StarCraft agent strategic training on a large human versus human game replay dataset
2020 15th Conference on Computer Science and Information Systems (FedCSIS)(2020)
摘要
Real-time strategy games are currently very popular as a testbed for AI research and education. StarCraft: Brood War (SC:BW) is one of such games. Recently, a new large, unlabeled human versus human SC:BW game replay dataset called STARDATA was published. This paper aims to prove that the player strategy diversity requirement of the dataset is met, i.e., that the diversity of player strategies in STARDATA replays is of sufficient quality. To this end, we built a competitive SC:BW agent from scratch and trained its strategic decision making process on STARDATA. The results show that in the current state of the competitive environment the agent is capable of keeping a stable rating and a decent win rate over a longer period of time. It also performs better than our other, simple rule-based agent. Therefore, we conclude that the strategy diversity requirement of STARDATA is met.
更多查看译文
AI 理解论文
溯源树
样例
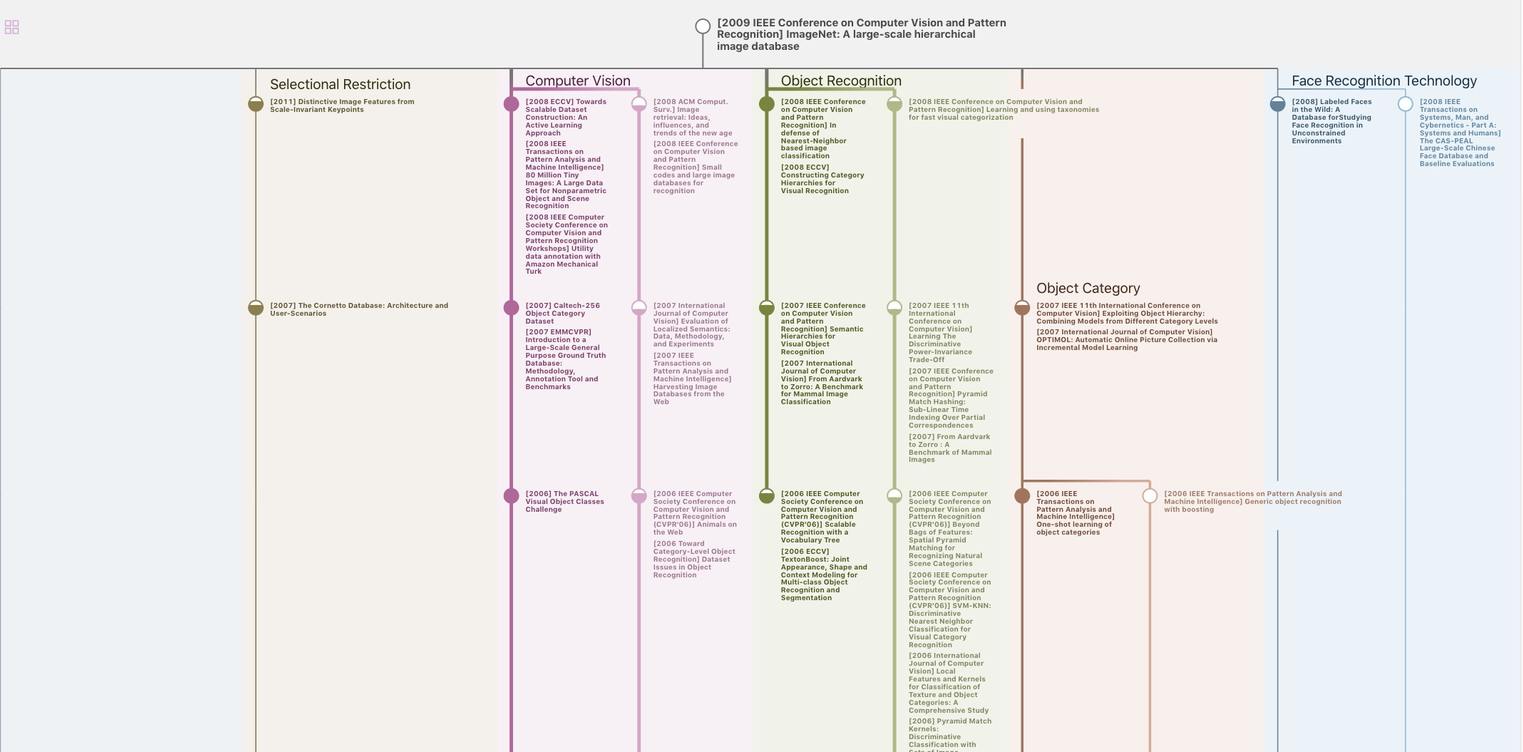
生成溯源树,研究论文发展脉络
Chat Paper
正在生成论文摘要