Neural Networks for Indoor Human Activity Reconstructions
IEEE Sensors Journal(2020)
摘要
Low cost, ubiquitous, tagless, and privacy aware indoor monitoring is essential to many existing or future applications, such as assisted living of elderly persons. We explore how well different types of neural networks in basic configurations can extract location and movement information from noisy experimental data (with both high-pitch and slow drift noise) obtained from capacitive sensors operating in loading mode at ranges much longer than the diagonal of their plates. Through design space exploration, we optimize and analyze the location and trajectory tracking inference performance of multilayer perceptron (MLP), autoregressive feedforward, 1D Convolutional (1D-CNN), and Long-Short Term Memory (LSTM) neural networks on experimental data collected using four capacitive sensors with 16 cm
$\times$
16 cm plates deployed on the boundaries of a 3 m
$\times$
3 m open space in our laboratory. We obtain the minimum error using a 1D-CNN [0.251 m distance Root Mean Square Error (RMSE) and 0.307 m Average Distance Error (ADE)] and the smoothest trajectory inference using an LSTM, albeit with higher localization errors (0.281 m RMSE and 0.326 m ADE). 1D Convolutional and window-based neural networks have best inference accuracy and smoother trajectory reconstruction. LSTMs seem to infer best the person movement dynamics.
更多查看译文
关键词
Capacitive sensors,Artificial neural networks,Monitoring,Trajectory,Senior citizens
AI 理解论文
溯源树
样例
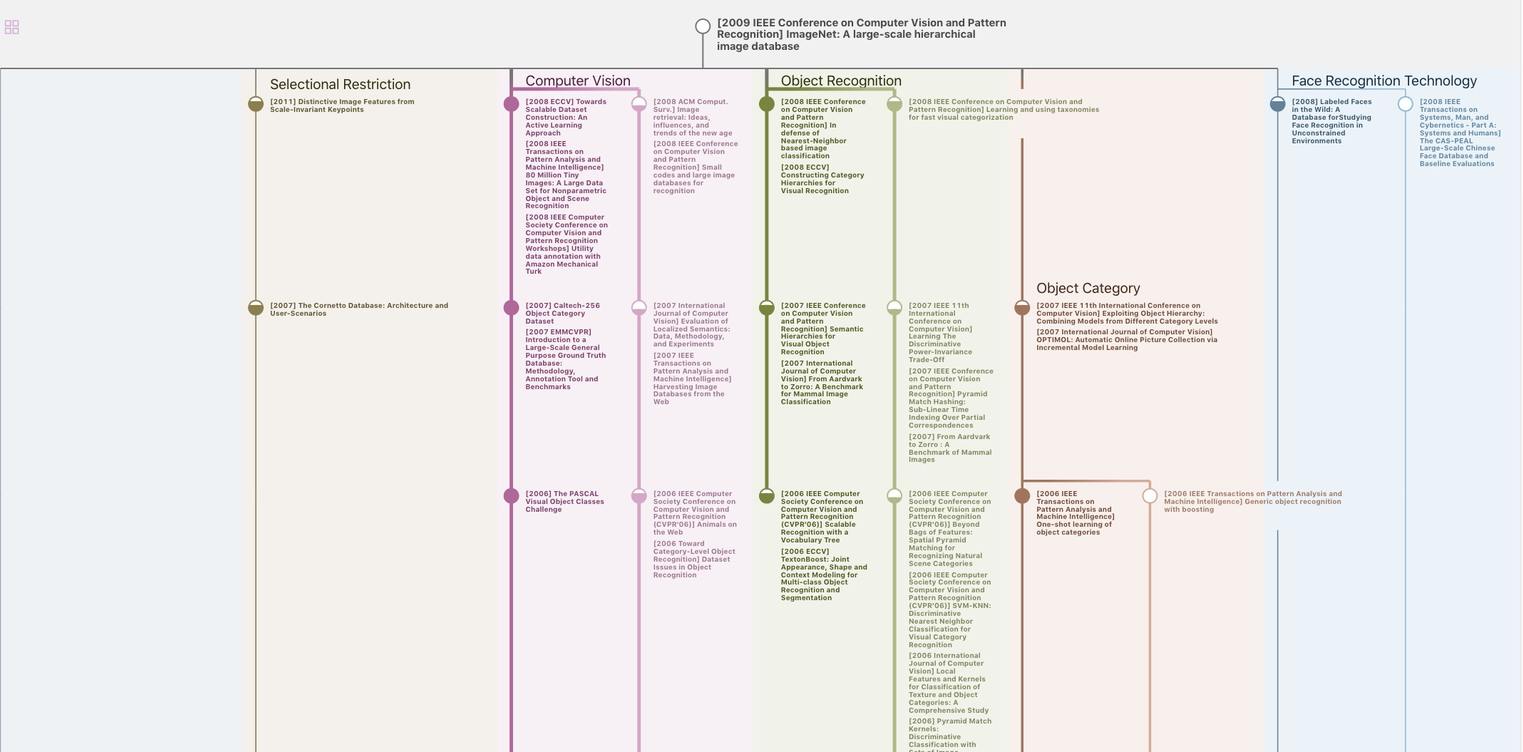
生成溯源树,研究论文发展脉络
Chat Paper
正在生成论文摘要