Automatic Feasibility Study via Data Quality Analysis for ML: A Case-Study on Label Noise
arxiv(2022)
摘要
In our experience of working with domain experts who are using today's AutoML systems, a common problem we encountered is what we call "unrealistic expectations" -- when users are facing a very challenging task with a noisy data acquisition process, while being expected to achieve startlingly high accuracy with machine learning (ML). Many of these are predestined to fail from the beginning. In traditional software engineering, this problem is addressed via a feasibility study, an indispensable step before developing any software system. In this paper, we present Snoopy, with the goal of supporting data scientists and machine learning engineers performing a systematic and theoretically founded feasibility study before building ML applications. We approach this problem by estimating the irreducible error of the underlying task, also known as the Bayes error rate (BER), which stems from data quality issues in datasets used to train or evaluate ML model artifacts. We design a practical Bayes error estimator that is compared against baseline feasibility study candidates on 6 datasets (with additional real and synthetic noise of different levels) in computer vision and natural language processing. Furthermore, by including our systematic feasibility study with additional signals into the iterative label cleaning process, we demonstrate in end-to-end experiments how users are able to save substantial labeling time and monetary efforts.
更多查看译文
关键词
Feasibility Study for ML,Data Quality for ML
AI 理解论文
溯源树
样例
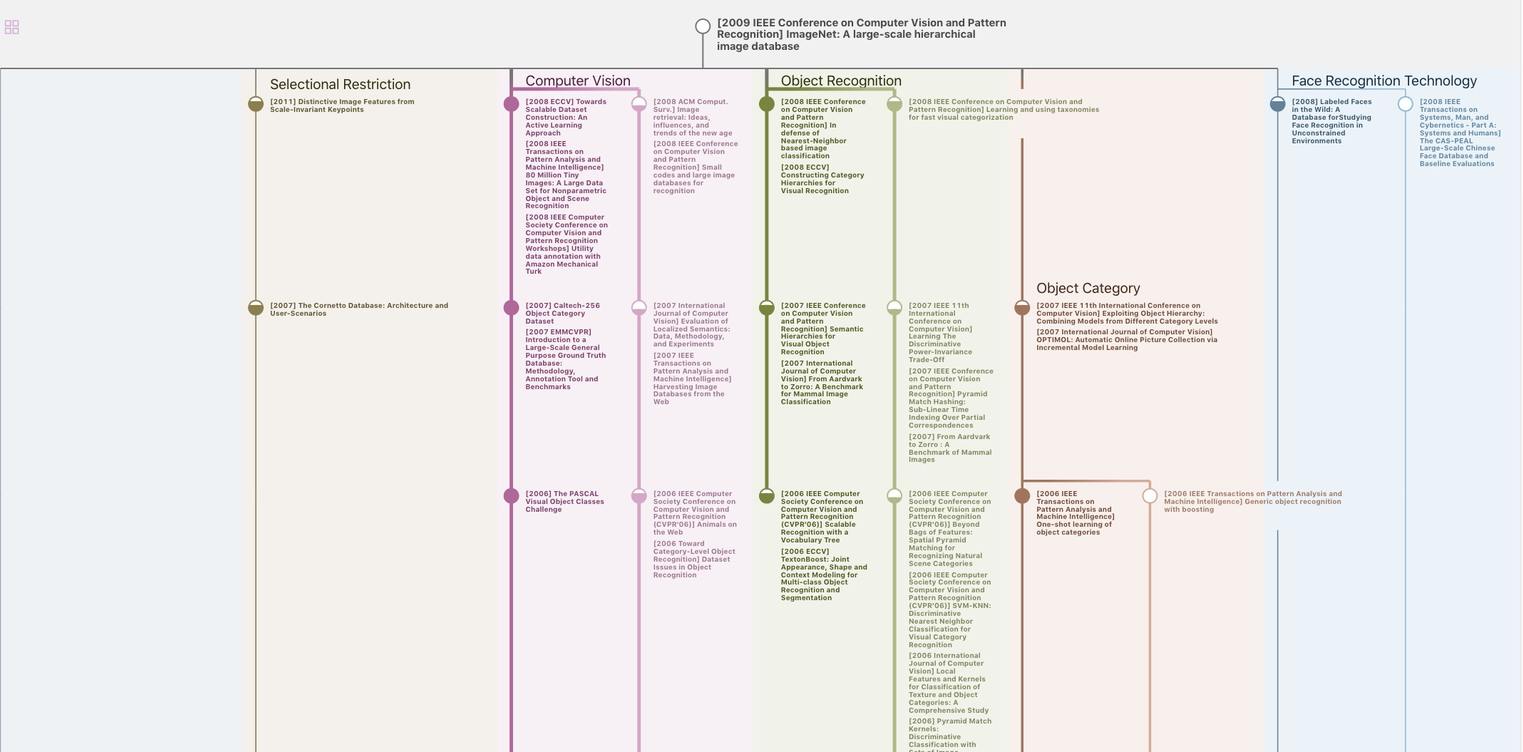
生成溯源树,研究论文发展脉络
Chat Paper
正在生成论文摘要