The Ridgelet Prior: A Covariance Function Approach To Prior Specification For Bayesian Neural Networks
JOURNAL OF MACHINE LEARNING RESEARCH(2021)
摘要
Bayesian neural networks attempt to combine the strong predictive performance of neural networks with formal quantification of uncertainty associated with the predictive output in the Bayesian framework. However, it remains unclear how to endow the parameters of the network with a prior distribution that is meaningful when lifted into the output space of the network. A possible solution is proposed that enables the user to posit an appropriate Gaussian process covariance function for the task at hand. Our approach constructs a prior distribution for the parameters of the network, called a ridgelet prior, that approximates the posited Gaussian process in the output space of the network. In contrast to existing work on the connection between neural networks and Gaussian processes, our analysis is non-asymptotic, with finite sample-size error bounds provided. This establishes the universality property that a Bayesian neural network can approximate any Gaussian process whose covariance function is sufficiently regular. Our experimental assessment is limited to a proof-of-concept, where we demonstrate that the ridgelet prior can out-perform an unstructured prior on regression problems for which a suitable Gaussian process prior can be provided.
更多查看译文
关键词
Bayesian neural networks, Gaussian processes, prior selection, ridgelet transform, statistical learning theory
AI 理解论文
溯源树
样例
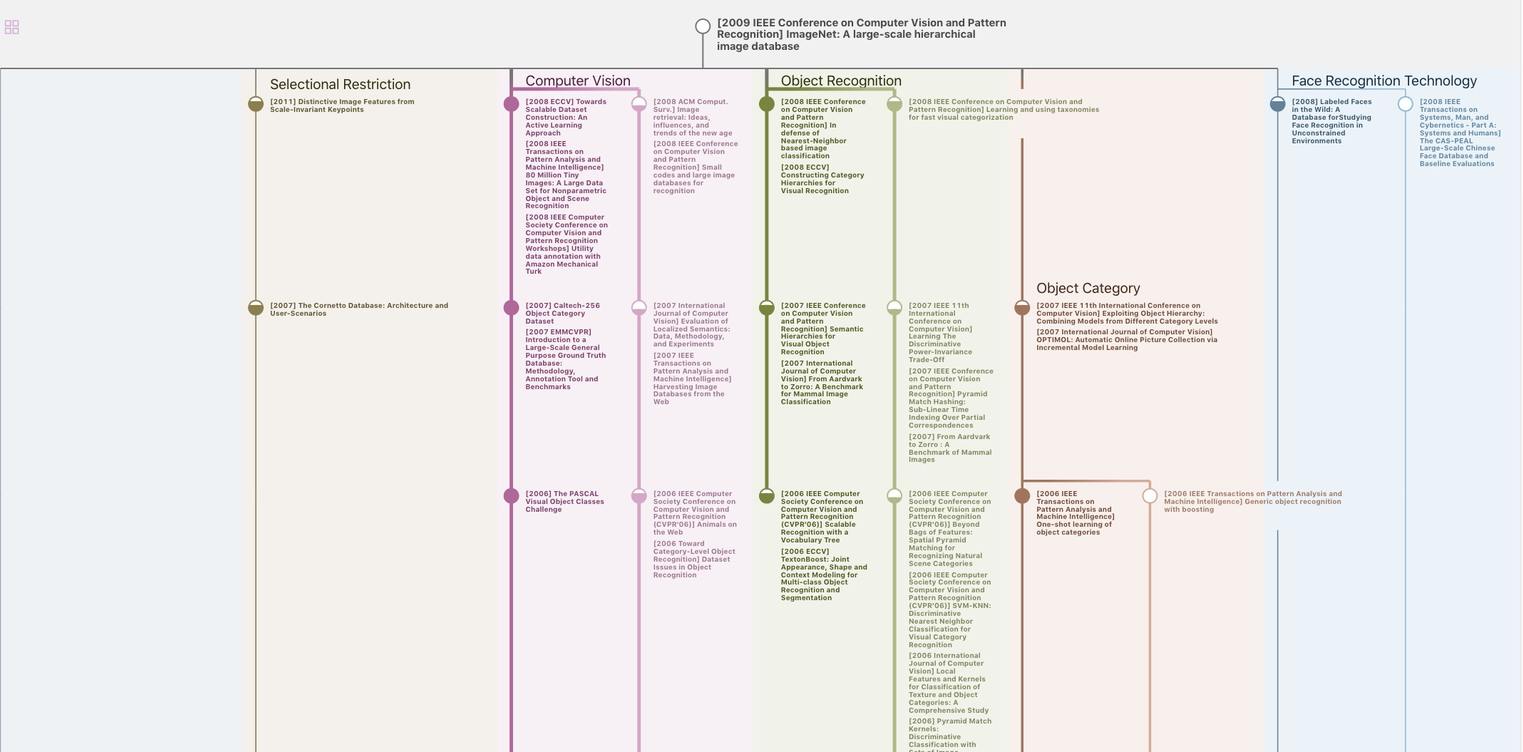
生成溯源树,研究论文发展脉络
Chat Paper
正在生成论文摘要