Training independent subnetworks for robust prediction
ICLR(2020)
摘要
Recent approaches to efficiently ensemble neural networks have shown that strong robustness and uncertainty performance can be achieved with a negligible gain in parameters over the original network. However, these methods still require multiple forward passes for prediction, leading to a significant computational cost. In this work, we show a surprising result: the benefits of using multiple predictions can be achieved `for free' under a single model's forward pass. In particular, we show that, using a multi-input multi-output (MIMO) configuration, one can utilize a single model's capacity to train multiple subnetworks that independently learn the task at hand. By ensembling the predictions made by the subnetworks, we improve model robustness without increasing compute. We observe a significant improvement in negative log-likelihood, accuracy, and calibration error on CIFAR10, CIFAR100, ImageNet, and their out-of-distribution variants compared to previous methods.
更多查看译文
关键词
independent subnetworks,robust prediction,training
AI 理解论文
溯源树
样例
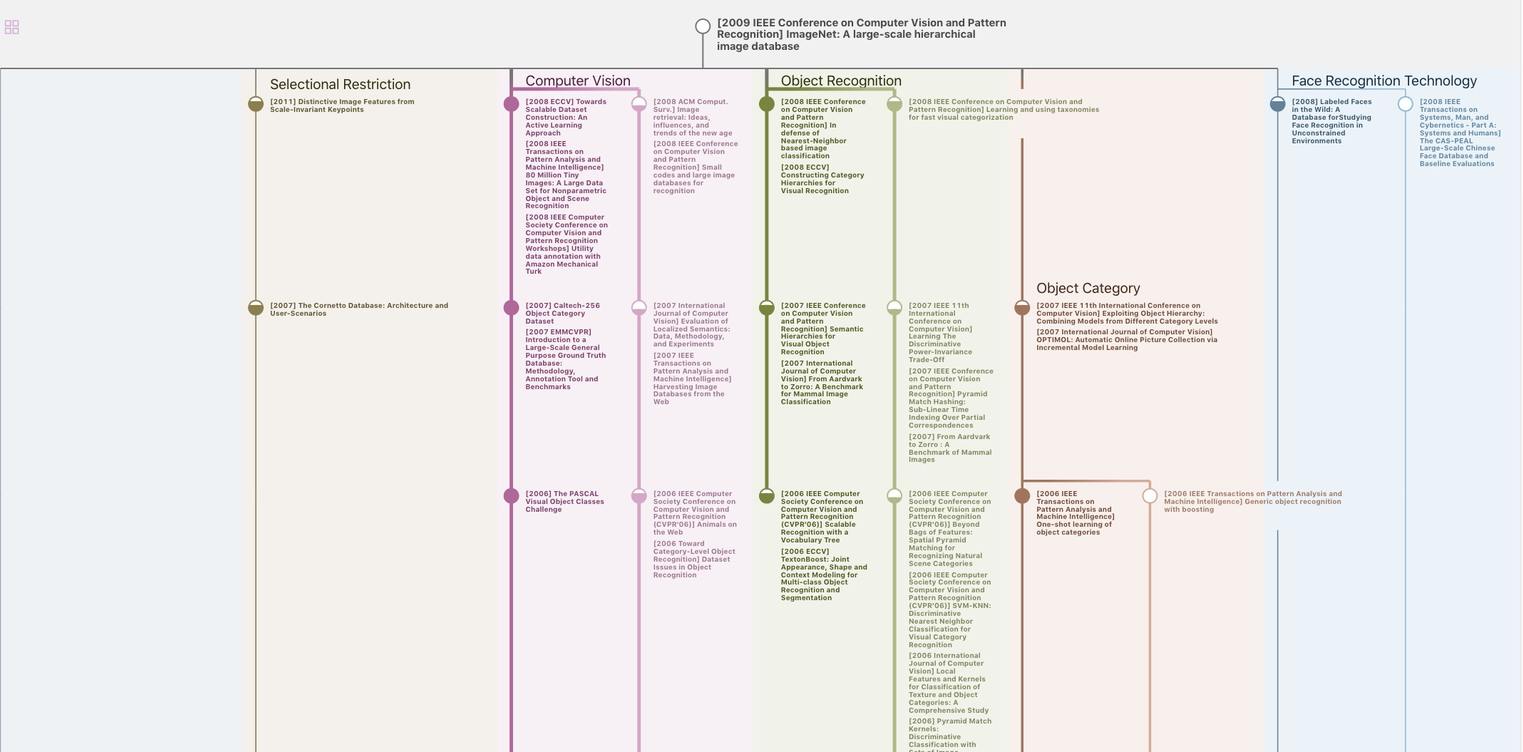
生成溯源树,研究论文发展脉络
Chat Paper
正在生成论文摘要