A Self-Supervised Representation Learning of Sentence Structure for Authorship Attribution
ACM Transactions on Knowledge Discovery from Data(2022)
摘要
AbstractThe syntactic structure of sentences in a document substantially informs about its authorial writing style. Sentence representation learning has been widely explored in recent years and it has been shown that it improves the generalization of different downstream tasks across many domains. Even though utilizing probing methods in several studies suggests that these learned contextual representations implicitly encode some amount of syntax, explicit syntactic information further improves the performance of deep neural models in the domain of authorship attribution. These observations have motivated us to investigate the explicit representation learning of syntactic structure of sentences. In this article, we propose a self-supervised framework for learning structural representations of sentences. The self-supervised network contains two components; a lexical sub-network and a syntactic sub-network which take the sequence of words and their corresponding structural labels as the input, respectively. Due to the n-to-1 mapping of words to their structural labels, each word will be embedded into a vector representation which mainly carries structural information. We evaluate the learned structural representations of sentences using different probing tasks, and subsequently utilize them in the authorship attribution task. Our experimental results indicate that the structural embeddings significantly improve the classification tasks when concatenated with the existing pre-trained word embeddings.
更多查看译文
关键词
Sentence representation, sentence structure, neural network, self-supervised learning, authorship attribution
AI 理解论文
溯源树
样例
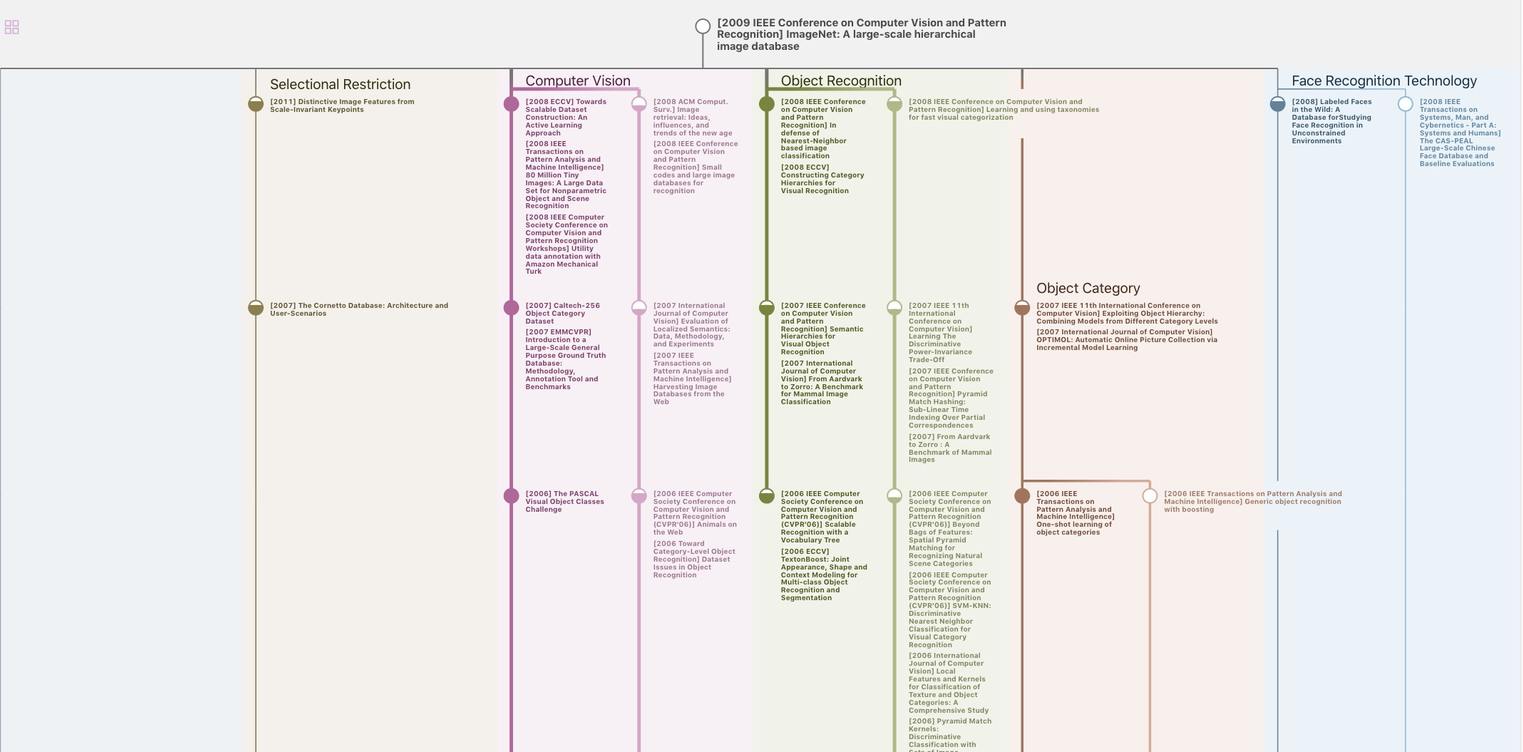
生成溯源树,研究论文发展脉络
Chat Paper
正在生成论文摘要