Deep Convolutional Neural Network-Based Inverse Filtering Approach for Speech De-Reverberation
2020 IEEE 30th International Workshop on Machine Learning for Signal Processing (MLSP)(2020)
摘要
In this paper, we introduce a spectral-domain inverse filtering approach for single-channel speech de-reverberation using deep convolutional neural network (CNN). The main goal is to better handle realistic reverberant conditions where the room impulse response (RIR) filter is longer than the short-time Fourier transform (STFT) analysis window. To this end, we consider the convolutive transfer function (CTF) model for the reverberant speech signal. In the proposed framework, the CNN architecture is trained to directly estimate the inverse filter of the CTF model. Among various choices for the CNN structure, we consider the U-net which consists of a fully-convolutional auto-encoder network with skip-connections. Experimental results show that the proposed method provides better dereverberation performance than the prevalent benchmark algorithms under various reverberation conditions.
更多查看译文
关键词
single-channel speech de-reverberation,inverse filtering,convolutive transfer function,deep convolutional neural network,U-net
AI 理解论文
溯源树
样例
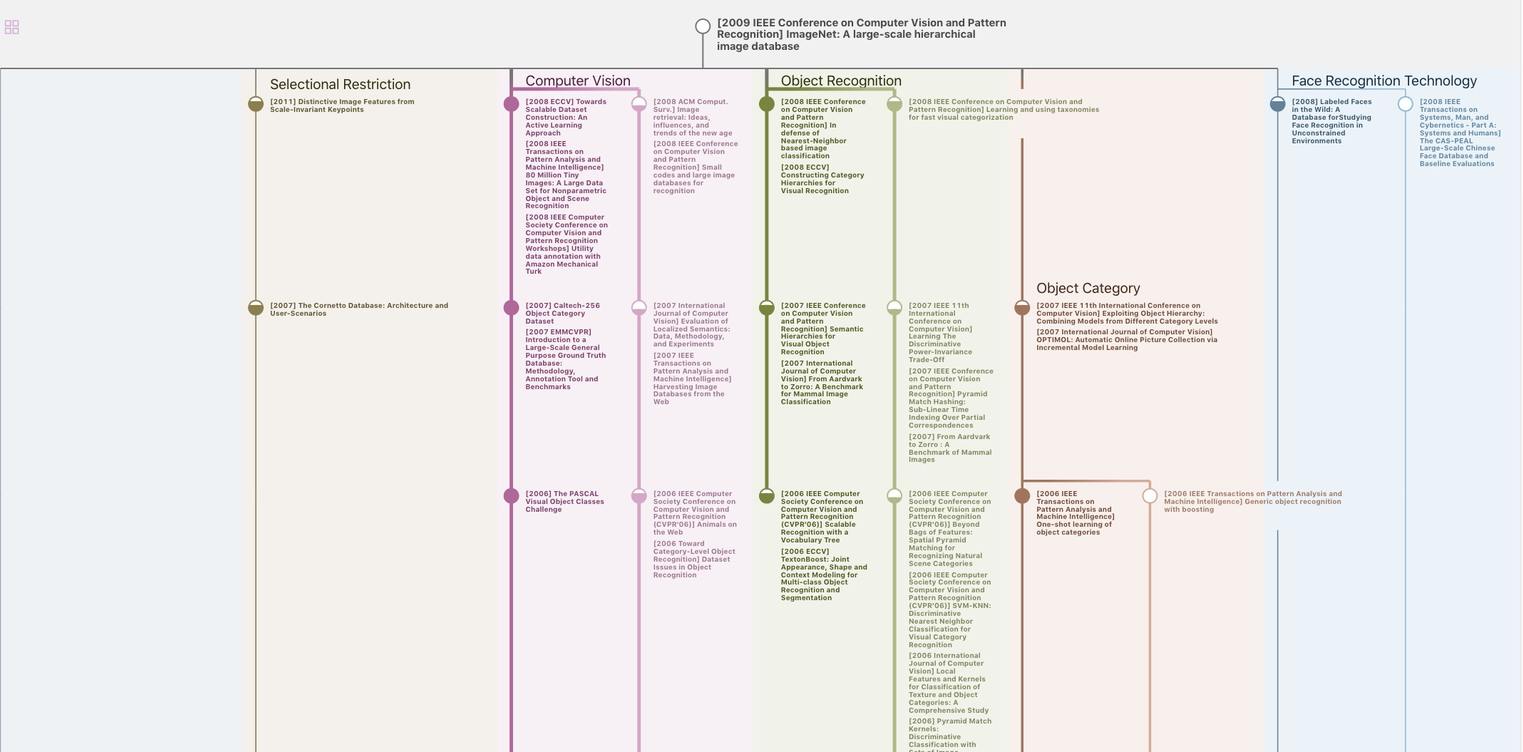
生成溯源树,研究论文发展脉络
Chat Paper
正在生成论文摘要