Ensemble-Bayesian SPC: Multi-mode process monitoring for novelty detection
IISE TRANSACTIONS(2017)
摘要
We propose a monitoring method based on a Bayesian analysis of an ensemble-of-classifiers for Statistical Process Control (SPC) of multi-mode systems. A specific case is considered, in which new modes of operations (new classes), also called "novelties," are identified during the monitoring stage of the system. The proposed Ensemble-Bayesian SPC (EB-SPC) models the known operating modes by categorizing their corresponding observations into data classes that are detected during the training stage, Ensembles of decision trees are trained over replicated subspaces of features, with class-dependent thresholds being computed and used to detect novelties. In contrast with existing monitoring approaches that often focus on a single operating mode as the "in-control" class, the EB-SPC exploits the joint Information of the trained classes and combines the posterior probabilities of various classifiers by using a "mixture-of-experts" approach. Performance evaluation on real datasets from both public repositories and real-world semiconductor datasets shows that the EB-SPC outperforms both conventional multivariate SPC as well as ensemble-of-classifiers methods and has a high potential for novelty detection including the monitoring of multimode systems.
更多查看译文
关键词
TSPC,ensemble,subspaces,bayes,novelty detection
AI 理解论文
溯源树
样例
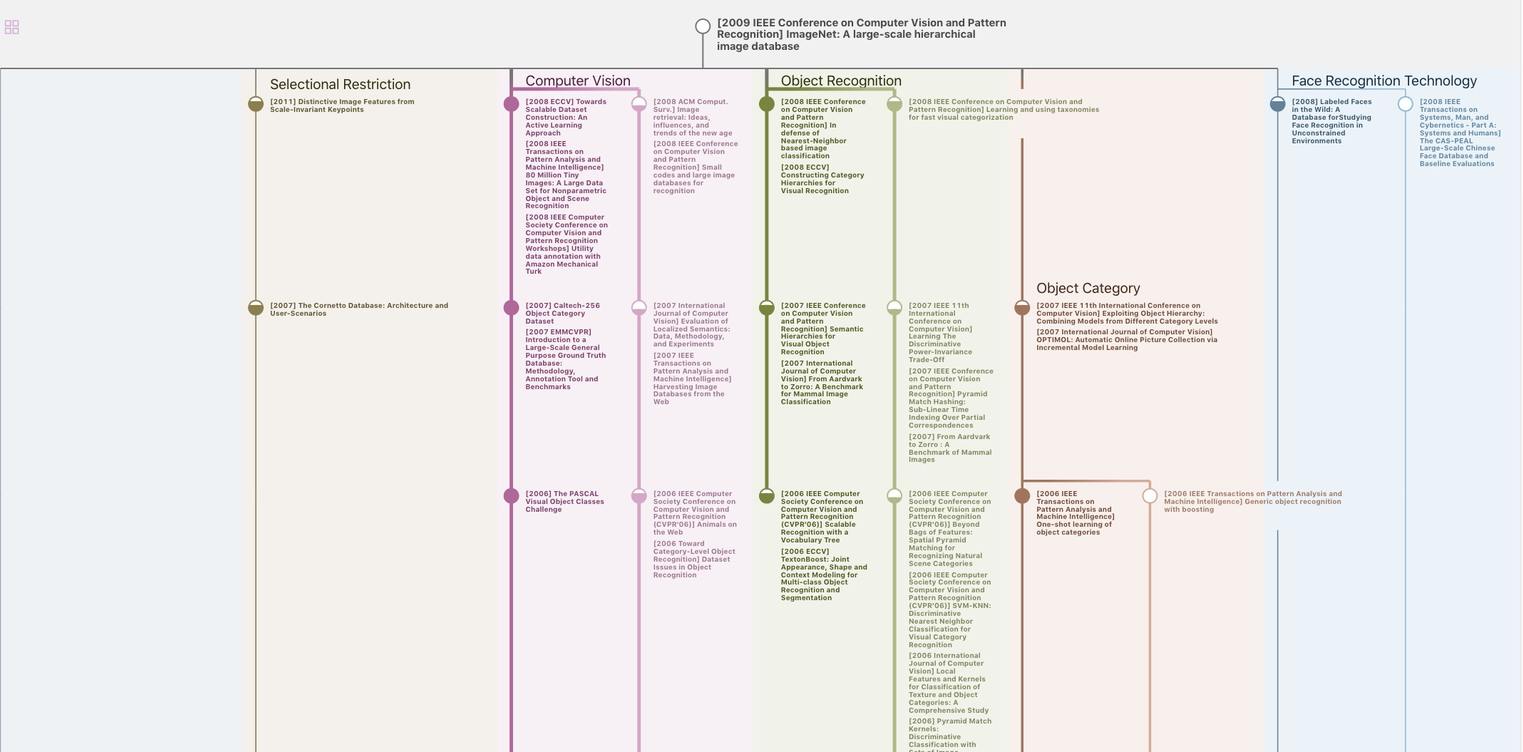
生成溯源树,研究论文发展脉络
Chat Paper
正在生成论文摘要