Bridging the Gap between Vision and Language Domains for Improved Image Captioning
MM '20: The 28th ACM International Conference on Multimedia Seattle WA USA October, 2020(2020)
摘要
Image captioning has attracted extensive research interests in recent years. Due to the great disparities between vision and language, an important goal of image captioning is to link the information in visual domain to textual domain. However, many approaches conduct this process only in the decoder, making it hard to understand the images and generate captions effectively. In this paper, we propose to bridge the gap between the vision and language domains in the encoder, by enriching visual information with textual concepts, to achieve deep image understandings. To this end, we propose to explore the textual-enriched image features. Specifically, we introduce two modules, namely Textual Distilling Module and Textual Association Module. The former distills relevant textual concepts from image features, while the latter further associates extracted concepts according to their semantics. In this manner, we acquire textual-enriched image features, which provide clear textual representations of image under no explicit supervision. The proposed approach can be used as a plugin and easily embedded into a wide range of existing image captioning systems. We conduct the extensive experiments on two benchmark image captioning datasets, i.e., MSCOCO and Flickr30k. The experimental results and analysis show that, by incorporating the proposed approach, all baseline models receive consistent improvements over all metrics, with the most significant improvement up to 10% and 9%, in terms of the task-specific metrics CIDEr and SPICE, respectively. The results demonstrate that our approach is effective and generalizes well to a wide range of models for image captioning.
更多查看译文
关键词
Image Captioning, Image Representations, Textual Concepts, Attention Mechanism
AI 理解论文
溯源树
样例
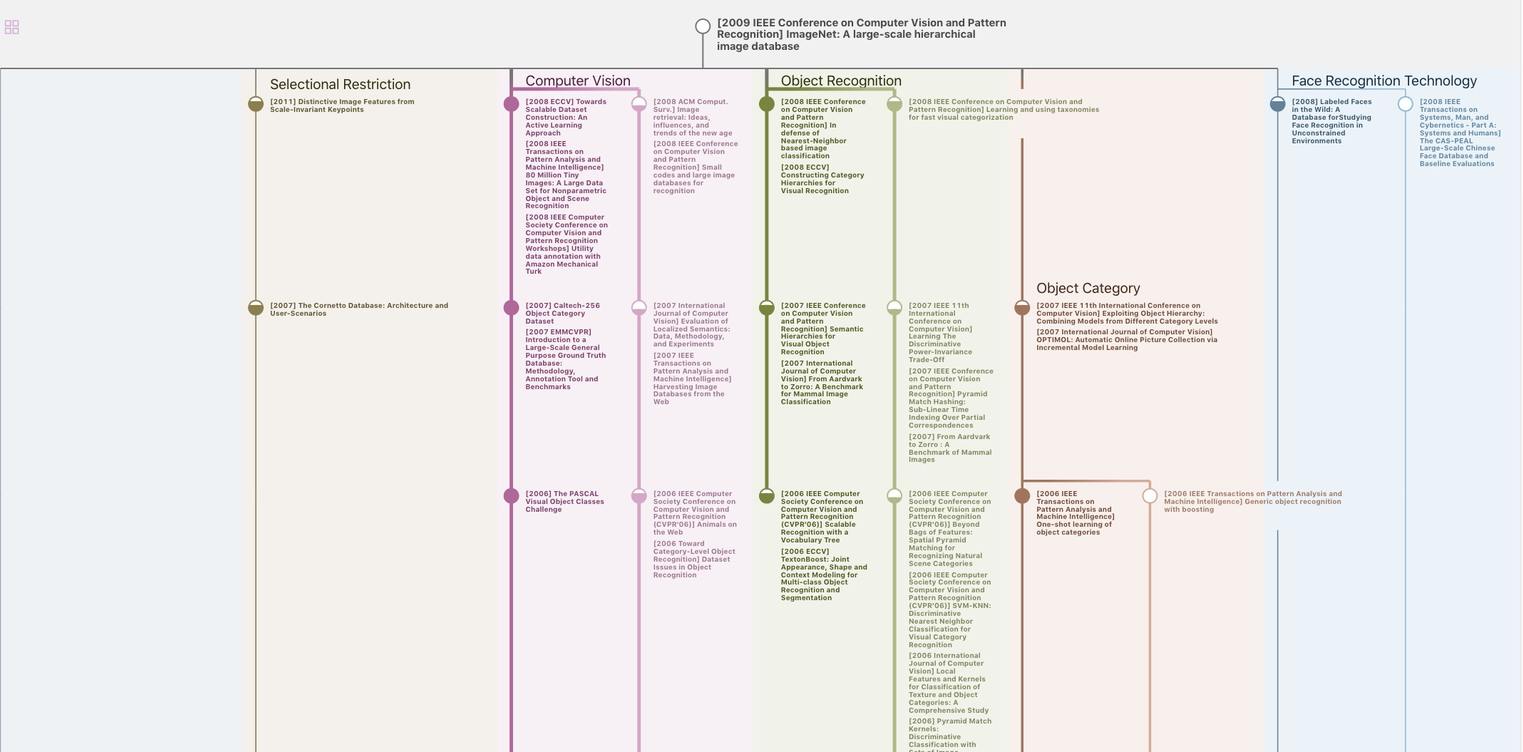
生成溯源树,研究论文发展脉络
Chat Paper
正在生成论文摘要