Multi-Biomarker Prediction Models for Multiple Infection Episodes Following Blunt Trauma
iScience(2020)
摘要
Severe trauma predisposes patients to multiple independent infection episodes (MIIEs), leading to augmented morbidity and mortality. We developed a method to identify increased MIIE risk before clinical signs appear, which is fundamentally different from existing approaches entailing infections' detection after their establishment. Applying machine learning algorithms to genome-wide transcriptome data from 128 adult blunt trauma patients' (42 MIIE cases and 85 non-cases) leukocytes collected ≤48 hr of injury and ≥3 days before any infection, we constructed a 15-transcript and a 26-transcript multi-biomarker panel model with the least absolute shrinkage and selection operator (LASSO) and Elastic Net, respectively, which accurately predicted MIIE (Area Under Receiver Operating Characteristics Curve [AUROC] [95% confidence intervals, CI]: 0.90 [0.84–0.96] and 0.92 [0.86–0.96]) and significantly outperformed clinical models. Gene Ontology and network analyses found various pathways to be relevant. External validation found our model to be generalizable. Our unique precision medicine approach can be applied to a wide range of patient populations and outcomes.
更多查看译文
关键词
Artificial Intelligence,Trauma,Virology
AI 理解论文
溯源树
样例
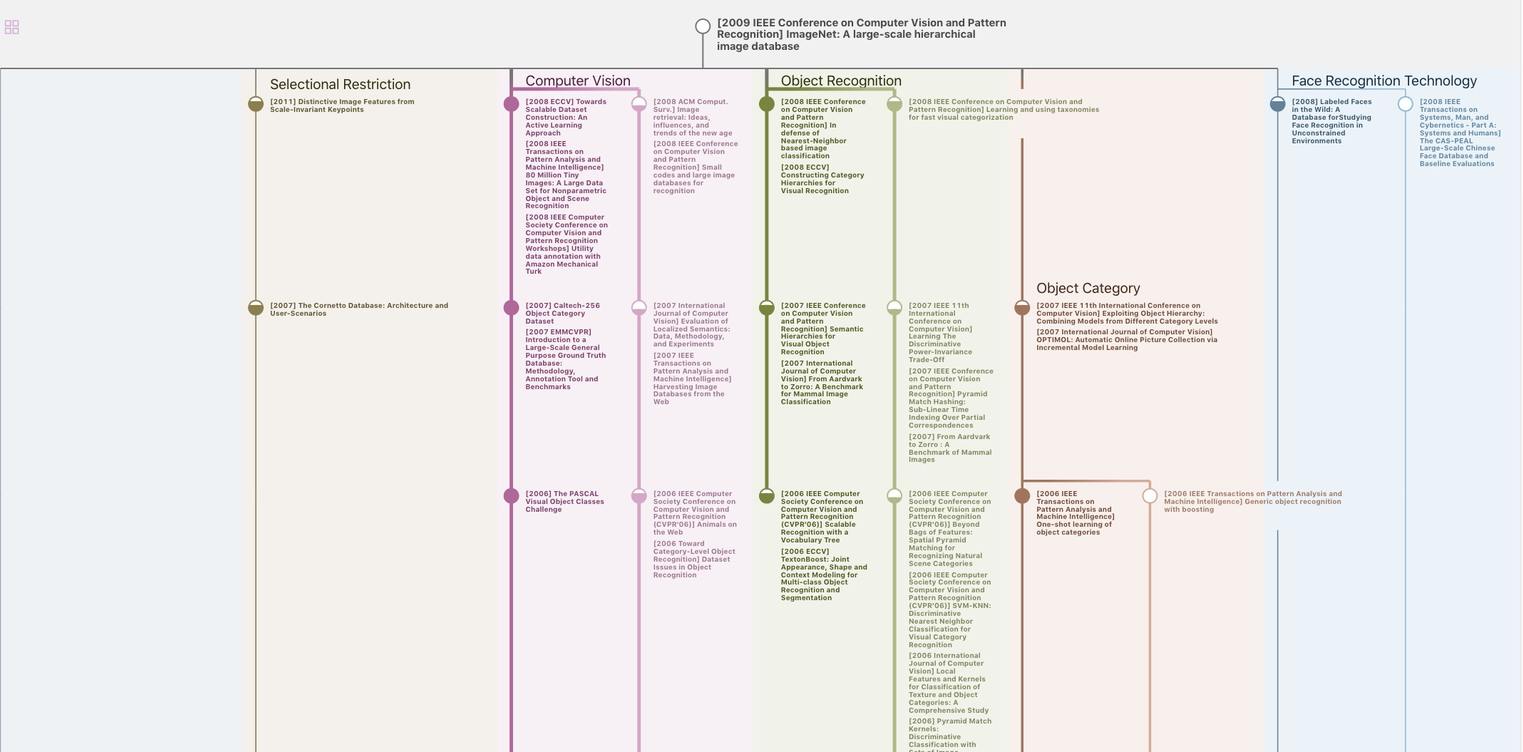
生成溯源树,研究论文发展脉络
Chat Paper
正在生成论文摘要