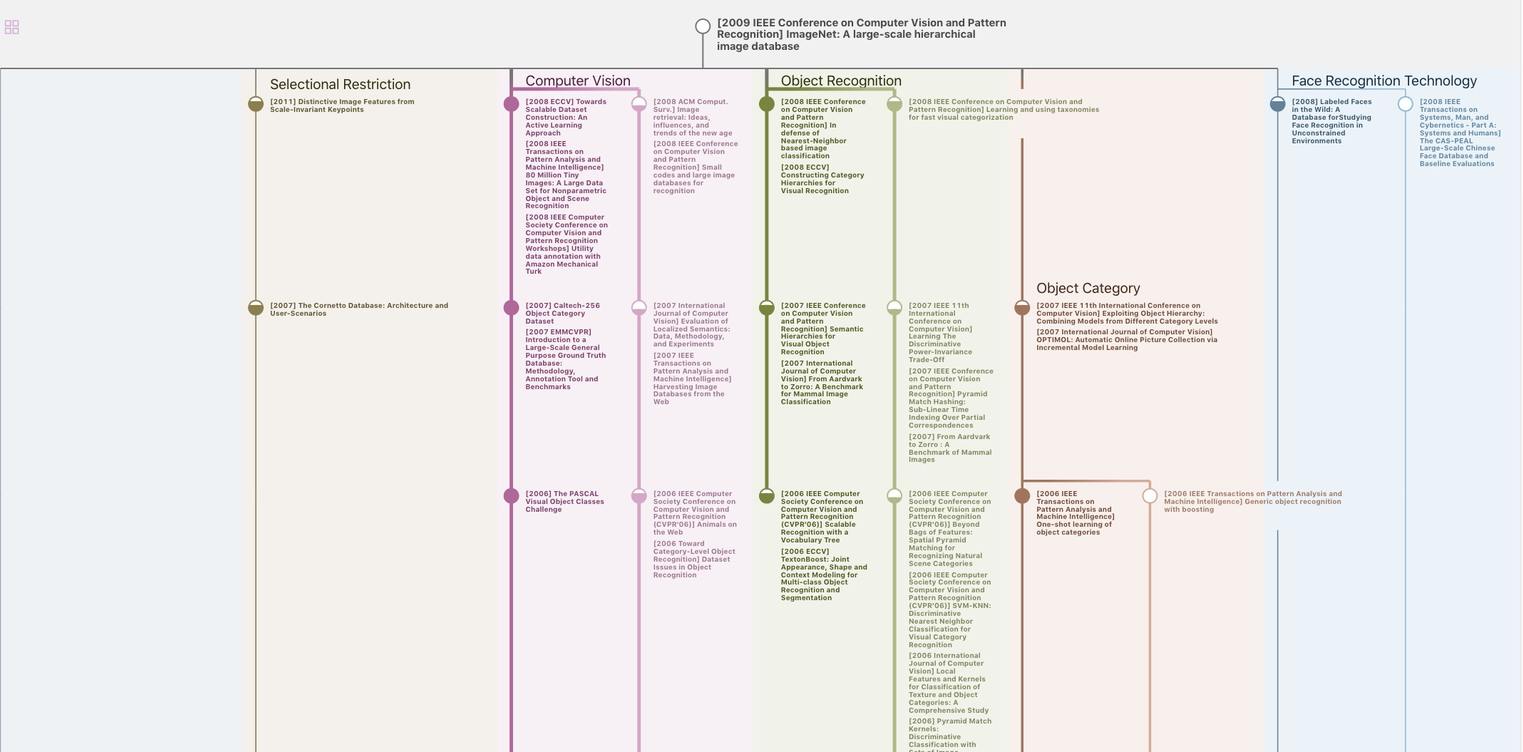
生成溯源树,研究论文发展脉络
Chat Paper
正在生成论文摘要