Sequential Likelihood-Free Inference with Neural Proposal
arxiv(2023)
摘要
Bayesian inference without the likelihood evaluation, or likelihood-free inference , has been a key research topic in simulation studies for gaining quantitatively validated simulation models on real-world datasets. As the likelihood evaluation is inaccessible, previous papers train the amortized neural network to esti-mate the ground-truth posterior for the simulation of interest. Training the network and accumulating the dataset alternatively in a sequential manner could save the total simulation budget by orders of mag-nitude. In the data accumulation phase, the new simulation inputs are chosen within a portion of the total simulation budget to accumulate upon the collected dataset so far. This newly accumulated data degenerates because the set of simulation inputs is hardly mixed, and this degenerated data collection process ruins the posterior inference. This paper introduces a new sampling approach, called Neural Pro-posal (NP), of the simulation input that resolves the biased data collection as it guarantees the i.i.d. sam-pling. The experiments show the improved performance of our sampler, especially for the simulations with multi-modal posteriors. (c) 2023 Elsevier B.V. All rights reserved.
更多查看译文
关键词
Likelihood-Free inference,Simulation parameter calibration,MCMC,Generative models
AI 理解论文
溯源树
样例
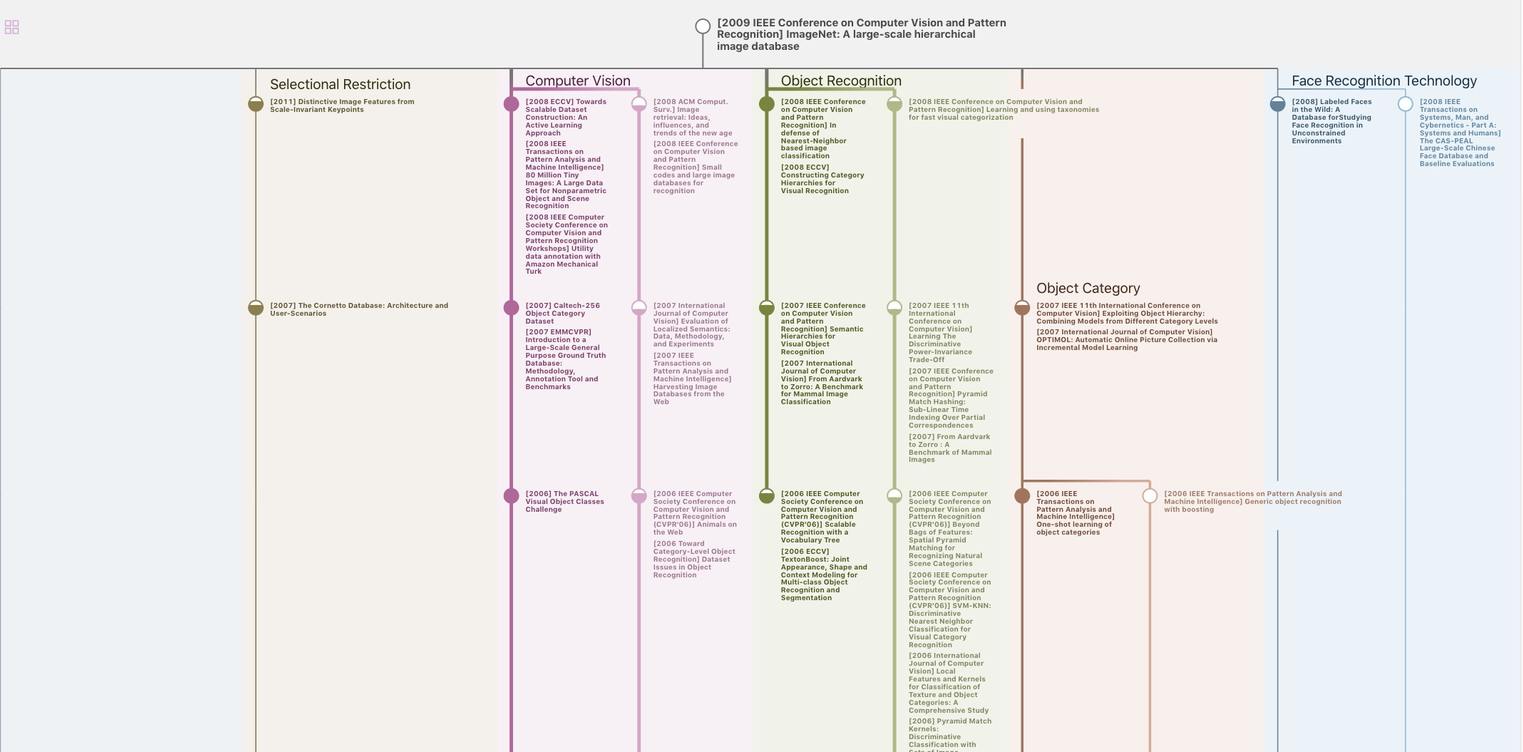
生成溯源树,研究论文发展脉络
Chat Paper
正在生成论文摘要