Bayesian Model Selection for Unsupervised Image Deconvolution with Structured Gaussian Priors
2021 IEEE Statistical Signal Processing Workshop (SSP)(2021)
摘要
This paper considers the comparison of models in the context of inverse problems. Most often, model comparison is addressed in a supervised manner, that can be time-consuming and partly arbitrary. Here we adopt an unsupervised Bayesian approach and quantitatively compare the models based on their posterior probabilities, directly calculated from available data without ground truth available. The probabilities depend on the evidences (marginal likelihoods) of the models and we resort to the Chib approach including a Gibbs sampler to compute them. We focus on the problem of image deconvolution, based on Gaussian models with unknown hyperparameters, in a circulant statement. We compare different impulse responses and covariance structures for image and noise.
更多查看译文
关键词
Bayesian model selection,unsupervised image deconvolution,structured Gaussian priors,inverse problems,unsupervised Bayesian approach,posterior probabilities,marginal likelihoods,Chib approach,Gaussian models,image covariance structures
AI 理解论文
溯源树
样例
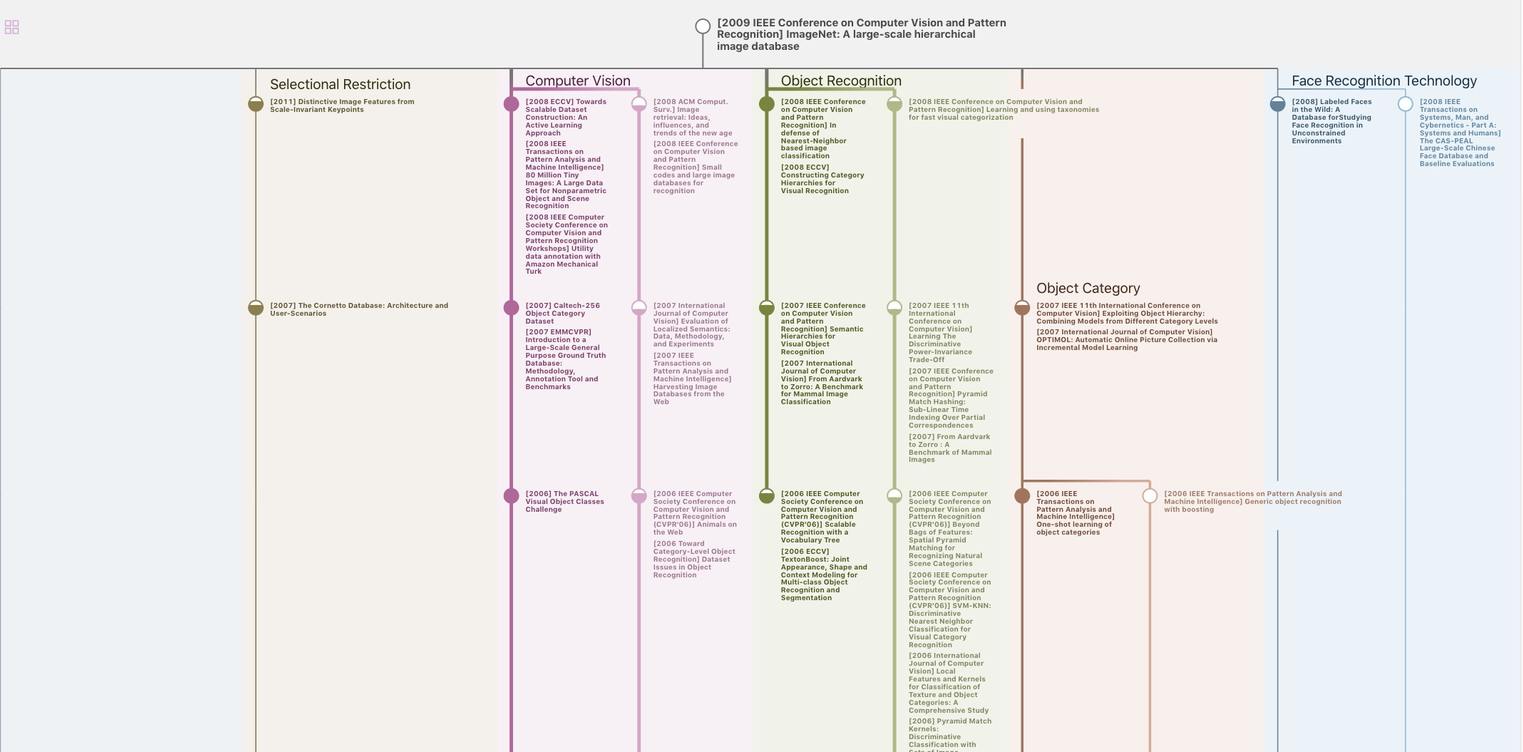
生成溯源树,研究论文发展脉络
Chat Paper
正在生成论文摘要