Self-Supervised Domain Adaptation With Consistency Training
2020 25TH INTERNATIONAL CONFERENCE ON PATTERN RECOGNITION (ICPR)(2020)
摘要
We consider the problem of unsupervised domain adaptation for image classification. To learn target-domain-aware features from the unlabeled data, we create a self-supervised pretext task by augmenting the unlabeled data with a certain type of transformation (specifically, image rotation) and ask the learner to predict the properties of the transformation. However, the obtained feature representation may contain a large amount of irrelevant information with respect to the main task. To provide further guidance, we force the feature representation of the augmented data to be consistent with that of the original data. Intuitively, the consistency introduces additional constraints to representation learning, therefore, the learned representation is more likely to focus on the right information about the main task. Our experimental results validate the proposed method and demonstrate state-of-the-art performance on classical domain adaptation benchmarks. Code is available at https://github.comaiaolong/ss-da-consistency.
更多查看译文
关键词
feature representation,representation learning,self-supervised domain adaptation,consistency training,unsupervised domain adaptation,image classification,target-domain-aware features,self-supervised pretext task,image rotation
AI 理解论文
溯源树
样例
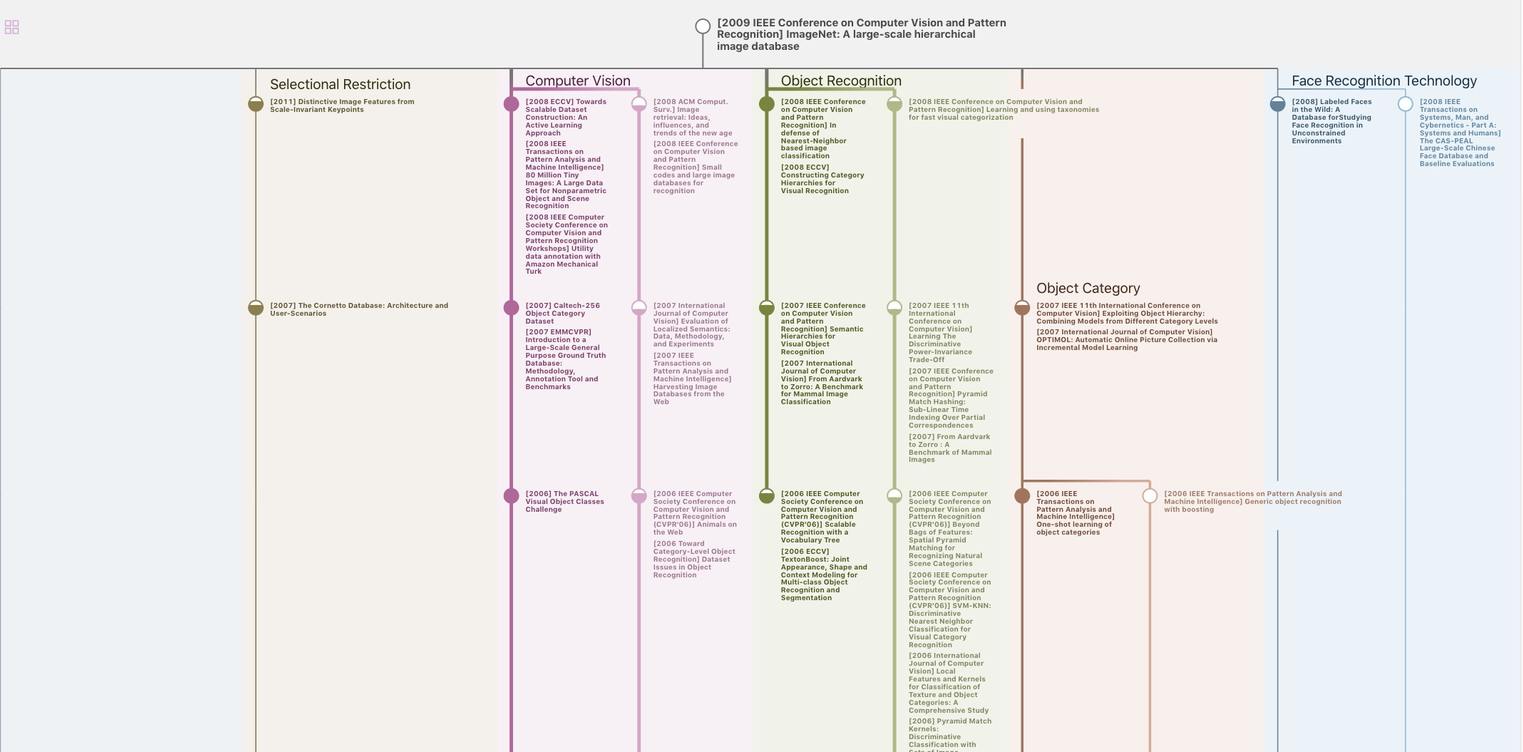
生成溯源树,研究论文发展脉络
Chat Paper
正在生成论文摘要